Book by Michael Heller and James Salzman: “A hidden set of rules governs who owns what–explaining everything from whether you can recline your airplane seat to why HBO lets you borrow a password illegally–and in this lively and entertaining guide, two acclaimed law professors reveal how things become “mine.”
“Mine” is one of the first words babies learn. By the time we grow up, the idea of ownership seems natural, whether buying a cup of coffee or a house. But who controls the space behind your airplane seat: you reclining or the squished laptop user behind? Why is plagiarism wrong, but it’s okay to knock-off a recipe or a dress design? And after a snowstorm, why does a chair in the street hold your parking space in Chicago, but in New York you lose the space and the chair?
Mine! explains these puzzles and many more. Surprisingly, there are just six simple stories that everyone uses to claim everything. Owners choose the story that steers us to do what they want. But we can always pick a different story. This is true not just for airplane seats, but also for battles over digital privacy, climate change, and wealth inequality. As Michael Heller and James Salzman show–in the spirited style of Freakonomics, Nudge, and Predictably Irrational–ownership is always up for grabs.
With stories that are eye-opening, mind-bending, and sometimes infuriating, Mine! reveals the rules of ownership that secretly control our lives….(More)”.
The Use of Mobility Data for Responding to the COVID-19 Pandemic
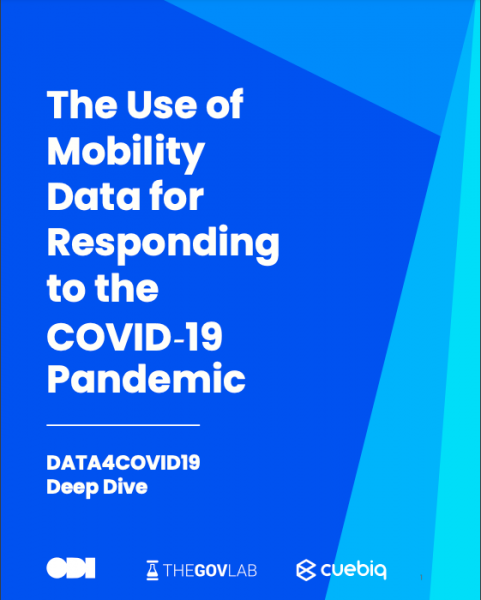
New Report, Repository and set of Case Studies commissioned by the Open Data Institute: “…The GovLab and Cuebiq firstly assembled a repository of mobility data collaboratives related to Covid-19. They then selected five of these to analyse further, and produced case studies on each of the collaboratives (which you can find below in the ‘Key outputs’ section).
After analysing these initiatives, Cuebiq and The GovLab then developed a synthesis report, which contains sections focused on:
- Mobility data – what it is and how it can be used
- Current practice – insights from five case studies
- Prescriptive analysis – recommendations for the future
Findings and recommendations
Based on this analysis, the authors of the report recommend nine actions which have the potential to enable more effective, sustainable and responsible re-use of mobility data through data collaboration to support decision making regarding pandemic prevention, monitoring, and response:
- Developing and clarifying governance framework to enable the trusted, transparent, and accountable reuse of privately held data in the public interest under a clear regulatory framework
- Building capacity of organisations in the public and private sector to reuse and act on data through investments in training, education, and reskilling of relevant authorities; especially driving support for institutions in the Global South
- Establishing data stewards in organisations who can coordinate and collaborate with counterparts on using data in the public’s interest and acting on it.
- Establishing dedicated and sustainable CSR (Corporate Social Responsibility) programs on data in organisations to coordinate and collaborate with counterparts on using and acting upon data in the public’s interest.
- Building a network of data stewards to coordinate and streamline efforts while promoting greater transparency; as well as exchange best practices and lessons learned.
- Engaging citizens about how their data is being used so clearly articulate how they want their data to be responsibly used, shared, and protected.
- Promoting technological innovation through collaboration between funders (eg governments and foundations) and researchers (eg data scientists) to develop and deploy useful, privacy-preserving technologies.
- Unlocking funds from a variety of sources to ensure projects are sustainable and can operate long term.
- Increase research and spur evidence gathering by publishing easily accessible research and creating dedicated centres to develop best practices.
This research begins to demonstrate the value that a handful of new data-sharing initiatives have had in the ongoing response to Covid-19. The pandemic isn’t yet over, and we will need to continue to assess and evaluate how data has been shared – both successfully and unsuccessfully – and who has benefited or been harmed in the process. More research is needed to highlight the lessons from this emergency that can be applied to future crises….(More)”.
