Paper by Meghan J. Ryan: “Burgeoning science and technology have provided the criminal justice system with the opportunity to address some of its shortcomings. And the criminal justice system has significant shortcomings. Among other issues, we have a mass incarceration problem; clearance rates are surprisingly low; there are serious concerns about wrongful convictions; and the system is layered with racial, religious, and other biases. Innovations that are widely used across industries, as well as those directed specifically at the criminal justice system, have the potential to improve upon such problems. But it is important to recognize that these innovations also have downsides, and criminal justice actors must proceed with caution and understand not only the potential of these interventions but also their limitations. Relevant to this calculation of caution is whether the innovation is broadly used across industry sectors or, rather, whether it has been specifically developed for use within the criminal justice system. These latter innovations have a record of not being sufficiently vetted for accuracy and reliability. Accordingly, criminal justice actors must be sufficiently well versed in basic science and technology so that they have the ability and the confidence to critically assess the usefulness of the various criminal justice innovations in light of their limitations. Considering lawyers’ general lack of competency in these areas, scientific and technological training is necessary to mold them into modern competent criminal justice actors. This training must be more than superficial subject-specific training, though; it must dig deeper, delving into critical thinking skills that include evaluating the accuracy and reliability of the innovation at issue, as well as assessing broader concerns such as the need for development transparency, possible intrusions on individual privacy, and incentives to curtail individual liberties given the innovation at hand….(More)”
New laws to strengthen Canadians’ privacy protection and trust in the digital economy
Press Release: “Canadians increasingly rely on digital technology to connect with loved ones, to work and to innovate. That’s why the Government of Canada is committed to making sure Canadians can benefit from the latest technologies, knowing that their personal information is safe and secure and that companies are acting responsibly.
Today, the Honourable François-Philippe Champagne, Minister of Innovation, Science and Industry, together with the Honourable David Lametti, Minister of Justice and Attorney General of Canada, introduced the Digital Charter Implementation Act, 2022, which will significantly strengthen Canada’s private sector privacy law, create new rules for the responsible development and use of artificial intelligence (AI), and continue advancing the implementation of Canada’s Digital Charter. As such, the Digital Charter Implementation Act, 2022 will include three proposed acts: the Consumer Privacy Protection Act, the Personal Information and Data Protection Tribunal Act, and the Artificial Intelligence and Data Act.
The proposed Consumer Privacy Protection Act will address the needs of Canadians who rely on digital technology and respond to feedback received on previous proposed legislation. This law will ensure that the privacy of Canadians will be protected and that innovative businesses can benefit from clear rules as technology continues to evolve. This includes:
- increasing control and transparency when Canadians’ personal information is handled by organizations;
- giving Canadians the freedom to move their information from one organization to another in a secure manner;
- ensuring that Canadians can request that their information be disposed of when it is no longer needed;
- establishing stronger protections for minors, including by limiting organizations’ right to collect or use information on minors and holding organizations to a higher standard when handling minors’ information;
- providing the Privacy Commissioner of Canada with broad order-making powers, including the ability to order a company to stop collecting data or using personal information; and
- establishing significant fines for non-compliant organizations—with fines of up to 5% of global revenue or $25 million, whichever is greater, for the most serious offences.
The proposed Personal Information and Data Protection Tribunal Act will enable the creation of a new tribunal to facilitate the enforcement of the Consumer Privacy Protection Act.
The proposed Artificial Intelligence and Data Act will introduce new rules to strengthen Canadians’ trust in the development and deployment of AI systems, including:
- protecting Canadians by ensuring high-impact AI systems are developed and deployed in a way that identifies, assesses and mitigates the risks of harm and bias;
- establishing an AI and Data Commissioner to support the Minister of Innovation, Science and Industry in fulfilling ministerial responsibilities under the Act, including by monitoring company compliance, ordering third-party audits, and sharing information with other regulators and enforcers as appropriate; and
- outlining clear criminal prohibitions and penalties regarding the use of data obtained unlawfully for AI development or where the reckless deployment of AI poses serious harm and where there is fraudulent intent to cause substantial economic loss through its deployment…(More)”.
How Period-Tracker Apps Treat Your Data, and What That Means if Roe v. Wade Is Overturned
Article by Nicole Nguyen and Cordilia James: “You might not talk to your friends about your monthly cycle, but there’s a good chance you talk to an app about it. And why not? Period-tracking apps are more convenient than using a diary, and the insights are more interesting, too.
