Manifesto by the Transatlantic Reflection Group on Democracy and the Rule of Law in the Age of ‘Artificial Intelligence’: “… calls for the effective and legitimate enforcement of laws concerning AI systems. In doing so, we recognise the important and complementary role of standards and compliance practices. Whereas the first manifesto focused on the relationship between democratic law-making and technology, this second manifesto shifts focus from the design of law in the age of AI to the enforcement of law. Concretely, we offer 10 recommendations for addressing the key enforcement challenges shared across transatlantic stakeholders. We call on those who support these recommendations to sign this manifesto…(More)”.
Using AI to support people with disability in the labour market
OECD Report: “People with disability face persisting difficulties in the labour market. There are concerns that AI, if managed poorly, could further exacerbate these challenges. Yet, AI also has the potential to create more inclusive and accommodating environments and might help remove some of the barriers faced by people with disability in the labour market. Building on interviews with more than 70 stakeholders, this report explores the potential of AI to foster employment for people with disability, accounting for both the transformative possibilities of AI-powered solutions and the risks attached to the increased use of AI for people with disability. It also identifies obstacles hindering the use of AI and discusses what governments could do to avoid the risks and seize the opportunities of using AI to support people with disability in the labour market…(More)”.
AI and Democracy’s Digital Identity Crisis
Paper by Shrey Jain, Connor Spelliscy, Samuel Vance-Law and Scott Moore: “AI-enabled tools have become sophisticated enough to allow a small number of individuals to run disinformation campaigns of an unprecedented scale. Privacy-preserving identity attestations can drastically reduce instances of impersonation and make disinformation easy to identify and potentially hinder. By understanding how identity attestations are positioned across the spectrum of decentralization, we can gain a better understanding of the costs and benefits of various attestations. In this paper, we discuss attestation types, including governmental, biometric, federated, and web of trust-based, and include examples such as e-Estonia, China’s social credit system, Worldcoin, OAuth, X (formerly Twitter), Gitcoin Passport, and EAS. We believe that the most resilient systems create an identity that evolves and is connected to a network of similarly evolving identities that verify one another. In this type of system, each entity contributes its respective credibility to the attestation process, creating a larger, more comprehensive set of attestations. We believe these systems could be the best approach to authenticating identity and protecting against some of the threats to democracy that AI can pose in the hands of malicious actors. However, governments will likely attempt to mitigate these risks by implementing centralized identity authentication systems; these centralized systems could themselves pose risks to the democratic processes they are built to defend. We therefore recommend that policymakers support the development of standards-setting organizations for identity, provide legal clarity for builders of decentralized tooling, and fund research critical to effective identity authentication systems…(More)”.
Transmission Versus Truth, Imitation Versus Innovation: What Children Can Do That Large Language and Language-and-Vision Models Cannot (Yet)
Paper by Eunice Yiu, Eliza Kosoy, and Alison Gopnik: “Much discussion about large language models and language-and-vision models has focused on whether these models are intelligent agents. We present an alternative perspective. First, we argue that these artificial intelligence (AI) models are cultural technologies that enhance cultural transmission and are efficient and powerful imitation engines. Second, we explore what AI models can tell us about imitation and innovation by testing whether they can be used to discover new tools and novel causal structures and contrasting their responses with those of human children. Our work serves as a first step in determining which particular representations and competences, as well as which kinds of knowledge or skills, can be derived from particular learning techniques and data. In particular, we explore which kinds of cognitive capacities can be enabled by statistical analysis of large-scale linguistic data. Critically, our findings suggest that machines may need more than large-scale language and image data to allow the kinds of innovation that a small child can produce…(More)”.
Public Value of Data: B2G data-sharing Within the Data Ecosystem of Helsinki
Paper by Vera Djakonoff: “Datafication penetrates all levels of society. In order to harness public value from an expanding pool of private-produced data, there has been growing interest in facilitating business-to-government (B2G) data-sharing. This research examines the development of B2G data-sharing within the data ecosystem of the City of Helsinki. The research has identified expectations ecosystem actors have for B2G data-sharing and factors that influence the city’s ability to unlock public value from private-produced data.
