Paper by Joshua S. Gans: “This paper surveys the relevant existing literature that can help researchers and policy makers understand the drivers of competition in markets that constitute the provision of artificial intelligence products. The focus is on three broad markets: training data, input data, and AI predictions. It is shown that a key factor in determining the emergence and persistence of market power will be the operation of markets for data that would allow for trading data across firm boundaries…(More)”.
Predicting IMF-Supported Programs: A Machine Learning Approach
Paper by Tsendsuren Batsuuri, Shan He, Ruofei Hu, Jonathan Leslie and Flora Lutz: “This study applies state-of-the-art machine learning (ML) techniques to forecast IMF-supported programs, analyzes the ML prediction results relative to traditional econometric approaches, explores non-linear relationships among predictors indicative of IMF-supported programs, and evaluates model robustness with regard to different feature sets and time periods. ML models consistently outperform traditional methods in out-of-sample prediction of new IMF-supported arrangements with key predictors that align well with the literature and show consensus across different algorithms. The analysis underscores the importance of incorporating a variety of external, fiscal, real, and financial features as well as institutional factors like membership in regional financing arrangements. The findings also highlight the varying influence of data processing choices such as feature selection, sampling techniques, and missing data imputation on the performance of different ML models and therefore indicate the usefulness of a flexible, algorithm-tailored approach. Additionally, the results reveal that models that are most effective in near and medium-term predictions may tend to underperform over the long term, thus illustrating the need for regular updates or more stable – albeit potentially near-term suboptimal – models when frequent updates are impractical…(More)”.
Facial Recognition Technology: Current Capabilities, Future Prospects, and Governance
Report by the National Academies of Sciences, Engineering, and Medicine: “Facial recognition technology is increasingly used for identity verification and identification, from aiding law enforcement investigations to identifying potential security threats at large venues. However, advances in this technology have outpaced laws and regulations, raising significant concerns related to equity, privacy, and civil liberties.
This report explores the current capabilities, future possibilities, and necessary governance for facial recognition technology. Facial Recognition Technology discusses legal, societal, and ethical implications of the technology, and recommends ways that federal agencies and others developing and deploying the technology can mitigate potential harms and enact more comprehensive safeguards…(More)”.
Why we’re fighting to make sure labor unions have a voice in how AI is implemented
Article by Liz Shuler and Mike Kubzansky: “Earlier this month, Google’s co-founder admitted that the company had “definitely messed up” after its AI tool, Gemini, produced historically inaccurate images—including depictions of racially diverse Nazis. Sergey Brin cited a lack of “thorough testing” of the AI tool, but the incident is a good reminder that, despite all the hype around generative AI replacing human output, the technology still has a long way to go.
Of course, that hasn’t stopped companies from deploying AI in the workplace. Some even use the technology as an excuse to lay workers off. Since last May, at least 4,000 people have lost their jobs to AI, and 70% of workers across the country live with the fear that AI is coming for theirs next. And while the technology may still be in its infancy, it’s developing fast. Earlier this year, AI pioneer Mustafa Suleyman said that “left completely to the market and to their own devices, [AI tools are] fundamentally labor-replacing.” Without changes now, AI could be coming to replace a lot of people’s jobs.
It doesn’t have to be this way. AI has enormous potential to build prosperity and unleash human creativity, but only if it also works for working people. Ensuring that happens requires giving the voice of workers—the people who will engage with these technologies every day, and whose lives, health, and livelihoods are increasingly affected by AI and automation—a seat at the decision-making table.
As president of the AFL-CIO, representing 12.5 million working people across 60 unions, and CEO of Omidyar Network, a social change philanthropy that supports responsible technology, we believe that the single best movement to give everyone a voice is the labor movement. Empowering workers—from warehouse associates to software engineers—is the most powerful tactic we have to ensure that AI develops in the interests of the many, not the few…(More)”.
Monitoring global trade using data on vessel traffic
Article by Graham Pilgrim, Emmanuelle Guidetti and Annabelle Mourougane: “Rising uncertainties and geo-political tensions, together with more complex trade relations have increased the demand for data and tools to monitor global trade in a timely manner. At the same time, advances in Big Data Analytics and access to a huge quantity of alternative data – outside the realm of official statistics – have opened new avenues to monitor trade. These data can help identify bottlenecks and disruptions in real time but need to be cleaned and validated.
