Book by Salman Khan: “…explores how artificial intelligence and GPT technology will transform learning, and offers a road map for teachers, parents, and students to navigate this exciting (and sometimes intimidating) new world.
A pioneer in the field of education technology, Khan examines the ins and outs of these cutting-edge tools and how they will revolutionize the way we learn and teach. For parents concerned about their children’s success, Khan illustrates how AI can personalize learning by adapting to each student’s individual pace and style, identifying strengths and areas for improvement, and offering tailored support and feedback to complement traditional classroom instruction. Khan emphasizes that embracing AI in education is not about replacing human interaction but enhancing it with customized and accessible learning tools that encourage creative problem-solving skills and prepare students for an increasingly digital world.
But Brave New Words is not just about technology—it’s about what this technology means for our society, and the practical implications for administrators, guidance counselors, and hiring managers who can harness the power of AI in education and the workplace. Khan also delves into the ethical and social implications of AI and large language models, offering thoughtful insights into how we can use these tools to build a more accessible education system for students around the world…(More)”.
US Senate AI Working Group Releases Policy Roadmap
Article by Gabby Miller: “On Wednesday, May 15, 2024, a bipartisan US Senate working group led by Majority Leader Sen. Chuck Schumer (D-NY), Sen. Mike Rounds (R-SD), Sen. Martin Heinrich (D-NM), and Sen. Todd Young (R-IN) released a report titled “Driving U.S. Innovation in Artificial Intelligence: A Roadmap for Artificial Intelligence Policy in the United States Senate.” The 31-page report follows a series of off-the-record “educational briefings,” including “the first ever all-senators classified briefing focused solely on AI,” and nine “AI Insight Forums” hosted in the fall of 2023 that drew on the participation of more than 150 experts from industry, academia, and civil society.
The report makes a number of recommendations on funding priorities, the development of new legislation, and areas that require further exploration. It also encourages the executive branch to share information “in a timely fashion and on an ongoing basis” about its AI priorities and “any AI-related Memorandums of Understanding with other countries and the results from any AI-related studies in order to better inform the legislative process.”…(More)”.
Artificial Intelligence and the Skill Premium
Paper by David E. Bloom et al: “How will the emergence of ChatGPT and other forms of artificial intelligence (AI) affect the skill premium? To address this question, we propose a nested constant elasticity of substitution production function that distinguishes among three types of capital: traditional physical capital (machines, assembly lines), industrial robots, and AI. Following the literature, we assume that industrial robots predominantly substitute for low-skill workers, whereas AI mainly helps to perform the tasks of high-skill workers. We show that AI reduces the skill premium as long as it is more substitutable for high-skill workers than low-skill workers are for high-skill workers…(More)”
QuantGov
About: “QuantGov is an open-source policy analytics platform designed to help create greater understanding and analysis of the breadth of government actions through quantifying policy text. By using the platform, researchers can quickly and effectively retrieve unique data that lies embedded in large bodies of text – data on text complexity, part of speech metrics, topic modeling, etc. …
QuantGov is a tool designed to make policy text more accessible. Think about it in terms of a hyper-powerful Google search that not only finds (1) specified content within massive quantities of text, but (2) also finds patterns and groupings and can even make predictions about what is in a document. Some recent use cases include the following:
- Analyzing state regulatory codes and predicting which parts of those codes are related to occupational licensing….And predicting which occupation the regulation is talking about….And determining the cost to receive the license.
- Analyzing Canadian province regulatory code while grouping individual regulations by industry-topic….And determining which Ministers are responsible for those regulations….And determining the complexity of the text for those regulation.
- Quantifying the number of tariff exclusions that exists due to the Trade Expansion Act of 1962 and recent tariff polices….And determining which products those exclusions target.
- Comparing the regulatory codes and content of 46 US states, 11 Canadian provinces, and 7 Australian states….While using consistent metrics that can lead to insights that provide legitimate policy improvements…(More)”.
