CALL FOR ACTION: “The spread of COVID-19 is a human tragedy and a worldwide crisis. The social and economic costs are huge, and they are contributing to a global slowdown. Despite the amount of data collected daily, we have not been able to leverage them to accelerate our understanding and action to counter COVID-19. As a result we have entered a global state of profound uncertainty and anxiety.
The current pandemic has not only shown vulnerabilities in our public health systems but has also made visible our failure to re-use data between the public and private sectors — what we call data collaboratives — to inform decision makers how to fight dynamic threats like the novel Coronavirus.
We have known for years that the re-use of aggregated and anonymized data — including from telecommunications, social media, and satellite feeds — can improve traditional models for tracking disease propagation. Telecommunications data has, for instance, been re-used to support the response to Ebola in Africa (Orange) and swine flu in Mexico (Telefónica). Social media data has been re-used to understand public perceptions around Zika in Brazil (Facebook). Satellite data has been used to track seasonal measles in Niger using nighttime lights. Geospatial data has similarly supported malaria surveillance and eradication efforts in Sub-Saharan Africa. In general, many infectious diseases have been monitored using mobile phones and mobility.
The potential and realized contributions of these and other data collaboratives reveal that the supply of and demand for data and data expertise are widely dispersed. They are spread across government, the private sector, and civil society and often poorly matched.
Much data needed by researchers is never made accessible to those who could productively put it to use while much data that is released is never used in a systematic and sustainable way during and post crisis.
This failure results in tremendous inefficiencies and costly delays in how we respond. It means lost opportunities to save lives and a persistent lack of preparation for future threats….(More)”. SIGN AND JOIN HERE.
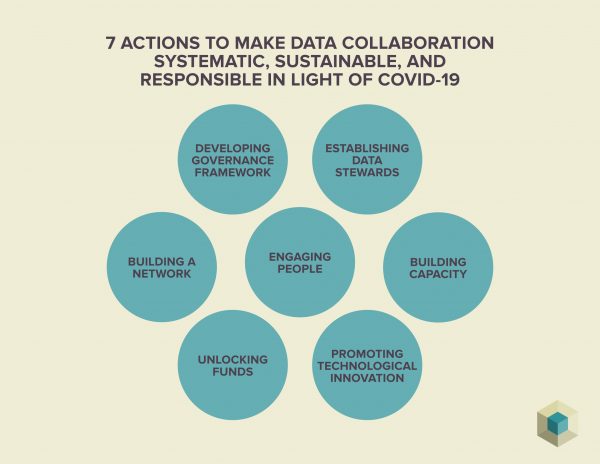