The research context is smart cities, with a specific focus on the City of Helsinki. Smart cities are in an advantageous position to develop novel public-private collaborations. Helsinki, on the international stage, stands out as a pioneer in the realm of data-driven smart city development. For this research, nine data ecosystem actors representing the city and companies participated in semi-structured thematic interviews through which their perceptions and experiences were mapped.
The theoretical framework of this research draws from the public value management (PVM) approach in examining the smart city data ecosystem and alignment of diverse interests for a shared purpose. Additionally, the research transcends the examination of the interests in isolation and looks at how technological artefacts shape the social context and interests surrounding them. Here, the focus is on the properties of data as an artefact with anti-rival value-generation potential.
The findings of this research reveal that while ecosystem actors recognise that more value can be drawn from data through collaboration, this is not apparent at the level of individual initiatives and transactions. This research shows that the city’s commitment to and facilitation of a long-term shared sense of direction and purpose among ecosystem actors is central to developing B2G data-sharing for public value outcomes. Here, participatory experimentation is key, promoting an understanding of the value of data and rendering visible the diverse motivations and concerns of ecosystem actors, enabling learning for wise, data-driven development…(More)”.
Can AI solve medical mysteries? It’s worth finding out
Article by Bina Venkataraman: “Since finding a primary care doctor these days takes longer than finding a decent used car, it’s little wonder that people turn to Google to probe what ails them. Be skeptical of anyone who claims to be above it. Though I was raised by scientists and routinely read medical journals out of curiosity, in recent months I’ve gone online to investigate causes of a lingering cough, ask how to get rid of wrist pain and look for ways to treat a bad jellyfish sting. (No, you don’t ask someone to urinate on it.)
Dabbling in self-diagnosis is becoming more robust now that people can go to chatbots powered by large language models scouring mountains of medical literature to yield answers in plain language — in multiple languages. What might an elevated inflammation marker in a blood test combined with pain in your left heel mean? The AI chatbots have some ideas. And researchers are finding that, when fed the right information, they’re often not wrong. Recently, one frustrated mother, whose son had seen 17 doctors for chronic pain, put his medical information into ChatGPT, which accurately suggested tethered cord syndrome — which then led a Michigan neurosurgeon to confirm an underlying diagnosis of spina bifida that could be helped by an operation.
The promise of this trend is that patients might be able to get to the bottom of mysterious ailments and undiagnosed illnesses by generating possible causes for their doctors to consider. The peril is that people may come to rely too much on these tools, trusting them more than medical professionals, and that our AI friends will fabricate medical evidence that misleads people about, say, the safety of vaccines or the benefits of bogus treatments. A question looming over the future of medicine is how to get the best of what artificial intelligence can offer us without the worst.
It’s in the diagnosis of rare diseases — which afflict an estimated 30 million Americans and hundreds of millions of people worldwide — that AI could almost certainly make things better. “Doctors are very good at dealing with the common things,” says Isaac Kohane, chair of the department of biomedical informatics at Harvard Medical School. “But there are literally thousands of diseases that most clinicians will have never seen or even have ever heard of.”..(More)”.
Interwoven Realms: Data Governance as the Bedrock for AI Governance
Essay by Stefaan G. Verhulst and Friederike Schüür: “In a world increasingly captivated by the opportunities and challenges of artificial intelligence (AI), there has been a surge in the establishment of committees, forums, and summits dedicated to AI governance. These platforms, while crucial, often overlook a fundamental pillar: the role of data governance. As we navigate through a plethora of discussions and debates on AI, this essay seeks to illuminate the often-ignored yet indispensable link between AI governance and robust data governance.
The current focus on AI governance, with its myriad ethical, legal, and societal implications, tends to sidestep the fact that effective AI governance is, at its core, reliant on the principles and practices of data governance. This oversight has resulted in a fragmented approach, leading to a scenario where the data and AI communities operate in isolation, often unaware of the essential synergy that should exist between them.
This essay delves into the intertwined nature of these two realms. It provides six reasons why AI governance is unattainable without a comprehensive and robust framework of data governance. In addressing this intersection, the essay aims to shed light on the necessity of integrating data governance more prominently into the conversation on AI, thereby fostering a more cohesive and effective approach to the governance of this transformative technology.
Six reasons why Data Governance is the bedrock for AI Governance...(More)”.