Financing the Digital Public Goods Ecosystem
Blog by the Digital Public Goods Alliance (DPGA): “… we believe that digital public goods (DPGs) are essential to unlocking the full potential of digital technologies to enhance human welfare at scale. Their relevance to one or multiple sustainable development goals (SDGs), combined with their adoptability and adaptability, allows DPGs to strengthen international digital cooperation. Stakeholders can join forces to support solutions that address many of today’s greatest global challenges in critical areas such as health, education and climate change. DPGs are of particular importance for resource constrained countries looking to accelerate development through improving access to digital services.
Still, precisely due to their nature as “public goods” – which ensures that no one can prevent others from benefiting from them – DPGs can be difficult to fund through market mechanisms, and some of them should not have to prioritise generating profit….
Sustainably funded infrastructural DPGs can become a reliable core for broader ecosystems through community building:
- For the Modular Open Source Identity Platform (MOSIP) core code management and evolution is fully funded by grants from a group of philanthropic and bilateral donors.** This enables the team responsible for managing and evolving the generic platform to focus exclusively on maximising utility for those the platform is designed to serve – in this case, countries in need of foundational digital identity systems.
- Similarly backed by grant funding for core code development and maintenance, the team behind District Health Information Software 2 (DHIS2) has prioritised community building within and between the 70+ countries that have adopted the software, enabling countries to share improvements and related innovations. This is best exemplified by Sri Lanka, the first country in the world to use DHIS2 for COVID-19 surveillance, who shared this groundbreaking innovation with the global DHIS2 community. Today, this system is operational in 38 countries and is under development in fourteen more.
- The data exchange layer X-Road, which is publicly funded by NIIS members (currently Estonia and Finland), demonstrates how infrastructural DPGs can use community building to advance both the core technology and the quality of downstream deployments. The X-Road Community connects a diverse group of individuals and allows anyone to contribute to the open-source technology. This community-based support and knowledge-sharing helps local vendors around the world build the expertise needed to provide quality services to stakeholders adopting the technology….(More)”.
Connecting parliaments: Harnessing digital dividends to increase transparency and citizen engagement
Paper by Julia Keutgen and Rebecca Rumbul: “…The overarching argument of this paper is that parliamentary digital transformation is a relatively underfunded area of work, but a vitally important one in achieving the very common overarching goals of open, accountable, inclusive and participative government. Improvements in how parliamentary digital capacity building can be done better are possible with better strategy, funding and cooperation, and when parliaments are enthusiastic and willing to take the opportunities offered to them to improve themselves.
Now more than ever, digital transformation has become essential for parliaments. Such transformation can have a significant impact in making parliaments more transparent and accountable and can enable them to leverage greater public interest and engagement in the legislative and electoral processes.
Good external digital engagement requires parliaments to review their own internal digital structures, assess where development and investment are needed, and how digital improvement will assist in achieving their goals. Differential priorities in the needs of the parliament or societal actors can form a guide, according to which specific areas for digital development might be prioritised. These steps require long-term investment, which should go in parallel with the digital transformation of the Executive. However, because a country’s digital transformation is primarily the preserve of the Executive, it can bypass the legislature and may be almost disproportionately influenced by the ruling party. Uneven digital transformation between public bodies and the legislature may weaken the profile and legitimacy of the legislature itself. Furthermore, governments that effectively restrict digital development within the legislature are essentially restricting democratic integrity.
Besides the long-term process of building and developing infrastructure, short-term pilot projects can be useful to test approaches and begin building the digital infrastructure of the future. Properly targeted funding, to achieve specified digital transformation goals, agreed in collaboration with the development agencies operating in target areas, can yield significant dividends in improving the digital democracy ecosystem. This approach can neutralise harmful, short-termist and wasteful approaches to digital deficiency, and remove the ability of the more unscrupulous parliaments to play development agencies off against each other to leverage greater rewards or resources.
Digital transformation of parliaments requires better strategy, funding and cooperation on the part of donors and implementers as parliaments are enthusiastic and willing to take the opportunities offered by digitalisation….(More)”.
Open data in action: initiatives during the initial stage of the COVID-19 pandemic
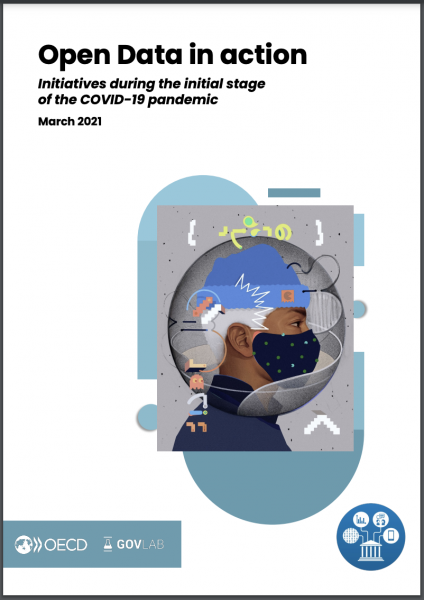
Report by OECD and The GovLab: “The COVID-19 pandemic has increased the demand for access to timely, relevant, and quality data. This demand has been driven by several needs: taking informed policy actions quickly, improving communication on the current state of play, carrying out scientific analysis of a dynamic threat, understanding its social and economic impact, and enabling civil society oversight and reporting.