One such alternative data source is the Automatic Identification System (AIS), developed by the International Maritime Organisation, facilitating the tracking of vessels across the globe. The system includes messages transmitted by ships to land or satellite receivers, available in quasi real time. While it was primarily designed to ensure vessel safety, this data is particularly well suited for providing insights on trade developments, as over 80% in volume of international merchandise trade is carried by sea (UNCTAD, 2022). Furthermore, AIS data holds granular vessel information and detailed location data, which combined with other data sources can enable the identification of activity at a port (or even berth) level, by vessel type or by the jurisdiction of vessel ownership.
For a number of years, the UN Global Platform has made AIS data available to those compiling official statistics, such as National Statistics Offices (NSOs) or International Organisations. This has facilitated the development of new methodologies, for instance the automated identification of port locations (Irish Central Statistics Office, 2022). The data has also been exploited by data scientists and research centres to monitor trade in specific commodities such as Liquefied Natural Gas (QuantCube Technology, 2022) or to analyse port and shipping operations in a specific country (Tsalamanis et al., 2018). Beyond trade, the dataset has been used to track CO2 emissions from the maritime sector (Clarke et al., 2023).
New work from the OECD Statistics and Data Directorate contributes to existing research in this field in two major ways. First, it proposes a new methodology to identify ports, at a higher level of precision than in past research. Second, it builds indicators to monitor port congestion and trends in maritime trade flows and provides a tool to get detailed information and better understand those flows…(More)”.
Commons-based Data Set: Governance for AI
Report by Open Future: “In this white paper, we propose an approach to sharing data sets for AI training as a public good governed as a commons. By adhering to the six principles of commons-based governance, data sets can be managed in a way that generates public value while making shared resources resilient to extraction or capture by commercial interests.
The purpose of defining these principles is two-fold:
We propose these principles as input into policy debates on data and AI governance. A commons-based approach can be introduced through regulatory means, funding and procurement rules, statements of principles, or data sharing frameworks. Secondly, these principles can also serve as a blueprint for the design of data sets that are governed and shared as a commons. To this end, we also provide practical examples of how these principles are being brought to life. Projects like Big Science or Common Voice have demonstrated that commons-based data sets can be successfully built.
