Paper by Sara Marcucci and Stefaan Verhulst: “The increasing complexity of global challenges, such as climate change, public health crises, and socioeconomic inequalities, underscores the need for a more sophisticated and adaptive policymaking approach. Evidence-Informed Decision-Making (EIDM) has emerged as a critical framework, leveraging data and research to guide policy design, implementation, and impact assessment. However, traditional evidence-based approaches, such as reliance on Randomized Controlled Trials (RCTs) and systematic reviews, face limitations, including resource intensity, contextual constraints, and difficulty in addressing real-time challenges. Artificial Intelligence offers transformative potential to enhance EIDM by enabling large-scale data analysis, pattern recognition, predictive modeling, and stakeholder engagement across the policy cycle. While generative AI has attracted significant attention, this paper emphasizes the broader spectrum of AI applications (beyond Generative AI) —such as natural language processing (NLP), decision trees, and basic machine learning algorithms—that continue to play a critical role in evidence-informed policymaking. These models, often more transparent and resource-efficient, remain highly relevant in supporting data analysis, policy simulations, and decision-support.
This paper explores AI’s role in three key phases of the policy cycle: (1) problem identification, where AI can support issue framing, trend detection, and scenario creation; (2) policy design, where AI-driven simulations and decision-support tools can improve solution alignment with real-world contexts; and (3) policy implementation and impact assessment, where AI can enhance monitoring, evaluation, and adaptive decision-making. Despite its promise, AI adoption in policymaking remains limited due to challenges such as algorithmic bias, lack of explainability, resource demands, and ethical concerns related to data privacy and environmental impact. To ensure responsible and effective AI integration, this paper highlights key recommendations: prioritizing augmentation over automation, embedding human oversight throughout AI-driven processes, facilitating policy iteration, and combining AI with participatory governance models…(More)”.
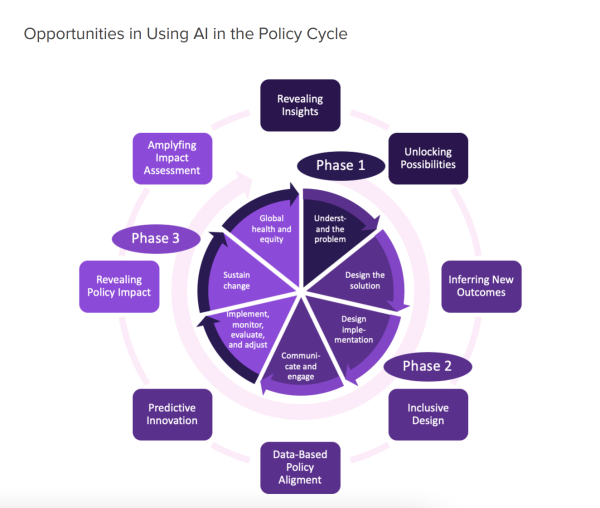