Paper by Michael Schudson: “Transparency” has become a widely recognized, even taken for granted, value in contemporary democracies, but this has been true only since the 1970s. For all of the obvious virtues of transparency for democracy, they have not always been recognized or they have been recognized, as in the U.S. Freedom of Information Act of 1966, with significant qualifications. This essay catalogs important shortcomings of transparency for democracy, as when it clashes with national security, personal privacy, and the importance of maintaining the capacity of government officials to talk frankly with one another without fear that half-formulated ideas, thoughts, and proposals will become public. And when government information becomes public, that does not make it equally available to all—publicity is not in itself democratic, as public information (as in open legislative committee hearings) is more readily accessed by empowered groups with lobbyists able to attend and monitor the provision of the information. Transparency is an element in democratic government, but it is by no means a perfect emblem of democracy….(More)”.
Project Patient Voice
Press Release: “The U.S. Food and Drug Administration today launched Project Patient Voice, an initiative of the FDA’s Oncology Center of Excellence (OCE). Through a new website, Project Patient Voice creates a consistent source of publicly available information describing patient-reported symptoms from cancer trials for marketed treatments. While this patient-reported data has historically been analyzed by the FDA during the drug approval process, it is rarely included in product labeling and, therefore, is largely inaccessible to the public.
“Project Patient Voice has been initiated by the Oncology Center of Excellence to give patients and health care professionals unique information on symptomatic side effects to better inform their treatment choices,” said FDA Principal Deputy Commissioner Amy Abernethy, M.D., Ph.D. “The Project Patient Voice pilot is a significant step in advancing a patient-centered approach to oncology drug development. Where patient-reported symptom information is collected rigorously, this information should be readily available to patients.”
Patient-reported outcome (PRO) data is collected using questionnaires that patients complete during clinical trials. These questionnaires are designed to capture important information about disease- or treatment-related symptoms. This includes how severe or how often a symptom or side effect occurs.
Patient-reported data can provide additional, complementary information for health care professionals to discuss with patients, specifically when discussing the potential side effects of a particular cancer treatment. In contrast to the clinician-reported safety data in product labeling, the data in Project Patient Voice is obtained directly from patients and can show symptoms before treatment starts and at multiple time points while receiving cancer treatment.
The Project Patient Voice website will include a list of cancer clinical trials that have available patient-reported symptom data. Each trial will include a table of the patient-reported symptoms collected. Each patient-reported symptom can be selected to display a series of bar and pie charts describing the patient-reported symptom at baseline (before treatment starts) and over the first 6 months of treatment. This information provides insights into side effects not currently available in standard FDA safety tables, including existing symptoms before the start of treatment, symptoms over time, and the subset of patients who did not have a particular symptom prior to starting treatment….(More)”.
Measuring Movement and Social Contact with Smartphone Data: A Real-Time Application to Covid-19
Paper by Victor Couture et al: “Tracking human activity in real time and at fine spatial scale is particularly valuable during episodes such as the COVID-19 pandemic. In this paper, we discuss the suitability of smartphone data for quantifying movement and social contact. We show that these data cover broad sections of the US population and exhibit movement patterns similar to conventional survey data. We develop and make publicly available a location exposure index that summarizes county-to-county movements and a device exposure index that quantifies social contact within venues. We use these indices to document how pandemic-induced reductions in activity vary across people and places….(More)”.
The Atlas of Surveillance
Electronic Frontier Foundation: “Law enforcement surveillance isn’t always secret. These technologies can be discovered in news articles and government meeting agendas, in company press releases and social media posts. It just hasn’t been aggregated before.
That’s the starting point for the Atlas of Surveillance, a collaborative effort between the Electronic Frontier Foundation and the University of Nevada, Reno Reynolds School of Journalism. Through a combination of crowdsourcing and data journalism, we are creating the largest-ever repository of information on which law enforcement agencies are using what surveillance technologies. The aim is to generate a resource for journalists, academics, and, most importantly, members of the public to check what’s been purchased locally and how technologies are spreading across the country.
We specifically focused on the most pervasive technologies, including drones, body-worn cameras, face recognition, cell-site simulators, automated license plate readers, predictive policing, camera registries, and gunshot detection. Although we have amassed more than 5,000 datapoints in 3,000 jurisdictions, our research only reveals the tip of the iceberg and underlines the need for journalists and members of the public to continue demanding transparency from criminal justice agencies….(More)”.
