GAO Report: “Generative artificial intelligence (AI) could revolutionize entire industries. In the nearer term, it may dramatically increase productivity and transform daily tasks in many sectors. However, both its benefits and risks, including its environmental and human effects, are unknown or unclear.
Generative AI uses significant energy and water resources, but companies are generally not reporting details of these uses. Most estimates of environmental effects of generative AI technologies have focused on quantifying the energy consumed, and carbon emissions associated with generating that energy, required to train the generative AI model. Estimates of water consumption by generative AI are limited. Generative AI is expected to be a driving force for data center demand, but what portion of data center electricity consumption is related to generative AI is unclear. According to the International Energy Agency, U.S. data center electricity consumption was approximately 4 percent of U.S. electricity demand in 2022 and could be 6 percent of demand in 2026.
While generative AI may bring beneficial effects for people, GAO highlights five risks and challenges that could result in negative human effects on society, culture, and people from generative AI (see figure). For example, unsafe systems may produce outputs that compromise safety, such as inaccurate information, undesirable content, or the enabling of malicious behavior. However, definitive statements about these risks and challenges are difficult to make because generative AI is rapidly evolving, and private developers do not disclose some key technical information.
Selected generative artificial antelligence risks and challenges that could result in human effects
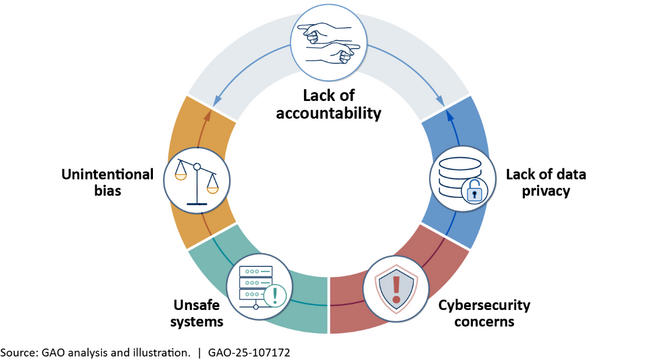
GAO identified policy options to consider that could enhance the benefits or address the challenges of environmental and human effects of generative AI. These policy options identify possible actions by policymakers, which include Congress, federal agencies, state and local governments, academic and research institutions, and industry. In addition, policymakers could choose to maintain the status quo, whereby they would not take additional action beyond current efforts. See below for details on the policy options…(More)”.