These principles, tailored for the governance of AI data sets, are built on our previous work on Data Commons Primer. They are also the outcome of our research into the governance of AI datasets, including the AI_Commons case study. Finally, they are based on ongoing efforts to define how AI systems can be shared and made open, in which we have been participating – including the OSI-led process to define open-source AI systems, and the DPGA Community of Practice exploring AI systems as Digital Public Goods…(More)”.
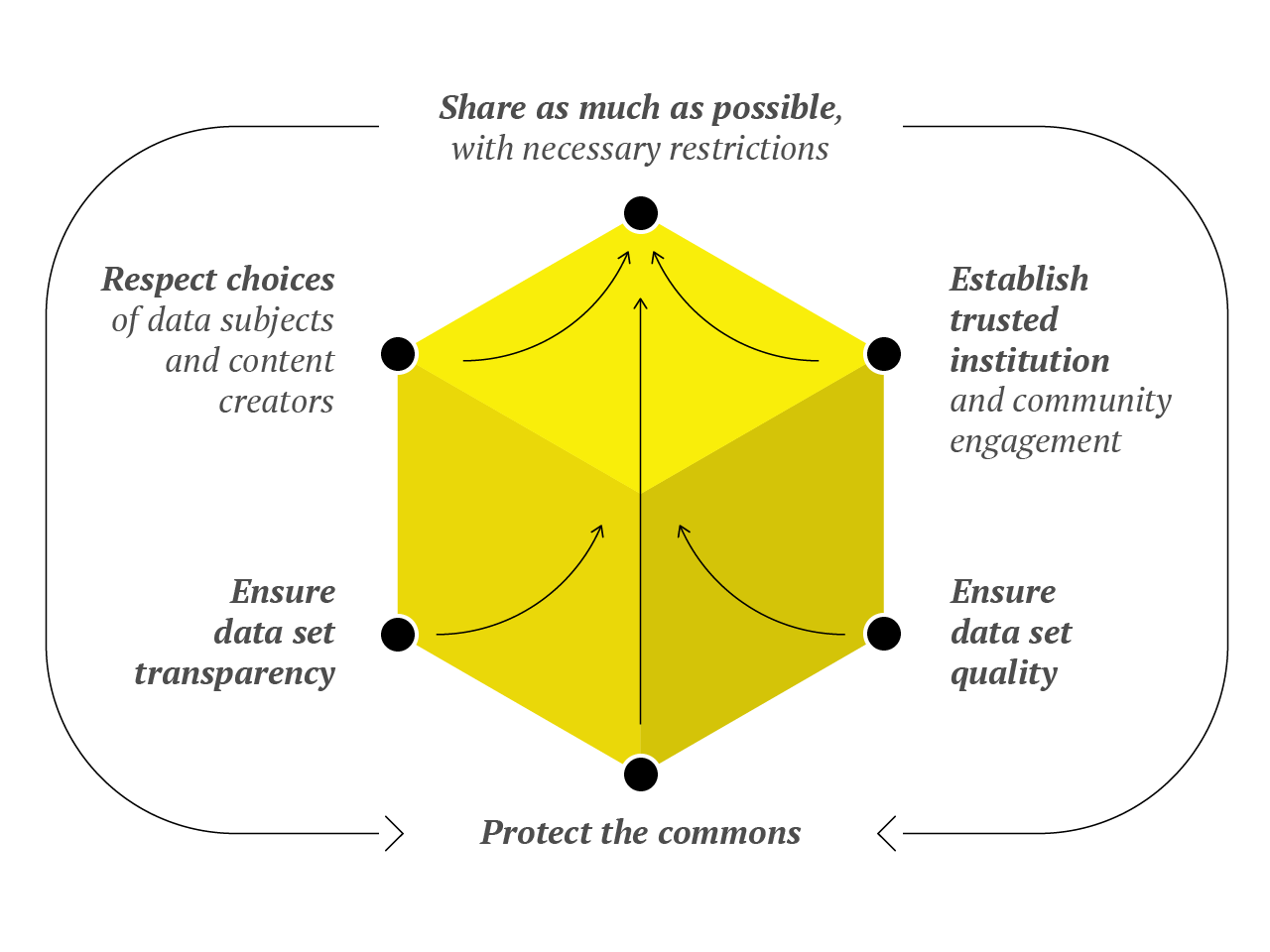
The six principles for commons-based data set governance are as follows:
Using online search activity for earlier detection of gynaecological malignancy
Paper by Jennifer F. Barcroft et al: Ovarian cancer is the most lethal and endometrial cancer the most common gynaecological cancer in the UK, yet neither have a screening program in place to facilitate early disease detection. The aim is to evaluate whether online search data can be used to differentiate between individuals with malignant and benign gynaecological diagnoses.
This is a prospective cohort study evaluating online search data in symptomatic individuals (Google user) referred from primary care (GP) with a suspected cancer to a London Hospital (UK) between December 2020 and June 2022. Informed written consent was obtained and online search data was extracted via Google takeout and anonymised. A health filter was applied to extract health-related terms for 24 months prior to GP referral. A predictive model (outcome: malignancy) was developed using (1) search queries (terms model) and (2) categorised search queries (categories model). Area under the ROC curve (AUC) was used to evaluate model performance. 844 women were approached, 652 were eligible to participate and 392 were recruited. Of those recruited, 108 did not complete enrollment, 12 withdrew and 37 were excluded as they did not track Google searches or had an empty search history, leaving a cohort of 235.s
The cohort had a median age of 53 years old (range 20–81) and a malignancy rate of 26.0%. There was a difference in online search data between those with a benign and malignant diagnosis, noted as early as 360 days in advance of GP referral, when search queries were used directly, but only 60 days in advance, when queries were divided into health categories. A model using online search data from patients (n = 153) who performed health-related search and corrected for sample size, achieved its highest sample-corrected AUC of 0.82, 60 days prior to GP referral.