But how much do you know about the ways apps and trackers collect, store—and sometimes share—your fertility and menstrual-cycle data?
The question has taken on new importance following the leak of a draft Supreme Court opinion that would overturn Roe v. Wade. Roe established a constitutional right to abortion, and should the court reverse its 1973 decision, about half the states in the U.S. are likely to restrict or outright ban the procedure.
Phone and app data have long been shared and sold without prominent disclosure, often for advertising purposes. HIPAA, aka the Health Insurance Portability and Accountability Act, might protect information shared between you and your healthcare provider, but it doesn’t typically apply to data you put into an app, even a health-related one. Flo Health Inc., maker of a popular period and ovulation tracker, settled with the Federal Trade Commission in 2021 for sharing sensitive health data with Facebook without making the practice clear to users.
The company completed an independent privacy audit earlier this year. “We remain committed to ensuring the utmost privacy for our users and want to make it clear that Flo does not share health data with any company,” a spokeswoman said.
In a scenario where Roe is overturned, your digital breadcrumbs—including the kind that come from period trackers—could be used against you in states where laws criminalize aiding in or undergoing abortion, say legal experts.
“The importance of menstrual data is not merely speculative. It has been relevant to the government before, in investigations and restrictions,” said Leah Fowler, research director at University of Houston’s Health Law and Policy Institute. She cited a 2019 hearing where Missouri’s state health department admitted to keeping a spreadsheet of Planned Parenthood abortion patients, which included the dates of their last menstrual period.
Prosecutors have also obtained other types of digital information, including text messages and search histories, as evidence for abortion-related cases…(More)”.
Machine Learning Can Predict Shooting Victimization Well Enough to Help Prevent It
Paper by Sara B. Heller, Benjamin Jakubowski, Zubin Jelveh & Max Kapustin: “This paper shows that shootings are predictable enough to be preventable. Using arrest and victimization records for almost 644,000 people from the Chicago Police Department, we train a machine learning model to predict the risk of being shot in the next 18 months. We address central concerns about police data and algorithmic bias by predicting shooting victimization rather than arrest, which we show accurately captures risk differences across demographic groups despite bias in the predictors. Out-of-sample accuracy is strikingly high: of the 500 people with the highest predicted risk, 13 percent are shot within 18 months, a rate 130 times higher than the average Chicagoan. Although Black male victims more often have enough police contact to generate predictions, those predictions are not, on average, inflated; the demographic composition of predicted and actual shooting victims is almost identical. There are legal, ethical, and practical barriers to using these predictions to target law enforcement. But using them to target social services could have enormous preventive benefits: predictive accuracy among the top 500 people justifies spending up to $123,500 per person for an intervention that could cut their risk of being shot in half….(More)”.
Digital Government Model
Report by USAID: “The COVID-19 pandemic demonstrated the importance of digital government processes and tools. Governments with digital systems, processes, and infrastructure in place were able to quickly scale emergency response assistance, communications, and payments. At the same time, the pandemic accelerated many risks associated with digital tools, such as mis- and disinformation, surveillance, and the exploitation of personal data.
USAID and development partners are increasingly supporting countries in the process of adopting technologies to create public value– broadly referred to as digital government–while mitigating and avoiding risks. The Digital Government Model provides a basis for establishing a shared understanding and language on the core components of digital government, including the contextual considerations and foundational elements that influence the success of digital government investments…(More)”
Sweeping Legislation Aims to Ban the Sale of Location Data
Article by Joseph Cox and Liz Landers: “Sen. Elizabeth Warren and a group of other Democratic lawmakers have introduced a bill that would essentially outlaw the sale of location data harvested from smartphones. The bill also presents a range of other powers to the Federal Trade Commission (FTC) and individual victims to push back against the multibillion-dollar location data industry.
The move comes after Motherboard reported multiple instances in which companies were selling location data of people who visited abortion clinics, and sometimes making subsets of that data freely available. Such data has taken on a new significance in the wake of the Supreme Court’s looming vote on whether to overturn the protections offered by Roe v. Wade. The bill also follows a wave of reporting from Motherboard and others on various abuses and data sales in the location data industry writ large.
“Data brokers profit from the location data of millions of people, posing serious risks to Americans everywhere by selling their most private information,” Warren told Motherboard in a statement. “With this extremist Supreme Court poised to overturn Roe v. Wade and states seeking to criminalize essential health care, it is more crucial than ever for Congress to protect consumers’ sensitive data. The Health and Location Data Protection Act will ban brokers from selling Americans’ location and health data, rein in giant data brokers, and set some long overdue rules of the road for this $200 billion industry.”…(More)”.
