Paper by Stephanie Cunningham-Reimann et al: “Indigenous data sovereignty is of global concern. The power of data through its multitude of uses can cause harm to Indigenous Peoples, communities, organisations and Nations in Canada and globally. Indigenous research principles play a vital role in guiding researchers, scholars and policy makers in their careers and roles. We define data, data sovereignty principles, ways of practicing Indigenous research principles, and recommendations for applying and actioning Indigenous data sovereignty through culturally safe self-reflection, interpersonal and reciprocal relationships built upon respect, reciprocity, relevance, responsibility and accountability. Research should be co-developed, co-led, and co-disseminated in partnership with Indigenous Peoples, communities, organisations and/or nations to build capacity, support self-determination, and reduce harms produced through the analysis and dissemination of research findings. OCAP® (Ownership, Control, Access & Possession), OCAS (Ownership, Control, Access & Stewardship), Inuit Qaujimajatuqangit principles in conjunction the 4Rs (respect, relevance, reciprocity & responsibility) and cultural competency including self-examination of the 3Ps (power, privilege, and positionality) of researchers, scholars and policy makers can be challenging, but will amplify the voices and understandings of Indigenous research by implementing Indigenous data sovereignty in Canada…(More)”
AI Commons: nourishing alternatives to Big Tech monoculture
Report by Joana Varon, Sasha Costanza-Chock, Mariana Tamari, Berhan Taye, and Vanessa Koetz: “‘Artificial Intelligence’ (AI) has become a buzzword all around the globe, with tech companies, research institutions, and governments all vying to define and shape its future. How can we escape the current context of AI development where certain power forces are pushing for models that, ultimately, automate inequalities and threaten socio-enviromental diversities? What if we could redefine AI? What if we could shift its production from a capitalist model to a more disruptive, inclusive, and decentralized one? Can we imagine and foster an AI Commons ecosystem that challenges the current dominant neoliberal logic of an AI arms race? An ecosystem encompassing researchers, developers, and activists who are thinking about AI from decolonial, transfeminist, antiracist, indigenous, decentralized, post-capitalist and/or socio-environmental justice perspectives?
This fieldscan research, commissioned by One Project and conducted by Coding Rights, aims to understand the (possibly) emerging “AI Common” ecosystem. Focused on key entities (organizations, cooperatives and collectives, networks, companies, projects, and others) from Africa, the Americas, and Europe advancing alternative possible AI futures, the authors identify 234 entities that are advancing the AI Commons ecosystem. The report finds powerful communities of practice, groups, and organizations producing nuanced criticism of the Big Tech-driven AI development ecosystem and, most importantly, imagining, developing, and, at times, deploying an alternative AI technology that’s informed and guided by the principles of decoloniality, feminism, antiracist, and post-capitalist AI systems…(More)”.
Developing a theory of robust democracy
Paper by Eva Sørensen and Mark E. Warren: “While many democratic theorists recognise the necessity of reforming liberal democracies to keep pace with social change, they rarely consider what enables such reform. In this conceptual article, we suggest that liberal democracies are politically robust when they are able to continuously adapt and innovate how they operate when doing so is necessary to continue to serve key democratic functions. These functions include securing the empowered inclusion of those affected, collective agenda setting and will formation, and the making of joint decisions. Three current challenges highlight the urgency of adapting and innovating liberal democracies to become more politically robust: an increasingly assertive political culture, the digitalisation of political communication and increasing global interdependencies. A democratic theory of political robustness emphasises the need to strengthen the capacity of liberal democracies to adapt and innovate in response to changes, just as it helps to frame the necessary adaptations and innovations in times such as the present…(More)”
The Impact of Artificial Intelligence on Societies
Book edited by Christian Montag and Raian Ali: “This book presents a recent framework proposed to understand how attitudes towards artificial intelligence are formed. It describes how the interplay between different variables, such as the modality of AI interaction, the user personality and culture, the type of AI applications (e.g. in the realm of education, medicine, transportation, among others), and the transparency and explainability of AI systems contributes to understand how user’s acceptance or a negative attitude towards AI develops. Gathering chapters from leading researchers with different backgrounds, this book offers a timely snapshot on factors that will be influencing the impact of artificial intelligence on societies…(More)”.