Tackling the misinformation epidemic with “In Event of Moon Disaster”
MIT Open Learning: “Can you recognize a digitally manipulated video when you see one? It’s harder than most people realize. As the technology to produce realistic “deepfakes” becomes more easily available, distinguishing fact from fiction will only get more challenging. A new digital storytelling project from MIT’s Center for Advanced Virtuality aims to educate the public about the world of deepfakes with “In Event of Moon Disaster.”
This provocative website showcases a “complete” deepfake (manipulated audio and video) of U.S. President Richard M. Nixon delivering the real contingency speech written in 1969 for a scenario in which the Apollo 11 crew were unable to return from the moon. The team worked with a voice actor and a company called Respeecher to produce the synthetic speech using deep learning techniques. They also worked with the company Canny AI to use video dialogue replacement techniques to study and replicate the movement of Nixon’s mouth and lips. Through these sophisticated AI and machine learning technologies, the seven-minute film shows how thoroughly convincing deepfakes can be….
Alongside the film, moondisaster.org features an array of interactive and educational resources on deepfakes. Led by Panetta and Halsey Burgund, a fellow at MIT Open Documentary Lab, an interdisciplinary team of artists, journalists, filmmakers, designers, and computer scientists has created a robust, interactive resource site where educators and media consumers can deepen their understanding of deepfakes: how they are made and how they work; their potential use and misuse; what is being done to combat deepfakes; and teaching and learning resources….(More)”.
The Coronavirus and Innovation
Essay by Scott E. Page: “The total impact of the coronavirus pandemic—the loss of life and the economic, social, and psychological costs arising from both the pandemic itself and the policies implemented to prevent its spread—defy any characterization. Though the pandemic continues to unsettle, disrupt, and challenge communities, we might take a moment to appreciate and applaud the diversity, breadth, and scope of our responses—from individual actions to national policies—and even more important, to reflect on how they will produce a post–Covid-19 world far better than the world that preceded it.
In this brief essay, I describe how our adaptive responses to the coronavirus will lead to beneficial policy innovations. I do so from the perspective of a many-model thinker. By that I mean that I will use several formal models to theoretically elucidate the potential pathways to creating a better world. I offer this with the intent that it instills optimism that our current efforts to confront this tragic and difficult challenge will do more than combat the virus now and teach us how to combat future viruses. They will, in the long run, result in an enormous number of innovations in policy, business practices, and our daily lives….(More)”.
Why Hundreds of Mathematicians Are Boycotting Predictive Policing
Courtney Linder at Popular Mechanics: “Several prominent academic mathematicians want to sever ties with police departments across the U.S., according to a letter submitted to Notices of the American Mathematical Society on June 15. The letter arrived weeks after widespread protests against police brutality, and has inspired over 1,500 other researchers to join the boycott.
These mathematicians are urging fellow researchers to stop all work related to predictive policing software, which broadly includes any data analytics tools that use historical data to help forecast future crime, potential offenders, and victims. The technology is supposed to use probability to help police departments tailor their neighborhood coverage so it puts officers in the right place at the right time….
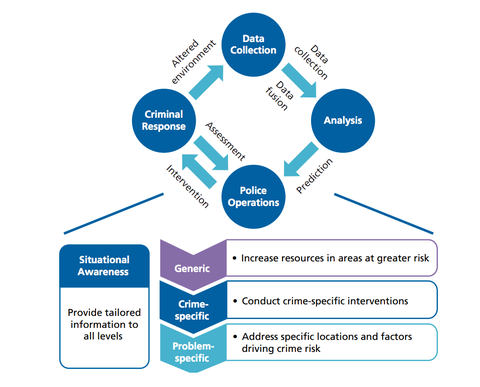
RAND
According to a 2013 research briefing from the RAND Corporation, a nonprofit think tank in Santa Monica, California, predictive policing is made up of a four-part cycle (shown above). In the first two steps, researchers collect and analyze data on crimes, incidents, and offenders to come up with predictions. From there, police intervene based on the predictions, usually taking the form of an increase in resources at certain sites at certain times. The fourth step is, ideally, reducing crime.
