Paper by Fabian Kalleitner, David W Schiestl, Georg Heiler: “Human mobility has become a major variable of interest during the COVID-19 pandemic and central to policy decisions all around the world. To measure individual mobility, research relies on a variety of indicators that commonly stem from two main data sources: survey self-reports and behavioral mobility data from mobile phones. However, little is known about how mobility from survey self-reports relates to popular mobility estimates using data from the Global System for Mobile Communications (GSM) and the Global Positioning System (GPS). Spanning March 2020 until April 2021, this study compares self-reported mobility from a panel survey in Austria to aggregated mobility estimates utilizing (1) GSM data and (2) Google’s GPS-based Community Mobility Reports. Our analyses show that correlations in mobility changes over time are high, both in general and when comparing subgroups by age, gender, and mobility category. However, while these trends are similar, the size of relative mobility changes over time differs substantially between different mobility estimates. Overall, while our findings suggest that these mobility estimates manage to capture similar latent variables, especially when focusing on changes in mobility over time, researchers should be aware of the specific form of mobility different data sources capture….(More)”.
The Data4COVID-19 Review: Assessing the Use of Non-Traditional Data During a Pandemic Crisis
Report by Hannah Chafetz, Andrew J. Zahuranec, Sara Marcucci, Behruz Davletov, and Stefaan Verhulst: “As the last two years of the COVID-19 pandemic demonstrate, pandemics pose major challenges on all levels–with cataclysmic effects on society.
Decision-makers from around the world have sought to mitigate the consequences of COVID-19 through the use of data, including data from non-traditional sources such as social media, wastewater, and credit card and telecommunications companies. However, there has been little research into how non-traditional data initiatives were designed or what impacts they had on COVID-19 responses.
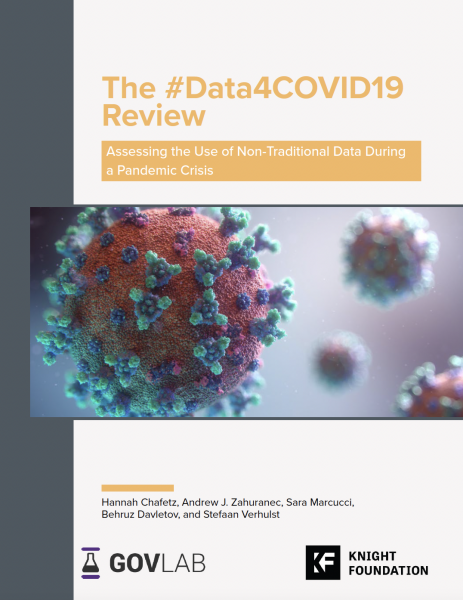
Over the last eight months, The GovLab, with the support of The Knight Foundation, has sought to fill this gap by conducting a study about how non-traditional data (NTD) sources have been used during COVID-19.
On October 31st, The GovLab published the report: “The COVID-19 Review: Assessing the Use of Non-Traditional Data During a Pandemic Crisis.” The report details how decision makers around the world have used non-traditional sources through a series of briefings intended for a generalist audience.
The briefings describe and assess how non-traditional data initiatives were designed, planned, and implemented, as well as the project results.
Findings
The briefings uncovered several findings about why, where, when, and how NTD was used during COVID-19, including that:
- Officials increasingly called for the use of NTD to answer questions where and when traditional data such as surveys and case data were not sufficient or could not be leveraged. However, the collection and use of traditional data was often needed to validate insights.
- NTD sources were primarily used to understand populations’ health, mobility (or physical movements), economic activity, and sentiment of the pandemic. In comparison with previous dynamic crises, COVID-19 was a watershed moment in terms of access to and re-use of non-traditional data in those four areas.
- The majority of NTD initiatives were fragmented and uncoordinated, reflecting the larger fragmented COVID-19 response. Many projects were focused on responding to COVID-19 after outbreaks occurred. This pattern reflected an overall lack of preparedness for the pandemic and need for the rapid development of initiatives to address its consequences.
- NTD initiatives frequently took the form of cross-sectoral data partnerships or collaborations developed to respond to specific needs. Many institutions did not have the systems and infrastructure in place for these collaborations to be sustainable.
- Many of the NTD initiatives involving granular, personal data were implemented without the necessary social license to do so–leading to public concerns about ethics and hindering public trust in non-traditional data.
Stefaan Verhulst, Co-Founder and Chief R&D of The GovLab explains: “The use of NTD offers growing potential during crisis situations. When managed responsibly, NTD use can help us understand the current state of the crisis, forecast how it will progress, and respond to different aspects of it in real-time.”…(More)”.
