Turing Institute: “…Policy Priority Inference builds on a behavioural computational model, taking into account the learning process of public officials, coordination problems, incomplete information, and imperfect governmental monitoring mechanisms. The approach is a unique mix of economic theory, behavioural economics, network science and agent-based modelling. The data that feeds the model for a specific country (or a sub-national unit, such as a state) includes measures of the country’s DIs and how they have moved over the years, specified government policy goals in relation to DIs, the quality of government monitoring of expenditure, and the quality of the country’s rule of law.
From these data alone – and, crucially, with no specific information on government expenditure, which is rarely made available – the model can infer the transformative resources a country has historically allocated to transform its SDGs, and assess the importance of SDG interlinkages between DIs. Importantly, it can also reveal where previously hidden inefficiencies lie.
How does it work? The researchers modelled the socioeconomic mechanisms of the policy-making process using agent-computing simulation. They created a simulator featuring an agent called “Government”, which makes decisions about how to allocate public expenditure, and agents called “Bureaucrats”, each of which is essentially a policy-maker linked to a single DI. If a Bureaucrat is allocated some resource, they will use a portion of it to improve their DI, with the rest lost to some degree of inefficiency (in reality, inefficiencies range from simple corruption to poor quality policies and inefficient government departments).
How much resource a Bureaucrat puts towards moving their DI depends on that agent’s experience: if becoming inefficient pays off, they’ll keep doing it. During the process, Government monitors the Bureaucrats, occasionally punishing inefficient ones, who may then improve their behaviour. In the model, a Bureaucrat’s chances of getting caught is linked to the quality of a government’s real-world monitoring of expenditure, and the extent to which they are punished is reflected in the strength of that country’s rule of law.
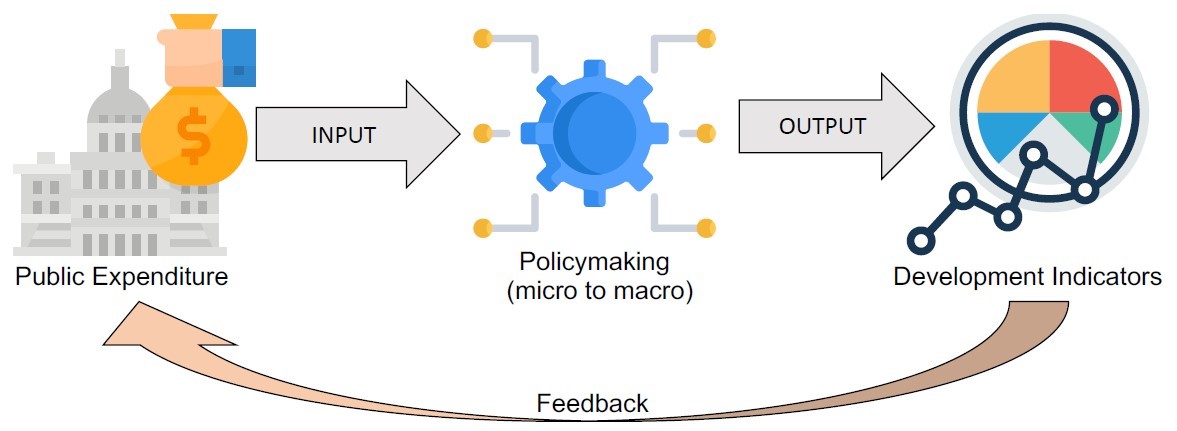
When the historical movements of a country’s DIs are reproduced through the internal workings of the model, the researchers have a powerful proxy for the real-world relationships between government activity, the movement of DIs, and the effects of the interlinkages between DIs, all of which are unique to that country. “Once we can match outcomes, we can discern something that’s going on in reality. But the fact that the method is matching the dynamics of real-world development indicators is just one of multiple ways that we validate our results,” Guerrero notes. This proxy can then be used to project which policy areas should be prioritised in future to best achieve the government’s specified development goals, including predictions of likely timescales.
What’s more, in combination with techniques from evolutionary computation, the model can identify DIs that are linked to large positive spillover effects. These DIs are dubbed “accelerators”. Targeting government resources at such development accelerators fosters not only more rapid results, but also more generalised development…(More)”.