The Nature and Dynamics of Collaboration
Book edited by Paul F. M. J. Verschure: “Human existence depends critically on how well diverse social, cultural and political groups can collaborate. Yet the phenomenon of collaboration itself is ill-defined and badly understood, and there is no straightforward formula for its successful realization. In The Nature and Dynamics of Collaboration, edited by Paul F. M. J. Verschure, experts from wide-ranging disciplines examine how human collaboration arises, breaks down, and potentially recovers. They explore the different contexts, boundary conditions, and drivers of collaboration to expand understanding of the underlying dynamic, multiscale processes in an effort to increase chances for ethical, sustainable, and productive collaboration in the future. This volume is accompanied by twenty-four podcasts, which provide insights from real-world examples…(More)”.
Data Stewardship as Environmental Stewardship
Article by Stefaan Verhulst and Sara Marcucci: “Why responsible data stewardship could help address today’s pressing environmental challenges resulting from artificial intelligence and other data-related technologies…
Even as the world grows increasingly reliant on data and artificial intelligence, concern over the environmental impact of data-related activities is increasing. Solutions remain elusive. The rise of generative AI, which rests on a foundation of massive data sets and computational power, risks exacerbating the problem.
In the below, we propose that responsible data stewardship offers a potential pathway to reducing the environmental footprint of data activities. By promoting practices such as data reuse, minimizing digital waste, and optimizing storage efficiency, data stewardship can help mitigate environmental harm. Additionally, data stewardship supports broader environmental objectives by facilitating better decision-making through transparent, accessible, and shared data. In the below, we suggest that advancing data stewardship as a cornerstone of environmental responsibility could provide a compelling approach to addressing the dual challenges of advancing digital technologies while safeguarding the environment…(More)”
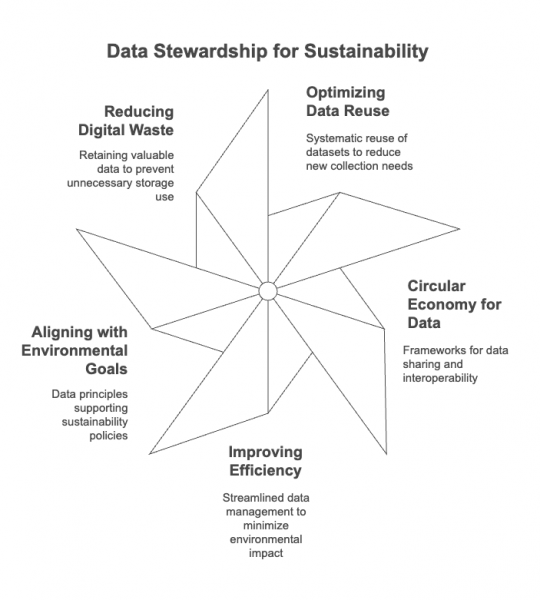
Data Governance Meets the EU AI Act
Article by Axel Schwanke: “..The EU AI Act emphasizes sustainable AI through robust data governance, promoting principles like data minimization, purpose limitation, and data quality to ensure responsible data collection and processing. It mandates measures such as data protection impact assessments and retention policies. Article 10 underscores the importance of effective data management in fostering ethical and sustainable AI development…This article states that high-risk AI systems must be developed using high-quality data sets for training, validation, and testing. These data sets should be managed properly, considering factors like data collection processes, data preparation, potential biases, and data gaps. The data sets should be relevant, representative, error-free, and complete as much as possible. They should also consider the specific context in which the AI system will be used. In some cases, providers may process special categories of personal data to detect and correct biases, but they must follow strict conditions to protect individuals’ rights and freedoms…
However, achieving compliance presents several significant challenges:
- Ensuring Dataset Quality and Relevance: Organizations must establish robust data and AI platforms to prepare and manage datasets that are error-free, representative, and contextually relevant for their intended use cases. This requires rigorous data preparation and validation processes.
- Bias and Contextual Sensitivity: Continuous monitoring for biases in data is critical. Organizations must implement corrective actions to address gaps while ensuring compliance with privacy regulations, especially when processing personal data to detect and reduce bias.
- End-to-End Traceability: A comprehensive data governance framework is essential to track and document data flow from its origin to its final use in AI models. This ensures transparency, accountability, and compliance with regulatory requirements.
- Evolving Data Requirements: Dynamic applications and changing schemas, particularly in industries like real estate, necessitate ongoing updates to data preparation processes to maintain relevance and accuracy.
