Article by Nicola Jones: “The Internet is a vast ocean of human knowledge, but it isn’t infinite. And artificial intelligence (AI) researchers have nearly sucked it dry.
The past decade of explosive improvement in AI has been driven in large part by making neural networks bigger and training them on ever-more data. This scaling has proved surprisingly effective at making large language models (LLMs) — such as those that power the chatbot ChatGPT — both more capable of replicating conversational language and of developing emergent properties such as reasoning. But some specialists say that we are now approaching the limits of scaling. That’s in part because of the ballooning energy requirements for computing. But it’s also because LLM developers are running out of the conventional data sets used to train their models.
A prominent study1 made headlines this year by putting a number on this problem: researchers at Epoch AI, a virtual research institute, projected that, by around 2028, the typical size of data set used to train an AI model will reach the same size as the total estimated stock of public online text. In other words, AI is likely to run out of training data in about four years’ time (see ‘Running out of data’). At the same time, data owners — such as newspaper publishers — are starting to crack down on how their content can be used, tightening access even more. That’s causing a crisis in the size of the ‘data commons’, says Shayne Longpre, an AI researcher at the Massachusetts Institute of Technology in Cambridge who leads the Data Provenance Initiative, a grass-roots organization that conducts audits of AI data sets.
The imminent bottleneck in training data could be starting to pinch. “I strongly suspect that’s already happening,” says Longpre…(More)”
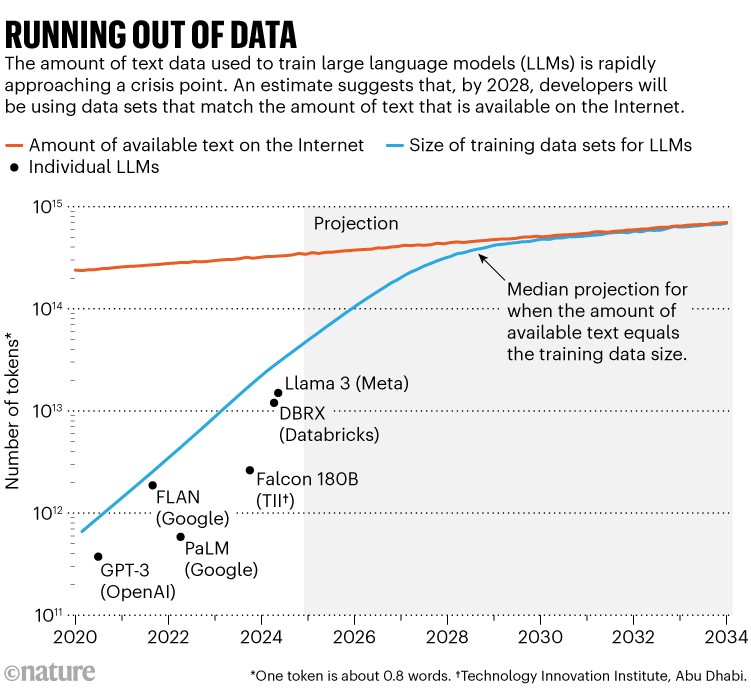