Article by Clare Watson: “Most biomedical and health researchers who declare their willingness to share the data behind journal articles do not respond to access requests or hand over the data when asked, a study reports1.
Livia Puljak, who studies evidence-based medicine at the Catholic University of Croatia in Zagreb, and her colleagues analysed 3,556 biomedical and health science articles published in a month by 282 BMC journals. (BMC is part of Springer Nature, the publisher of Nature; Nature’s news team is editorially independent of its publisher.)
The team identified 381 articles with links to data stored in online repositories and another 1,792 papers for which the authors indicated in statements that their data sets would be available on reasonable request. The remaining studies stated that their data were in the published manuscript and its supplements, or generated no data, so sharing did not apply.
But of the 1,792 manuscripts for which the authors stated they were willing to share their data, more than 90% of corresponding authors either declined or did not respond to requests for raw data (see ‘Data-sharing behaviour’). Only 14%, or 254, of the contacted authors responded to e-mail requests for data, and a mere 6.7%, or 120 authors, actually handed over the data in a usable format. The study was published in the Journal of Clinical Epidemiology on 29 May.
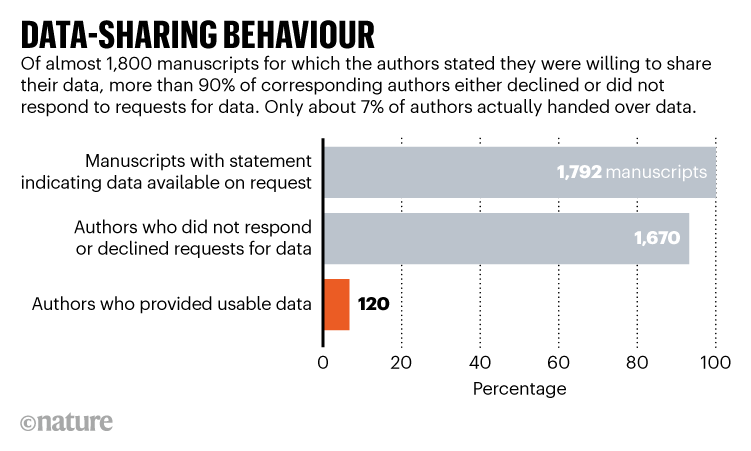
Puljak was “flabbergasted” that so few researchers actually shared their data. “There is a gap between what people say and what people do,” she says. “Only when we ask for the data can we see their attitude towards data sharing.”
“It’s quite dismaying that [researchers] are not coming forward with the data,” says Rebecca Li, who is executive director of non-profit global data-sharing platform Vivli and is based in Cambridge, Massachusetts…(More)”.