This report…assesses how open government data (OGD) was used to react and respond to the COVID-19 pandemic during initial stage of the crisis (March-July 2020) based on initiatives collected through an open call for evidence. It also seeks to transform lessons learned into considerations for policy makers on how to improve OGD policies to better prepare for future shocks…(More)”.
The (Im)possibility of Fairness: Different Value Systems Require Different Mechanisms For Fair Decision Making
Sorelle A. Friedler, Carlos Scheidegger, Suresh Venkatasubramanian at Communications of the ACM: “Automated decision-making systems (often machine learning-based) now commonly determine criminal sentences, hiring choices, and loan applications. This widespread deployment is concerning, since these systems have the potential to discriminate against people based on their demographic characteristics. Current sentencing risk assessments are racially biased, and job advertisements discriminate on gender. These concerns have led to an explosive growth in fairness-aware machine learning, a field that aims to enable algorithmic systems that are fair by design.
To design fair systems, we must agree precisely on what it means to be fair. One such definition is individual fairness: individuals who are similar (with respect to some task) should be treated similarly (with respect to that task). Simultaneously, a different definition states that demographic groups should, on the whole, receive similar decisions. This group fairness definition is inspired by civil rights law in the U.S. and U.K. Other definitions state that fair systems should err evenly across demographic groups. Many of these definitions have been incorporated into machine learning pipelines.
In this article, we introduce a framework for understanding these different definitions of fairness and how they relate to each other. Crucially, our framework shows these definitions and their implementations correspond to different axiomatic beliefs about the world. We present two such worldviews and will show they are fundamentally incompatible. First, one can believe the observation processes that generate data for machine learning are structurally biased. This belief provides a justification for seeking non-discrimination. When one believes that demographic groups are, on the whole, fundamentally similar, group fairness mechanisms successfully guarantee the top-level goal of non-discrimination: similar groups receiving similar treatment. Alternatively, one can assume the observed data generally reflects the true underlying reality about differences between people. These worldviews are in conflict; a single algorithm cannot satisfy either definition of fairness under both worldviews. Thus, researchers and practitioners ought to be intentional and explicit about world-views and value assumptions: the systems they design will always encode some belief about the world….(More)”.
The World Happiness Report 2021
Report by the Sustainable Development Solutions Network: “There has been surprising resilience in how people rate their lives overall. The Gallup World Poll data are confirmed for Europe by the separate Eurobarometer surveys and several national surveys.
- The change from 2017-2019 to 2020 varied considerably among countries, but not enough to change rankings in any significant fashion materially. The same countries remain at the top.
- Emotions changed more than did life satisfaction during the first year of COVID-19, worsening more during lockdown and recovering faster, as illustrated by large samples of UK data. For the world as a whole, based on the annual data from the Gallup World Poll, there was no overall change in positive affect, but there was a roughly 10% increase in the number of people who said they were worried or sad the previous day.
- Trust and the ability to count on others are major supports to life evaluations, especially in the face of crises. To feel that your lost wallet would be returned if found by a police officer, by a neighbour, or a stranger, is estimated to be more important for happiness than income, unemployment, and major health risks (see Figure 2.4 in chapter 2)
- Trust is even more important in explaining the very large international differences in COVID-19 death rates, which were substantially higher in the Americas and Europe than in East Asia, Australasia, and Africa, as shown here (see Figure 2.5 of chapter 2). These differences were almost half due to differences in the age structure of populations (COVID-19 much more deadly for the old), whether the country is an island, and how exposed each country was, early in the pandemic, to large numbers of infections in nearby countries. Whatever the initial circumstances, the most effective strategy for controlling COVID-19 was to drive community transmission to zero and to keep it there. Countries adopting this strategy had death rates close to zero, and were able to avoid deadly second waves, and ended the year with less loss of income and lower death rates.
- Factors supporting successful COVID-19 strategies include
- confidence in public institutions. Trusted public institutions were more likely to choose the right strategy and have their populations support the required actions. For example, Brazil’s death rate was 93 per 100,000, higher than in Singapore, and of this difference, over a third could be explained by the difference in public trust….(More)”
Coming wave of video games could build empathy on racism, environment and aftermath of war
Mike Snider at USA Today: “Some of the newest video games in development aren’t really games at all, but experiences that seek to build empathy for others.