- Secure Data Processing: Compliance demands strict adherence to secure processing practices for personal data, ensuring privacy and security while enabling bias detection and mitigation.
Example: Real Estate Data
Immowelt’s real estate price map, awarded as the top performer in a 2022 test of real estate price maps, exemplifies the challenges of achieving high-quality datasets. The prepared data powers numerous services and applications, including data analysis, price predictions, personalization, recommendations, and market research…(More)”
Why Digital Public Goods, including AI, Should Depend on Open Data
Article by Cable Green: “Acknowledging that some data should not be shared (for moral, ethical and/or privacy reasons) and some cannot be shared (for legal or other reasons), Creative Commons (CC) thinks there is value in incentivizing the creation, sharing, and use of open data to advance knowledge production. As open communities continue to imagine, design, and build digital public goods and public infrastructure services for education, science, and culture, these goods and services – whenever possible and appropriate – should produce, share, and/or build upon open data.
Open Data and Digital Public Goods (DPGs)
CC is a member of the Digital Public Goods Alliance (DPGA) and CC’s legal tools have been recognized as digital public goods (DPGs). DPGs are “open-source software, open standards, open data, open AI systems, and open content collections that adhere to privacy and other applicable best practices, do no harm, and are of high relevance for attainment of the United Nations 2030 Sustainable Development Goals (SDGs).” If we want to solve the world’s greatest challenges, governments and other funders will need to invest in, develop, openly license, share, and use DPGs.
Open data is important to DPGs because data is a key driver of economic vitality with demonstrated potential to serve the public good. In the public sector, data informs policy making and public services delivery by helping to channel scarce resources to those most in need; providing the means to hold governments accountable and foster social innovation. In short, data has the potential to improve people’s lives. When data is closed or otherwise unavailable, the public does not accrue these benefits.CC was recently part of a DPGA sub-committee working to preserve the integrity of open data as part of the DPG Standard. This important update to the DPG Standard was introduced to ensure only open datasets and content collections with open licenses are eligible for recognition as DPGs. This new requirement means open data sets and content collections must meet the following criteria to be recognised as a digital public good.
- Comprehensive Open Licensing:
- The entire data set/content collection must be under an acceptable open licence. Mixed-licensed collections will no longer be accepted.
- Accessible and Discoverable:
- All data sets and content collection DPGs must be openly licensed and easily accessible from a distinct, single location, such as a unique URL.
- Permitted Access Restrictions:
- Certain access restrictions – such as logins, registrations, API keys, and throttling – are permitted as long as they do not discriminate against users or restrict usage based on geography or any other factors…(More)”.
Mindmasters: The Data-Driven Science of Predicting and Changing Human Behavior
Book by Sandra Matz: “There are more pieces of digital data than there are stars in the universe. This data helps us monitor our planet, decipher our genetic code, and take a deep dive into our psychology.
As algorithms become increasingly adept at accessing the human mind, they also become more and more powerful at controlling it, enticing us to buy a certain product or vote for a certain political candidate. Some of us say this technological trend is no big deal. Others consider it one of the greatest threats to humanity. But what if the truth is more nuanced and mind-bending than that?
In Mindmasters, Columbia Business School professor Sandra Matz reveals in fascinating detail how big data offers insights into the most intimate aspects of our psyches and how these insights empower an external influence over the choices we make. This can be creepy, manipulative, and downright harmful, with scandals like that of British consulting firm Cambridge Analytica being merely the tip of the iceberg. Yet big data also holds enormous potential to help us live healthier, happier lives—for example, by improving our mental health, encouraging better financial decisions, or enabling us to break out of our echo chambers..(More)”.
Problems of participatory processes in policymaking: a service design approach
Paper by Susana Díez-Calvo, Iván Lidón, Rubén Rebollar, Ignacio Gil-Pérez: “This study aims to identify and map the problems of participatory processes in policymaking through a Service Design approach….Fifteen problems of participatory processes in policymaking were identified, and some differences were observed in the perception of these problems between the stakeholders responsible for designing and implementing the participatory processes (backstage stakeholders) and those who are called upon to participate (frontstage stakeholders). The problems were found to occur at different stages of the service and to affect different stakeholders. A number of design actions were proposed to help mitigate these problems from a human-centred approach. These included process improvements, digital opportunities, new technologies and staff training, among others…(More)”.