Paper by Jake Porway: “There is huge potential for AI and data science to play a productive role in advancing social impact. However, the field of “AI for good” and “data for good” is not only overshadowed by the public conversations about the risks rampant data misuse can pose to civil society, it is also a fractured and disconnected space without a single shared vision. One of the biggest issues preventing AI for Good from becoming an established field is a lack of clarity. “Data” and “AI” are treated like they’re well-defined terms, but they are stand-ins for a huge set of different – and sometimes competing – outcomes, outputs, and activities. The terms “AI for Good” and “Data for Good” is as unhelpful as saying “Wood for Good”. We would laugh at a term as vague as “Wood for Good”, which would lump together activities as different as building houses to burning wood in cook stoves to making paper, combining architecture with carpentry, forestry with fuel. However, we are content to say “AI for Good”, and its related phrases “we need to use our data better” or “we need to be data-driven”, when data is arguably even more general than something like wood.
One way to cut through this fog is to be far more clear about what a given AI-for-good initiative seeks to achieve, and by what means. To that end, we’ve created a taxonomy, a family tree of AI-and-Data-for-Good initiatives, that groups initiatives based on their goals and strategies. This taxonomy came about as a result of studying and classifying over 600 initiatives labeled as “AI for good” or “data for good” publicly (you can read more about the methodology here). At the highest level, the taxonomy identifies six major branches of AI and data for good activities, which will help field builders, funders, and nonprofits better understand the distinctions in this space. Within each branch, you’ll see sub-branches that further subdivide initiatives, which we hope will aid collaboration for the groups within those branches and help funders make more strategic funding decisions.
Below you’ll find a landscape map of a subset of initiatives in the AI and Data for Good space, categorized into those six main branches we observed. Before we dive into describing each branch, here are a few caveats on how to interpret this work:
- We are focused on initiatives that are enabling and advancing the field of Data for Good, not trying to catalog every data science project every nonprofit or company is doing (though that would be an interesting landscape to see as well). These initiatives are those focused on advancing the field of using data and AI for good.
- We chose to categorize initiatives, not organizations. It would be impossible to classify “Microsoft”, when their activities span funding through Microsoft Philanthropies, creating open satellite imagery through Microsoft AI for Earth, providing infrastructure through nonprofit licenses of Microsoft Azure, and so on. Therefore you may see organizations appear multiple times across the landscape.
- The current database of organizations is minuscule – it is nowhere near exhaustive. We have only mapped enough initiatives to show the results for feedback, but we encourage you to recommend other initiatives you know of but don’t see here with this form.
- While we are incredibly privileged to have advisors contributing to this project from countries like Brazil, Nigeria, and Nepal, the initiative still skew Western…(More)”.
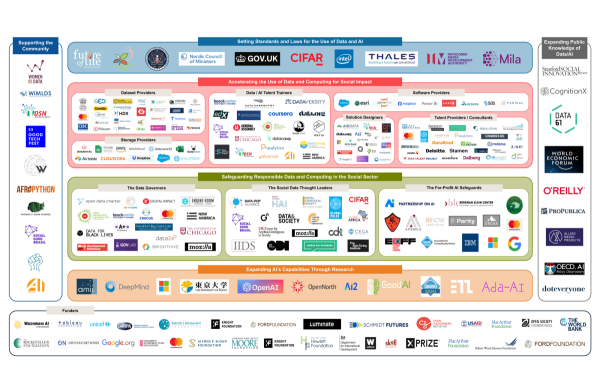