Artificial intelligence and complex sustainability policy problems: translating promise into practice
Paper by Ruby O’Connor et al: “Addressing sustainability policy challenges requires tools that can navigate complexity for better policy processes and outcomes. Attention on Artificial Intelligence (AI) tools and expectations for their use by governments have dramatically increased over the past decade. We conducted a narrative review of academic and grey literature to investigate how AI tools are being used and adapted for policy and public sector decision-making. We found that academics, governments, and consultants expressed positive expectations about AI, arguing that AI could or should be used to address a wide range of policy challenges. However, there is much less evidence of how public decision makers are actually using AI tools or detailed insight into the outcomes of use. From our findings we draw four lessons for translating the promise of AI into practice: 1) Document and evaluate AI’s application to sustainability policy problems in the real-world; 2) Focus on existing and mature AI technologies, not speculative promises or external pressures; 3) Start with the problem to be solved, not the technology to be applied; and 4) Anticipate and adapt to the complexity of sustainability policy problems…(More)”.
Automatic Generation of Model and Data Cards: A Step Towards Responsible AI
Paper by Jiarui Liu, Wenkai Li, Zhijing Jin, Mona Diab: “In an era of model and data proliferation in machine learning/AI especially marked by the rapid advancement of open-sourced technologies, there arises a critical need for standardized consistent documentation. Our work addresses the information incompleteness in current human-generated model and data cards. We propose an automated generation approach using Large Language Models (LLMs). Our key contributions include the establishment of CardBench, a comprehensive dataset aggregated from over 4.8k model cards and 1.4k data cards, coupled with the development of the CardGen pipeline comprising a two-step retrieval process. Our approach exhibits enhanced completeness, objectivity, and faithfulness in generated model and data cards, a significant step in responsible AI documentation practices ensuring better accountability and traceability…(More)”.
Big data for everyone
Article by Henrietta Howells: “Raw neuroimaging data require further processing before they can be used for scientific or clinical research. Traditionally, this could be accomplished with a single powerful computer. However, much greater computing power is required to analyze the large open-access cohorts that are increasingly being released to the community. And processing pipelines are inconsistently scripted, which can hinder reproducibility efforts. This creates a barrier for labs lacking access to sufficient resources or technological support, potentially excluding them from neuroimaging research. A paper by Hayashi and colleagues in Nature Methods offers a solution. They present https://brainlife.io, a freely available, web-based platform for secure neuroimaging data access, processing, visualization and analysis. It leverages ‘opportunistic computing’, which pools processing power from commercial and academic clouds, making it accessible to scientists worldwide. This is a step towards lowering the barriers for entry into big data neuroimaging research…(More)”.
We don’t need an AI manifesto — we need a constitution
Article by Vivienne Ming: “Loans drive economic mobility in America, even as they’ve been a historically powerful tool for discrimination. I’ve worked on multiple projects to reduce that bias using AI. What I learnt, however, is that even if an algorithm works exactly as intended, it is still solely designed to optimise the financial returns to the lender who paid for it. The loan application process is already impenetrable to most, and now your hopes for home ownership or small business funding are dying in a 50-millisecond computation…
In law, the right to a lawyer and judicial review are a constitutional guarantee in the US and an established civil right throughout much of the world. These are the foundations of your civil liberties. When algorithms act as an expert witness, testifying against you but immune to cross examination, these rights are not simply eroded — they cease to exist.
People aren’t perfect. Neither ethics training for AI engineers nor legislation by woefully uninformed politicians can change that simple truth. I don’t need to assume that Big Tech chief executives are bad actors or that large companies are malevolent to understand that what is in their self-interest is not always in mine. The framers of the US Constitution recognised this simple truth and sought to leverage human nature for a greater good. The Constitution didn’t simply assume people would always act towards that greater good. Instead it defined a dynamic mechanism — self-interest and the balance of power — that would force compromise and good governance. Its vision of treating people as real actors rather than better angels produced one of the greatest frameworks for governance in history.
Imagine you were offered an AI-powered test for post-partum depression. My company developed that very test and it has the power to change your life, but you may choose not to use it for fear that we might sell the results to data brokers or activist politicians. You have a right to our AI acting solely for your health. It was for this reason I founded an independent non-profit, The Human Trust, that holds all of the data and runs all of the algorithms with sole fiduciary responsibility to you. No mother should have to choose between a life-saving medical test and her civil rights…(More)”.