Sex and Gender Bias in Technology and Artificial Intelligence
Book edited by Davide Cirillo, Silvina Catuara Solarz, and Emre Guney: “…details the integration of sex and gender as critical factors in innovative technologies (artificial intelligence, digital medicine, natural language processing, robotics) for biomedicine and healthcare applications. By systematically reviewing existing scientific literature, a multidisciplinary group of international experts analyze diverse aspects of the complex relationship between sex and gender, health and technology, providing a perspective overview of the pressing need of an ethically-informed science. The reader is guided through the latest implementations and insights in technological areas of accelerated growth, putting forward the neglected and overlooked aspects of sex and gender in biomedical research and healthcare solutions that leverage artificial intelligence, biosensors, and personalized medicine approaches to predict and prevent disease outcomes. The reader comes away with a critical understanding of this fundamental issue for the sake of better future technologies and more effective clinical approaches….(More)”.
Could an algorithm predict the next pandemic?
Article by Simon Makin: “Leap is a machine-learning algorithm that uses sequence data to classify influenza viruses as either avian or human. The model had been trained on a huge number of influenza genomes — including examples of H5N8 — to learn the differences between those that infect people and those that infect birds. But the model had never seen an H5N8 virus categorized as human, and Carlson was curious to see what it made of this new subtype.
Somewhat surprisingly, the model identified it as human with 99.7% confidence. Rather than simply reiterating patterns in its training data, such as the fact that H5N8 viruses do not typically infect people, the model seemed to have inferred some biological signature of compatibility with humans. “It’s stunning that the model worked,” says Carlson. “But it’s one data point; it would be more stunning if I could do it a thousand more times.”
The zoonotic process of viruses jumping from wildlife to people causes most pandemics. As climate change and human encroachment on animal habitats increase the frequency of these events, understanding zoonoses is crucial to efforts to prevent pandemics, or at least to be better prepared.
Researchers estimate that around 1% of the mammalian viruses on the planet have been identified1, so some scientists have attempted to expand our knowledge of this global virome by sampling wildlife. This is a huge task, but over the past decade or so, a new discipline has emerged — one in which researchers use statistical models and machine learning to predict aspects of disease emergence, such as global hotspots, likely animal hosts or the ability of a particular virus to infect humans. Advocates of such ‘zoonotic risk prediction’ technology argue that it will allow us to better target surveillance to the right areas and situations, and guide the development of vaccines and therapeutics that are most likely to be needed.
However, some researchers are sceptical of the ability of predictive technology to cope with the scale and ever-changing nature of the virome. Efforts to improve the models and the data they rely on are under way, but these tools will need to be a part of a broader effort if they are to mitigate future pandemics…(More)”.
Charting an Equity-Centered Public Health Data System
Introduction to Special Issue by Alonzo L. Plough: “…The articles in this special issue were written with that vision in mind; several of them even informed the commission’s deliberations. Each article addresses an issue essential to the challenge of building an equity-focused public health data system:
- Why Equity Matters in Public Health Data. Authors Anita Chandra, Laurie T. Martin, Joie D. Acosta, Christopher Nelson, Douglas Yeung, Nabeel Qureshi, and Tara Blagg explore where and how equity has been lacking in public health data and the implications of considering equity to the tech and data sectors.
- What is Public Health Data? As authors Joie D. Acosta, Anita Chandra, Douglas Yeung, Christopher Nelson, Nabeel Qureshi, Tara Blagg, and Laurie T. Martin explain, good public health data are more than just health data. We need to reimagine the types of data we collect and from where, as well data precision, granularity, timeliness, and more.
- Public Health Data and Special Populations. People of color, women, people with disabilities, and people who are lesbian, gay bisexual trans-gendered queer are among the populations that have been inconsistently represented in public health data over time. This article by authors Tina J. Kauh and Maryam Khojasteh reviews findings for each population, as well as commonalities across populations.
- Public health data interoperability and connectedness. What are challenges to connecting public health data swiftly yet accurately? What gaps need to be filled? How can the data and tech sector help address these issues? These are some of the questions explored in this article by authors Laurie T. Martin, Christopher Nelson, Douglas Yeung, Joie D. Acosta, Nabeel Qureshi, Tara Blagg, and Anita Chandra.
- Integrating Tech and Data Expertise into the Public Health Workforce. This article by authors Laurie T. Martin, Anita Chandra, Christopher Nelson, Douglas Yeung, Joie D. Acosta, Nabeel Qureshi, and Tara Blag envisions what a tech-savvy public health workforce will look like and how it can be achieved through new workforce models, opportunities to expand capacity, and training….(More)”.