Among the five such projects getting funding grants and support from 3D software engine maker Unity is “Our America,” in which the player takes the role of a Black man who is driving with his son when their car is pulled over by a police officer.
The father worries about getting his car registration from the glove compartment because the officer “might think it’s a gun or something,” the character says in the trailer.
On the project’s website, the developers describe “Our America” as “an autobiographical VR Experience” in which “the audience must make quick decisions, answer questions – but any wrong move is the difference between life and death.”…
The other Unity for Humanity winners include:
- Ahi Kā Rangers: An ecological mobile game with development led by Māori creators.
- Dot’s Home: A game that explores historical housing injustices faced by Black and brown home buyers.
- Future Aleppo: A VR experience for children to rebuild homes and cities destroyed by war.
- Samudra: A children’s environmental puzzle game that takes the player across a polluted sea to learn about pollution and plastic waste.
While “Our America” may serve best as a VR experience, other projects such as “Dot’s Home” may be available on mobile devices to expand its accessibility….(More)”.
European Data Economy: Between Competition and Regulation
Report by René Arnold, Christian Hildebrandt, and Serpil Taş: “Data and its economic impact permeates all sectors of the economy. The data economy is not a new sector, but more like a challenge for all firms to compete and innovate as part of a new wave of economic value creation.
With data playing an increasingly important role across all sectors of the economy, the results of this report point European policymakers to promote the development and adoption of unified reference architectures. These architectures constitute a technology-neutral and cross-sectoral approach that will enable companies small and large to compete and to innovate—unlocking the economic potential of data capture in an increasingly digitized world.
Data access appears to be less of a hindrance to a thriving data economy due to the net increase in capabilities in data capture, elevation, and analysis. What does prove difficult for firms is discovering existing datasets and establishing their suitability for achieving their economic objectives. Reference architectures can facilitate this process as they provide a framework to locate potential providers of relevant datasets and carry sufficient additional information (metadata) about datasets to enable firms to understand whether a particular dataset, or parts of it, fits their purpose.
Whether third-party data access is suitable to solve a specific business task in the first place ought to be a decision at the discretion of the economic actors involved. As our report underscores, data captured in one context with a specific purpose may not be fit for another context or another purpose. Consequently, a firm has to evaluate case-by-case whether first-party data capture, third-party data access, or a mixed approach is the best solution. This evaluation will naturally depend on whether there is any other firm capturing data suitable for the task that is willing to negotiate conditions for third-party access to this data. Unified data architectures may also lower the barriers for a firm capturing suitable data to engage in negotiations, since its adoption will lower the costs of making the data ready for a successful exchange. Such architectures may further integrate licensing provisions ensuring that data, once exchanged, is not used beyond the agreed purpose. It can also bring in functions that improve the discoverability of potential data providers….(More)”.
How can we measure productivity in the public sector?
Ravi Somani at the World Bank: “In most economies, the public sector is a major purchaser of goods, services and labor. According to the Worldwide Bureaucracy Indicators, globally the public sector accounts for around 25% of GDP and 38% of formal employment. Generating efficiency gains in the public sector can, therefore, have important implications for a country’s overall economic performance.
Public-sector productivity measures the rate with which inputs are converted into desirable outputs in the public sector. Measures can be developed at the level of the employee, organization, or overall public sector, and can be tracked over time. Such information allows policymakers to identify good and bad performers, understand what might be correlated with good performance, and measure the returns to different types of public expenditures. This knowledge can be used to improve the allocation of public resources in the future and maximize the impact of the public purse.
But how can we measure it?
However, measuring productivity in the public sector can be tricky because:
- There are often no market transactions for public services, or they are distorted by subsidies and other market imperfections.
- Many public services are complex, requiring (often immeasurable) inputs from multiple individuals and organizations.
- There is often a substantial time lag between investments in inputs and the realization of outputs and outcomes.
This recent World Bank publication provides a summary of the different approaches to measuring productivity in the public sector, presented in the table below. For simplicity, the approaches are separated into: ‘macro’ approaches, which provide aggregate information at the level of an organization, sector, or service as a whole; and ‘micro’ approaches, which can be applied to the individual employee, task, project, and process.
There is no silver bullet for accurately measuring public-sector productivity – each approach has its own limitations. For example, the cost-weighted-output approach requires activity-level data, necessitates different approaches for different sectors, and results in metrics with difficult-to-interpret absolute levels. Project-completion rates require access to project-level data and may not fully account for differences in the quality and complexity of projects. The publication includes a list of the pros, cons, and implementation requirements for each approach….(More)”.