A Fourth Wave of Open Data? Exploring the Spectrum of Scenarios for Open Data and Generative AI
Report by Hannah Chafetz, Sampriti Saxena, and Stefaan G. Verhulst: “Since late 2022, generative AI services and large language models (LLMs) have transformed how many individuals access, and process information. However, how generative AI and LLMs can be augmented with open data from official sources and how open data can be made more accessible with generative AI – potentially enabling a Fourth Wave of Open Data – remains an under explored area.
For these reasons, The Open Data Policy Lab (a collaboration between The GovLab and Microsoft) decided to explore the possible intersections between open data from official sources and generative AI. Throughout the last year, the team has conducted a range of research initiatives about the potential of open data and generative including a panel discussion, interviews, and Open Data Action Labs – a series of design sprints with a diverse group of industry experts.
These initiatives were used to inform our latest report, “A Fourth Wave of Open Data? Exploring the Spectrum of Scenarios for Open Data and Generative AI,” (May 2024) which provides a new framework and recommendations to support open data providers and other interested parties in making open data “ready” for generative AI…
The report outlines five scenarios in which open data from official sources (e.g. open government and open research data) and generative AI can intersect. Each of these scenarios includes case studies from the field and a specific set of requirements that open data providers can focus on to become ready for a scenario. These include…(More)” (Arxiv).
“Data Commons”: Under Threat by or The Solution for a Generative AI Era ? Rethinking Data Access and Re-us
Article by Stefaan G. Verhulst, Hannah Chafetz and Andrew Zahuranec: “One of the great paradoxes of our datafied era is that we live amid both unprecedented abundance and scarcity. Even as data grows more central to our ability to promote the public good, so too does it remain deeply — and perhaps increasingly — inaccessible and privately controlled. In response, there have been growing calls for “data commons” — pools of data that would be (self-)managed by distinctive communities or entities operating in the public’s interest. These pools could then be made accessible and reused for the common good.
Data commons are typically the results of collaborative and participatory approaches to data governance [1]. They offer an alternative to the growing tendency toward privatized data silos or extractive re-use of open data sets, instead emphasizing the communal and shared value of data — for example, by making data resources accessible in an ethical and sustainable way for purposes in alignment with community values or interests such as scientific research, social good initiatives, environmental monitoring, public health, and other domains.
Data commons can today be considered (the missing) critical infrastructure for leveraging data to advance societal wellbeing. When designed responsibly, they offer potential solutions for a variety of wicked problems, from climate change to pandemics and economic and social inequities. However, the rapid ascent of generative artificial intelligence (AI) technologies is changing the rules of the game, leading both to new opportunities as well as significant challenges for these communal data repositories.
On the one hand, generative AI has the potential to unlock new insights from data for a broader audience (through conversational interfaces such as chats), fostering innovation, and streamlining decision-making to serve the public interest. Generative AI also stands out in the realm of data governance due to its ability to reuse data at a massive scale, which has been a persistent challenge in many open data initiatives. On the other hand, generative AI raises uncomfortable questions related to equitable access, sustainability, and the ethical re-use of shared data resources. Further, without the right guardrails, funding models and enabling governance frameworks, data commons risk becoming data graveyards — vast repositories of unused, and largely unusable, data.
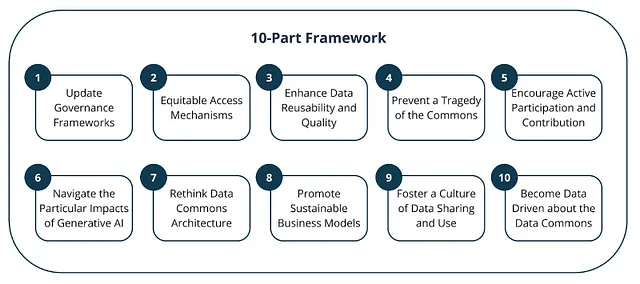
In what follows, we lay out some of the challenges and opportunities posed by generative AI for data commons. We then turn to a ten-part framework to set the stage for a broader exploration on how to reimagine and reinvigorate data commons for the generative AI era. This framework establishes a landscape for further investigation; our goal is not so much to define what an updated data commons would look like but to lay out pathways that would lead to a more meaningful assessment of the design requirements for resilient data commons in the age of generative AI…(More)”