Online search data appears to be different between individuals with malignant and benign gynaecological conditions, with a signal observed in advance of GP referral date. Online search data needs to be evaluated in a larger dataset to determine its value as an early disease detection tool and whether its use leads to improved clinical outcomes…(More)”.
Responsible Data Re-use in Developing Countries: Social Licence through Public Engagement
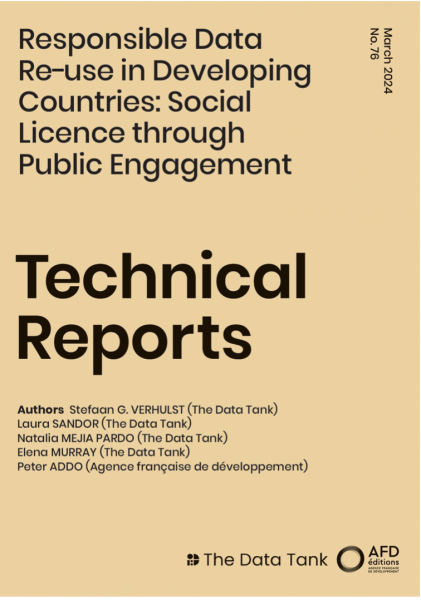
Report by Stefaan Verhulst, Laura Sandor, Natalia Mejia Pardo, Elena Murray and Peter Addo: “The datafication era has transformed the technological landscape, digitizing multiple areas of human life and offering opportunities for societal progress through the re-use of digital data. Developing countries stand to benefit from datafication but are faced with challenges like insufficient data quality and limited infrastructure. One of the primary obstacles to unlocking data re-use lies in agency asymmetries—disparities in decision-making authority among stakeholders—which fuel public distrust. Existing consent frameworks amplify the challenge, as they are individual-focused, lack information, and fail to address the nuances of data re-use. To address these limitations, a Social License for re-use becomes imperative—a community-focused approach that fosters responsible data practices and benefits all stakeholders. This shift is crucial for establishing trust and collaboration, and bridging the gap between institutions, governments, and citizens…(More)”.
Central banks use AI to assess climate-related risks
Article by Huw Jones: “Central bankers said on Tuesday they have broken new ground by using artificial intelligence to collect data for assessing climate-related financial risks, just as the volume of disclosures from banks and other companies is set to rise.
The Bank for International Settlements, a forum for central banks, the Bank of Spain, Germany’s Bundesbank and the European Central Bank said their experimental Gaia AI project was used to analyse company disclosures on carbon emissions, green bond issuance and voluntary net-zero commitments.
Regulators of banks, insurers and asset managers need high-quality data to assess the impact of climate-change on financial institutions. However, the absence of a single reporting standard confronts them with a patchwork of public information spread across text, tables and footnotes in annual reports.
Gaia was able to overcome differences in definitions and disclosure frameworks across jurisdictions to offer much-needed transparency, and make it easier to compare indicators on climate-related financial risks, the central banks said in a joint statement.
Despite variations in how the same data is reported by companies, Gaia focuses on the definition of each indicator, rather than how the data is labelled.
Furthermore, with the traditional approach, each additional key performance indicator, or KPI, and each new institution requires the analyst to either search for the information in public corporate reports or contact the institution for information…(More)”.
Data Disquiet: Concerns about the Governance of Data for Generative AI
Paper by Susan Aaronson: “The growing popularity of large language models (LLMs) has raised concerns about their accuracy. These chatbots can be used to provide information, but it may be tainted by errors or made-up or false information (hallucinations) caused by problematic data sets or incorrect assumptions made by the model. The questionable results produced by chatbots has led to growing disquiet among users, developers and policy makers. The author argues that policy makers need to develop a systemic approach to address these concerns. The current piecemeal approach does not reflect the complexity of LLMs or the magnitude of the data upon which they are based, therefore, the author recommends incentivizing greater transparency and accountability around data-set development…(More)”.