New York City Takes Aim at AI
Article by Samuel Greengard: “As concerns over artificial intelligence (AI) grow and angst about its potential impact increase, political leaders and government agencies are taking notice. In November, U.S. president Joe Biden issued an executive order designed to build guardrails around the technology. Meanwhile, the European Union (EU) is currently developing a legal framework around responsible AI.
Yet, what is often overlooked about artificial intelligence is that it’s more likely to impact people on a local level. AI touches housing, transportation, healthcare, policing and numerous other areas relating to business and daily life. It increasingly affects citizens, government employees, and businesses in both obvious and unintended ways.
One city attempting to position itself at the vanguard of AI is New York. In October 2023, New York City announced a blueprint for developing, managing, and using the technology responsibly. The New York City Artificial Intelligence Action Plan—the first of its kind in the U.S.—is designed to help officials and the public navigate the AI space.
“It’s a fairly comprehensive plan that addresses both the use of AI within city government and the responsible use of the technology,” says Clifford S. Stein, Wai T. Chang Professor of Industrial Engineering and Operations Research and Interim Director of the Data Science Institute at Columbia University.
Adds Stefaan Verhulst, co-founder and chief research and development officer at The GovLab and Senior Fellow at the Center for Democracy and Technology (CDT), “AI localism focuses on the idea that cities are where most of the action is in regard to AI.”…(More)”.
Updates to the OECD’s definition of an AI system explained
Article by Stuart Russell: “Obtaining consensus on a definition for an AI system in any sector or group of experts has proven to be a complicated task. However, if governments are to legislate and regulate AI, they need a definition to act as a foundation. Given the global nature of AI, if all governments can agree on the same definition, it allows for interoperability across jurisdictions.
Recently, OECD member countries approved a revised version of the Organisation’s definition of an AI system. We published the definition on LinkedIn, which, to our surprise, received an unprecedented number of comments.
We want to respond better to the interest our community has shown in the definition with a short explanation of the rationale behind the update and the definition itself. Later this year, we can share even more details once they are finalised.
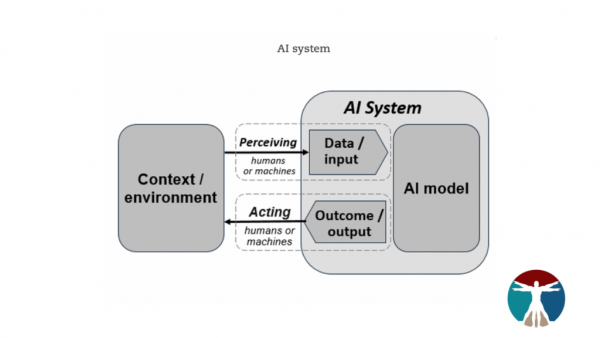
How OECD countries updated the definition
Here are the revisions to the current text of the definition of “AI System” in detail, with additions set out in bold and subtractions in strikethrough):
An AI system is a machine-based system that can, for a given set of human-defined explicit or implicit objectives, infers, from the input it receives, how to generate outputs such as makes predictions, content, recommendations, or decisions that can influenceing physical real or virtual environments. Different AI systems are designed to operate with varying in their levels of autonomy and adaptiveness after deployment…(More)”
Elon Musk is now taking applications for data to study X — but only EU risk researchers need apply…
Article by Natasha Lomas: “Lawmakers take note: Elon Musk-owned X appears to have quietly complied with a hard legal requirement in the European Union that requires larger platforms (aka VLOPs) to provide researchers with data access in order to study systemic risks arising from use of their services — risks such as disinformation, child safety issues, gender-based violence and mental heath concerns.
X (or Twitter as it was still called at the time) was designated a VLOP under the EU’s Digital Services Act (DSA) back in April after the bloc’s regulators confirmed it meets their criteria for an extra layer of rules to kick in that are intended to drive algorithmic accountability via applying transparency measures on larger platforms.
Researchers intending to study systemic risks in the EU now appear to at least be able to apply for access to study X’s data by accessing a web form through a button which appears at the bottom of this page on its developer platform. (Note researchers can be based in the EU but don’t have to be to meet the criteria; they just need to intend to study systemic risks in the EU.)…(More)”.