How the Federal Government Buys Our Cell Phone Location Data
Article by Bennett Cyphers: “…Weather apps, navigation apps, coupon apps, and “family safety” apps often request location access in order to enable key features. But once an app has location access, it typically has free rein to share that access with just about anyone.
That’s where the location data broker industry comes in. Data brokers entice app developers with cash-for-data deals, often paying per user for direct access to their device. Developers can add bits of code called “software development kits,” or SDKs, from location brokers into their apps. Once installed, a broker’s SDK is able to gather data whenever the app itself has access to it: sometimes, that means access to location data whenever the app is open. In other cases, it means “background” access to data whenever the phone is on, even if the app is closed.
One app developer received the following marketing email from data broker Safegraph:
SafeGraph can monetize between $1-$4 per user per year on exhaust data (across location, matches, segments, and other strategies) for US mobile users who have strong data records. We already partner with several GPS apps with great success, so I would definitely like to explore if a data partnership indeed makes sense.
But brokers are not limited to data from apps they partner with directly. The ad tech ecosystem provides ample opportunities for interested parties to skim from the torrents of personal information that are broadcast during advertising auctions. In a nutshell, advertising monetization companies (like Google) partner with apps to serve ads. As part of the process, they collect data about users—including location, if available—and share that data with hundreds of different companies representing digital advertisers. Each of these companies uses that data to decide what ad space to bid on, which is a nasty enough practice on its own. But since these “bidstream” data flows are largely unregulated, the companies are also free to collect the data as it rushes past and store it for later use.
The data brokers covered in this post add another layer of misdirection to the mix. Some of them may gather data from apps or advertising exchanges directly, but others acquire data exclusively from other data brokers. For example, Babel Street reportedly purchases all of its data from Venntel. Venntel, in turn, acquires much of its data from its parent company, the marketing-oriented data broker Gravy Analytics. And Gravy Analytics has purchased access to data from the brokers Complementics, Predicio, and Mobilewalla. We have little information about where those companies get their data—but some of it may be coming from any of the dozens of other companies in the business of buying and selling location data.
If you’re looking for an answer to “which apps are sharing data?”, the answer is: “It’s almost impossible to know.” Reporting, technical analysis, and right-to-know requests through laws like GDPR have revealed relationships between a handful of apps and location data brokers. For example, we know that the apps Muslim Pro and Muslim Mingle sold data to X-Mode, and that navigation app developer Sygic sent data to Predicio (which sold it to Gravy Analytics and Venntel). However, this is just the tip of the iceberg. Each of the location brokers discussed in this post obtains data from hundreds or thousands of different sources. Venntel alone has claimed to gather data from “over 80,000” different apps. Because much of its data comes from other brokers, most of these apps likely have no direct relationship with Venntel. As a result, the developers of the apps fueling this industry likely have no idea where their users’ data ends up. Users, in turn, have little hope of understanding whether and how their data arrives in these data brokers’ hands…(More)”.
Roadside safety messages increase crashes by distracting drivers
Article by Jonathan Hall and Joshua Madsen: “Behavioural interventions involve gently suggesting that people reconsider or change specific undesirable behaviours. They are a low-cost, easy-to-implement and increasingly common tool used by policymakers to encourage socially desirable behaviours.
Examples of behavioural interventions include telling people how their electricity usage compares to their neighbours or sending text messages reminding people to pay fines.
Many of these interventions are expressly designed to “seize people’s attention” at a time when they can take the desired action. Unfortunately, seizing people’s attention can crowd out other, more important considerations, and cause even a simple intervention to backfire with costly individual and social consequences.
One such behavioural intervention struck us as odd: Several U.S. states display year-to-date fatality statistics (number of deaths) on roadside dynamic message signs (DMSs). The hope is that these sobering messages will reduce traffic crashes, a leading cause of death of five- to 29-year-olds worldwide. Perhaps because of its low cost and ease of implementation, at least 28 U.S. states have displayed fatality statistics at least once since 2012. We estimate that approximately 90 million drivers have been exposed to such messages.
