The Data Stewards Academy…A self-directed learning program from the Open Data Policy Lab (The GovLab): “Communities across the world face unprecedented challenges. Strained by climate change, crumbling infrastructure, growing economic inequality, and the continued costs of the COVID-19 pandemic, institutions need new ways of solving public problems and improving how they operate.
In recent years, data has been increasingly used to inform policies and interventions targeted at these issues. Yet, many of these data projects, data collaboratives, and open data initiatives remain scattered. As we enter into a new age of data use and re-use, a third wave of open data, it is more important than ever to be strategic and purposeful, to find new ways to connect the demand for data with its supply to meet institutional objectives in a socially responsible way.
This self-directed learning program, adapted from a selective executive education course, will help data stewards (and aspiring data stewards) develop a data re-use strategy to solve public problems. Noting the ways data resources can inform their day-to-day and strategic decision-making, the course provides learners with ways they can use data to improve how they operate and pursue goals in the public’s interests. By working differently—using agile methods and data analytics—public, private, and civil sector leaders can promote data re-use and reduce data access inequities in ways that advance their institution’s goals.
In this self-directed learning program, we will teach participants how to develop a 21st century data strategy. Participants will learn:
- Why It Matters: A discussion of the three waves of open data and how data re-use has proven to be transformative;
- The Current State of Play: Current practice around data re-use, including deficits of current approaches and the need to shift from ad hoc engagements to more systematic, sustainable, and responsible models;
- Defining Demand: Methodologies for how organizations can formulate questions that data can answer; and make data collaboratives more purposeful;
- Mapping Supply: Methods for organizations to discover and assess the open and private data needed to answer the questions at hand that potentially may be available to them;
- Matching Supply with Demand: Operational models for connecting and meeting the needs of supply- and demand-side actors in a sustainable way;
- Identifying Risks: Overview of the risks that can emerge in the course of data re-use;
- Mitigating Risks and Other Considerations: Technical, legal and contractual issues that can be leveraged or may arise in the course of data collaboration and other data work; and
- Institutionalizing Data Re-use: Suggestions for how organizations can incorporate data re-use into their organizational structure and foster future collaboration and data stewardship.
The Data Stewardship Executive Education Course was designed and implemented by program leads Stefaan Verhulst, co-founder and chief research development officer at the GovLab, and Andrew Young, The GovLab’s knowledge director, in close collaboration with a global network of expert faculty and advisors. It aims to….(More)”.
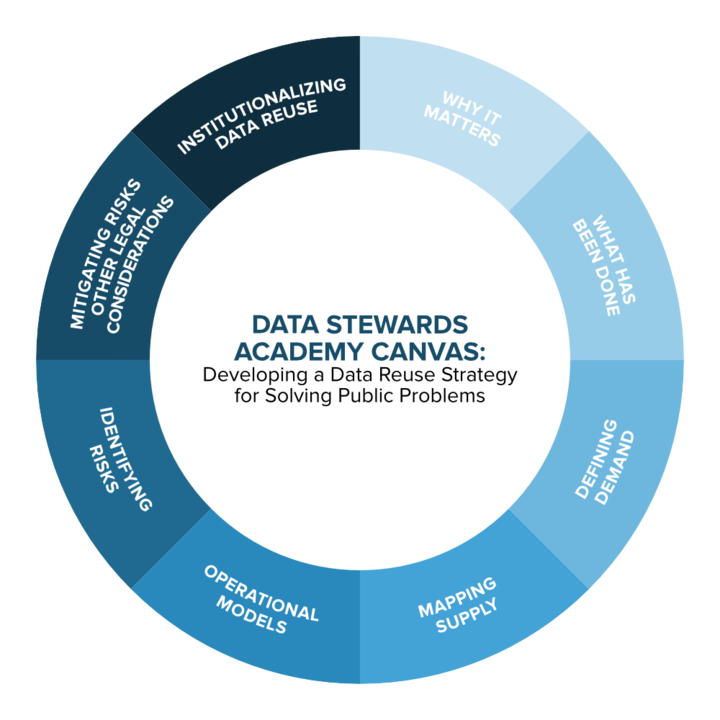