“Law enforcement agencies should assess the immediate effects of the intervention to ensure that there are no immediately visible problems,” the authors note. “Agencies should also track longer-term changes by examining collected data, performing additional analysis, and modifying operations as needed.”
In many cases, predictive policing software was meant to be a tool to augment police departments that are facing budget crises with less officers to cover a region. If cops can target certain geographical areas at certain times, then they can get ahead of the 911 calls and maybe even reduce the rate of crime.
But in practice, the accuracy of the technology has been contested—and it’s even been called racist….(More)”.
Differential Privacy for Privacy-Preserving Data Analysis
Introduction to a Special Blog Series by NIST: “…How can we use data to learn about a population, without learning about specific individuals within the population? Consider these two questions:
- “How many people live in Vermont?”
- “How many people named Joe Near live in Vermont?”
The first reveals a property of the whole population, while the second reveals information about one person. We need to be able to learn about trends in the population while preventing the ability to learn anything new about a particular individual. This is the goal of many statistical analyses of data, such as the statistics published by the U.S. Census Bureau, and machine learning more broadly. In each of these settings, models are intended to reveal trends in populations, not reflect information about any single individual.
But how can we answer the first question “How many people live in Vermont?” — which we’ll refer to as a query — while preventing the second question from being answered “How many people name Joe Near live in Vermont?” The most widely used solution is called de-identification (or anonymization), which removes identifying information from the dataset. (We’ll generally assume a dataset contains information collected from many individuals.) Another option is to allow only aggregate queries, such as an average over the data. Unfortunately, we now understand that neither approach actually provides strong privacy protection. De-identified datasets are subject to database-linkage attacks. Aggregation only protects privacy if the groups being aggregated are sufficiently large, and even then, privacy attacks are still possible [1, 2, 3, 4].
Differential Privacy
Differential privacy [5, 6] is a mathematical definition of what it means to have privacy. It is not a specific process like de-identification, but a property that a process can have. For example, it is possible to prove that a specific algorithm “satisfies” differential privacy.
Informally, differential privacy guarantees the following for each individual who contributes data for analysis: the output of a differentially private analysis will be roughly the same, whether or not you contribute your data. A differentially private analysis is often called a mechanism, and we denote it ℳ.
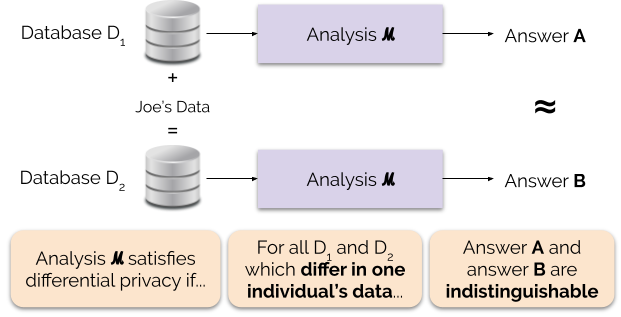
Figure 1 illustrates this principle. Answer “A” is computed without Joe’s data, while answer “B” is computed with Joe’s data. Differential privacy says that the two answers should be indistinguishable. This implies that whoever sees the output won’t be able to tell whether or not Joe’s data was used, or what Joe’s data contained.
We control the strength of the privacy guarantee by tuning the privacy parameter ε, also called a privacy loss or privacy budget. The lower the value of the ε parameter, the more indistinguishable the results, and therefore the more each individual’s data is protected.

We can often answer a query with differential privacy by adding some random noise to the query’s answer. The challenge lies in determining where to add the noise and how much to add. One of the most commonly used mechanisms for adding noise is the Laplace mechanism [5, 7].
Queries with higher sensitivity require adding more noise in order to satisfy a particular `epsilon` quantity of differential privacy, and this extra noise has the potential to make results less useful. We will describe sensitivity and this tradeoff between privacy and usefulness in more detail in future blog posts….(More)”.
What Ever Happened to Digital Contact Tracing?