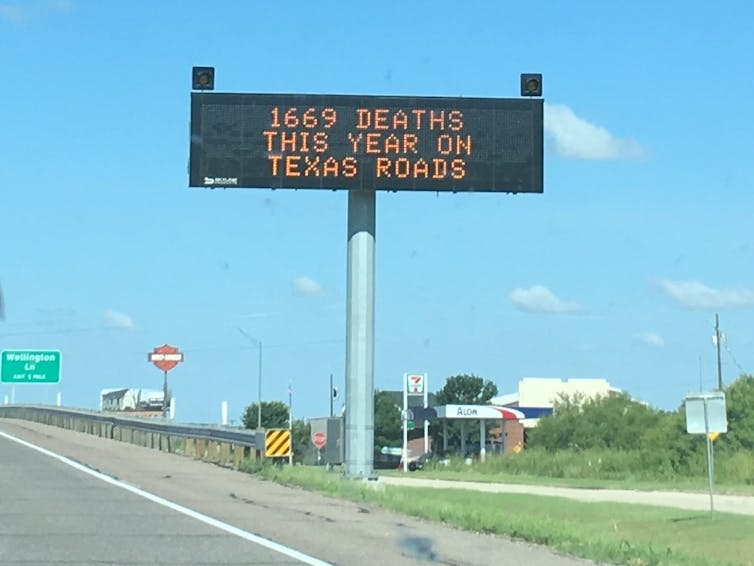
Startling results
As academic researchers with backgrounds in information disclosure and transportation policy, we teamed up to investigate and quantify the effects of these messages. What we found startled us.
Contrary to policymakers’ expectations (and ours), we found that displaying fatality messages increases the number of crashes…(More)”.
Reimagining the Request for Proposal
Article by Devon Davey, Heather Hiscox & Nicole Markwick : “In recent years, the social sector and the communities it serves have called for deep structural change to address our most serious social injustices. Yet one of the basic tools we use to fund change, the request for proposal (RFP), has remained largely unchanged. We believe that RFPs must become part of the larger call for systemic reform….
At first glance, the RFP process may seem neutral or fair. Yet RFPs are often designed by individuals in high-level positions without meaningful input from community members and frontline staff—those who are most familiar with social injustices and who often hold the least institutional power. What’s more, those who both issue and respond to RFPs often rely on their social capital to find and collaborate on RFP opportunities. Since social networks are highly homogeneous, RFP participation is limited to the professionals who have social connections to the issuer, resulting in a more limited pool of applicants.
This selection process is further compounded by the human propensity to hire people who look the same and who reflect similar ways of thinking. Social sector decision makers and power holders tend to be—among other identities—white. This lack of diversity, furthered by historical oppression, has ensured that white privilege and ways of working have come to dominate within the philanthropic and nonprofit sectors. This concentration of power and lack of diverse perspectives and experiences shaping RFPs results in projects failing to respond to the needs of communities and, in many cases, projects that directly perpetuate racism, colonialism, misogyny, ableism, sexism, and other forms of systemic and individual oppression.
The rigid structure of RFPs plays an important role in many of the negative outcomes of projects. Effective social change work is emergent, is iterative, and centers trust by nature. By contrast, RFPs frequently apply inflexible work scopes, limited timelines and budgets, and unproven solutions that are developed within the blinders of institutional power. Too often, funders force programs into implementation because they want to see results according to a specified plan. This rigidity can produce initiatives that are ineffective and removed from community needs. As consultant Joyce Lee-Ibarra says, “[RFPs] feel fundamentally transactional, when the work I want to do is relational.”…(More)”.
Imagining Governance for Emerging Technologies
Essay by Debra J.H. Mathews, Rachel Fabi and Anaeze C. Offodile: “…How should such technologies be regulated and governed? It is increasingly clear that past governance structures and strategies are not up to the task. What these technologies require is a new governance approach that accounts for their interdisciplinary impacts and potential for both good and ill at both the individual and societal level.
To help lay the groundwork for a novel governance framework that will enable policymakers to better understand these technologies’ cross-sectoral footprint and anticipate and address the social, legal, ethical, and governance issues they raise, our team worked under the auspices of the National Academy of Medicine’s Committee on Emerging Science, Technology, and Innovation in health and medicine (CESTI) to develop an analytical approach to technology impacts and governance. The approach is grounded in detailed case studies—including the vignettes about Robyn and Liam—which have informed the development of a set of guiding principles (see sidebar).
Based on careful analysis of past governance, these case studies also contain a plausible vision of what might happen in the future. They illuminate ethical issues and help reveal governance tools and choices that could be crucial to delivering social benefits and reducing or avoiding harms. We believe that the approach taken by the committee will be widely applicable to considering the governance of emerging health technologies. Our methodology and process, as we describe here, may also be useful to a range of stakeholders involved in governance issues like these…(More)”.