Innovative Data Science Approaches to Identify Individuals, Populations, and Communities at High Risk for Suicide
Report by the National Academies of Sciences, Engineering, and Medicine: “Emerging real-time data sources, together with innovative data science techniques and methods – including artificial intelligence and machine learning – can help inform upstream suicide prevention efforts. Select social media platforms have proactively deployed these methods to identify individual platform users at high risk for suicide, and in some cases may activate local law enforcement, if needed, to prevent imminent suicide. To explore the current scope of activities, benefits, and risks of leveraging innovative data science techniques to help inform upstream suicide prevention at the individual and population level, the Forum on Mental Health and Substance Use Disorders of the National Academies of Sciences, Engineering, and Medicine convened a virtual workshop series consisting of three webinars held on April 28, May 12, and June 30, 2022. This Proceedings highlights presentations and discussions from the workshop…(More)”
Nudging the Nudger: A Field Experiment on the Effect of Performance Feedback to Service Agents on Increasing Organ Donor Registrations
Paper by Julian House, Nicola Lacetera, Mario Macis & Nina Mazar: “We conducted a randomized controlled trial involving nearly 700 customer-service representatives (CSRs) in a Canadian government service agency to study whether providing CSRs with performance feedback with or without peer comparison affected their subsequent organ donor registration rates. Despite having no tie to remuneration or promotion, the provision of individual performance feedback three times over one year resulted in a 25% increase in daily signups, compared to otherwise similar encouragement and reminders. Adding benchmark information that compared CSRs performance to average and top peer performance did not further enhance this effect. Registrations increased more among CSRs whose performance was already above average, and there was no negative effect on lower-performing CSRs. A post-intervention survey showed that CSRs found the information included in the treatments helpful and encouraging. However, performance feedback without benchmark information increased perceived pressure to perform…(More)”.
Global healthcare fairness: We should be sharing more, not less, data
Paper by Kenneth P. Seastedt et al: “The availability of large, deidentified health datasets has enabled significant innovation in using machine learning (ML) to better understand patients and their diseases. However, questions remain regarding the true privacy of this data, patient control over their data, and how we regulate data sharing in a way that does not encumber progress or further potentiate biases for underrepresented populations. After reviewing the literature on potential reidentifications of patients in publicly available datasets, we argue that the cost—measured in terms of access to future medical innovations and clinical software—of slowing ML progress is too great to limit sharing data through large publicly available databases for concerns of imperfect data anonymization. This cost is especially great for developing countries where the barriers preventing inclusion in such databases will continue to rise, further excluding these populations and increasing existing biases that favor high-income countries. Preventing artificial intelligence’s progress towards precision medicine and sliding back to clinical practice dogma may pose a larger threat than concerns of potential patient reidentification within publicly available datasets. While the risk to patient privacy should be minimized, we believe this risk will never be zero, and society has to determine an acceptable risk threshold below which data sharing can occur—for the benefit of a global medical knowledge system….(More)”.
Can Smartphones Help Predict Suicide?
Ellen Barry in The New York Times: “In March, Katelin Cruz left her latest psychiatric hospitalization with a familiar mix of feelings. She was, on the one hand, relieved to leave the ward, where aides took away her shoelaces and sometimes followed her into the shower to ensure that she would not harm herself.
But her life on the outside was as unsettled as ever, she said in an interview, with a stack of unpaid bills and no permanent home. It was easy to slide back into suicidal thoughts. For fragile patients, the weeks after discharge from a psychiatric facility are a notoriously difficult period, with a suicide rate around 15 times the national rate, according to one study.
This time, however, Ms. Cruz, 29, left the hospital as part of a vast research project which attempts to use advances in artificial intelligence to do something that has eluded psychiatrists for centuries: to predict who is likely to attempt suicide and when that person is likely to attempt it, and then, to intervene.
On her wrist, she wore a Fitbit programmed to track her sleep and physical activity. On her smartphone, an app was collecting data about her moods, her movement and her social interactions. Each device was providing a continuous stream of information to a team of researchers on the 12th floor of the William James Building, which houses Harvard’s psychology department.
In the field of mental health, few new areas generate as much excitement as machine learning, which uses computer algorithms to better predict human behavior. There is, at the same time, exploding interest in biosensors that can track a person’s mood in real time, factoring in music choices, social media posts, facial expression and vocal expression.
Matthew K. Nock, a Harvard psychologist who is one of the nation’s top suicide researchers, hopes to knit these technologies together into a kind of early-warning system that could be used when an at-risk patient is released from the hospital…(More)”.
Call it data liberation day: Patients can now access all their health records digitally
Article by Casey Ross: “The American Revolution had July 4. The allies had D-Day. And now U.S. patients, held down for decades by information hoarders, can rally around a new turning point, October 6, 2022 — the day they got their health data back.
Under federal rules taking effect Thursday, health care organizations must give patients unfettered access to their full health records in digital format. No more long delays. No more fax machines. No more exorbitant charges for printed pages.
Just the data, please — now…The new federal rules — passed under the 21st Century Cures Act — are designed to shift the balance of power to ensure that patients can not only get their data, but also choose who else to share it with. It is the jumping-off point for a patient-mediated data economy that lets consumers in health care benefit from the fluidity they’ve had for decades in banking: they can move their information easily and electronically, and link their accounts to new services and software applications.
“To think that we actually have greater transparency about our personal finances than about our own health is quite an indictment,” said Isaac Kohane, a professor of biomedical informatics at Harvard Medical School. “This will go some distance toward reversing that.”
Even with the rules now in place, health data experts said change will not be fast or easy. Providers and other data holders — who have dug in their heels at every step — can still withhold information under certain exceptions. And many questions remain about protocols for sharing digital records, how to verify access rights, and even what it means to give patients all their data. Does that extend to every measurement in the ICU? Every log entry? Every email? And how will it all get standardized?…(More)”