Chas Kissick, Elliot Setzer, and Jacob Schulz at Lawfare: “In May of this year, Prime Minister Boris Johnson pledged the United Kingdom would develop a “world beating” track and trace system by June 1 to stop the spread of the novel coronavirus. But on June 18, the government quietly abandoned its coronavirus contact-tracing app, a key piece of the “world beating” strategy, and instead promised to switch to a model designed by Apple and Google. The delayed app will not be ready until winter, and the U.K.’s Junior Health Minister told reporters that “it isn’t a priority for us at the moment.” When Johnson came under fire in Parliament for the abrupt U-turn, he replied: “I wonder whether the right honorable and learned Gentleman can name a single country in the world that has a functional contact tracing app—there isn’t one.”
Johnson’s rebuttal is perhaps a bit reductive, but he’s not that far off.
You probably remember the idea of contact-tracing apps: the technological intervention that seemed to have the potential to save lives while enabling a hamstrung economy to safely inch back open; it was a fixation of many public health and privacy advocates; it was the thing that was going to help us get out of this mess if we could manage the risks.
Yet nearly three months after Google and Apple announced with great fanfare their partnership to build a contact-tracing API, contact-tracing apps have made an unceremonious exit from the front pages of American newspapers. Countries, states and localities continue to try to develop effective digital tracing strategies. But as Jonathan Zittrain puts it, the “bigger picture momentum appears to have waned.”
What’s behind contact-tracing apps’ departure from the spotlight? For one, there’s the onset of a larger pandemic apathy in the U.S; many politicians and Americans seem to have thrown up their hands or put all their hopes in the speedy development of a vaccine. Yet, the apps haven’t even made much of a splash in countries that havetaken the pandemic more seriously. Anxieties about privacy persist. But technical shortcomings in the apps deserve the lion’s share of the blame. Countries have struggled to get bespoke apps developed by government technicians to work on Apple phones. The functionality of some Bluetooth-enabled models vary widely depending on small changes in phone positioning. And most countries have only convinced a small fraction of their populace to use national tracing apps.
Maybe it’s still possible that contact-tracing apps will make a miraculous comeback and approach the level of efficacy observers once anticipated.
But even if technical issues implausibly subside, the apps are operating in a world of unknowns.
Most centrally, researchers still have no real idea what level of adoption is required for the apps to actually serve their function. Some estimates suggest that 80 percent of current smartphone owners in a given area would need to use an app and follow its recommendations for digital contact tracing to be effective. But other researchers have noted that the apps could slow the rate of infections even if little more than 10 percent of a population used a tracing app. It will be an uphill battle even to hit the 10 percent mark in America, though. Survey data show that fewer than three in 10 Americans intend to use contact-tracing apps if they become available…(More).
Citizen initiatives facing COVID-19: Due to spontaneous generation or the product of social capital in Mexico City?
UNDP: “Since the COVID-19 pandemic arrived in Mexico, multiple citizen responses[1] have emerged to tackle its impacts: digital aid platforms such as Frena la Curva (Stop the curve) and México Covid19; groups of makers that design medical and protective equipment; Zapotec indigenous women that teach how to make hand sanitizer at home; public buses that turn into mobile markets; and much more.
There are initiatives that aid 10, 20, 3,000 or more people; initiatives that operate inside a housing unit, a municipality or across the City. Some responses have come from civil society organizations; others from collectives of practitioners or from groups of friends and family; there are even those made out of groups of strangers that the pandemic turned into partners working for the same goal….
Within the plurality of initiatives that have emerged, sometimes, seemingly in a spontaneous way, there is a common denominator: people are reacting in a collaboratively way to the crisis to solve the needs that the pandemic is leaving behind.
Different research studies connect social cohesion and social capital with the response’s capacity of a community in situations of crisis and natural disasters; and with its subsequent recovery. These concepts —derived from sociology— include aspects such as the level of union; relationships and networks; and interaction between people in a community.
This can be seen when, for example, a group of people in a neighborhood gets together to buy groceries for neighbors who have lost their incomes. Also, when a collective of professionals react to the shortages of protective equipment for health workers by creating low-cost prototypes, or when a civil organization collaborates with local authorities to bring water to households that lack access to clean water.
It would seem that a high rate of social capital and social cohesion might ease the rise of the citizen initiatives that aim to tackle the challenges that ensue from the pandemic. These do not come out of nowhere….(More)”.
