Nick Paumgarten at the New Yorker: “…Parcak is a pioneer in the use of remote sensing, via satellite, to find and map potential locations that would otherwise be invisible to us. Variations in the chemical composition of the earth reveal the ghost shadows of ancient walls and citadels, watercourses and planting fields. The nifty kid-friendly name for all this is “archeology from space,” which also happens to be the title of Parcak’s new book. That’s a bit of a misnomer, because, technically, the satellites in question are in the mid-troposphere, and also the archeology still happens on, or under, the ground. In spite of the whiz-bang abracadabra of the multispectral imagery, Parcak is, at heart, a shovel bum…..Another estimate of Parcak’s, based on satellite data: there are roughly fifty million unmapped archeological sites around the world. Many, if not most, will be gone or corrupted by 2040, she says, the threats being not just looting but urban development, illegal construction, and climate change. In 2016, Parcak won the ted Prize, a grant of a million dollars; she used it to launch a project called GlobalXplorer, a crowdsourcing platform, by which citizen Indiana Joneses can scrutinize satellite maps and identify potential new sites, adding these to a database without publicly revealing the coördinates. The idea is to deploy more eyeballs (and, ultimately, more benevolent shovel bums) in the race against carbon and greed….(More)”.
The war to free science
Brian Resnick and Julia Belluz at Vox: “The 27,500 scientists who work for the University of California generate 10 percent of all the academic research papers published in the United States.
Their university recently put them in a strange position: Sometime this year, these scientists will not be able to directly access much of the world’s published research they’re not involved in.
That’s because in February, the UC system — one of the country’s largest academic institutions, encompassing Berkeley, Los Angeles, Davis, and several other campuses — dropped its nearly $11 million annual subscription to Elsevier, the world’s largest publisher of academic journals.
On the face of it, this seemed like an odd move. Why cut off students and researchers from academic research?
In fact, it was a principled stance that may herald a revolution in the way science is shared around the world.
The University of California decided it doesn’t want scientific knowledge locked behind paywalls, and thinks the cost of academic publishing has gotten out of control.
Elsevier owns around 3,000 academic journals, and its articles account for some 18 percentof all the world’s research output. “They’re a monopolist, and they act like a monopolist,” says Jeffrey MacKie-Mason, head of the campus libraries at UC Berkeley and co-chair of the team that negotiated with the publisher.Elsevier makes huge profits on its journals, generating billions of dollars a year for its parent company RELX .
This is a story about more than subscription fees. It’s about how a private industry has come to dominate the institutions of science, and how librarians, academics, and even pirates are trying to regain control.
The University of California is not the only institution fighting back. “There are thousands of Davids in this story,” says University of California Davis librarian MacKenzie Smith, who, like so many other librarians around the world, has been pushing for more open access to science. “But only a few big Goliaths.”…(More)”.
Virtuous and vicious circles in the data life-cycle
Paper by Elizabeth Yakel, Ixchel M. Faniel, and Zachary J. Maiorana: “In June 2014, ‘Data sharing reveals complexity in the westward spread of domestic animals across Neolithic Turkey’, was published in PLoS One (Arbuckle et al. 2014). In this article, twenty-three authors, all zooarchaeologists, representing seventeen different archaeological sites in Turkey investigated the domestication of animals across Neolithic southwest Asia, a pivotal era of change in the region’s economy. The PLoS One article originated in a unique data sharing, curation, and reuse project in which a majority of the authors agreed to share their data and perform analyses across the aggregated datasets. The extent of data sharing and the breadth of data reuse and collaboration were previously unprecedented in archaeology. In the present article, we conduct a case study of the collaboration leading to the development of the PLoS One article. In particular, we focus on the data sharing, data curation, and data reuse practices exercised during the project in order to investigate how different phases in the data life-cycle affected each other.
Studies of data practices have generally engaged issues from the singular perspective of data producers, sharers, curators, or reusers. Furthermore, past studies have tended to focus on one aspect of the life-cycle (production, sharing, curation, reuse, etc.). A notable exception is Carlson and Anderson’s (2007) comparative case study of four research projects which discusses the life-cycle of data from production through sharing with an eye towards reuse. However, that study primarily addresses the process of data sharing. While we see from their research that data producers’ and curators’ decisions and actions regarding data are tightly coupled and have future consequences, those consequences are not fully explicated since the authors do not discuss reuse in depth.
Taking a perspective that captures the trajectory of data, our case study discusses actions and their consequences throughout the data life-cycle. Our research theme explores how different stakeholders and their work practices positively and/or negatively affected other phases of the life-cycle. More specifically, we focus on data production practices and data selection decisions made during data sharing as these have frequent and diverse consequences for other life-cycle phases in our case study. We address the following research questions:
- How do different aspects of data production positively and negatively impact other phases in the life-cycle?
- How do data selection decisions during sharing positively and negatively impact other phases in the life-cycle?
- How can the work of data curators intervene to reinforce positive actions or mitigate negative actions?…(More)”
The Landscape of Open Data Policies
Apograf: “Open Access (OA) publishing has a long history, going back to the early 1990s, and was born with the explicit intention of improving access to scholarly literature. The internet has played a pivotal role in garnering support for free and reusable research publications, as well as stronger and more democratic peer-review systems — ones are not bogged down by the restrictions of influential publishing platforms….
Looking back, looking forward
Launched in 1991, ArXiv.org was a pioneering platform in this regard, a telling example of how researchers could cooperate to publish academic papers for free and in full view for the public. Though it has limitations — papers are curated by moderators and are not peer-reviewed — arXiv is a demonstration of how technology can be used to overcome some of the incentive and distribution problems that scientific research had long been subjected to.
The scientific community has itself assumed the mantle to this end: the Budapest Open Access Initiative (BOAI) and the Berlin Declaration on Open Access Initiative, launched in 2002 and 2003 respectively, are considered landmark movements in the push for unrestricted access to scientific research. While mostly symbolic, the effort highlighted the growing desire to solve the problems plaguing the space through technology.
The BOAI manifesto begins with a statement that is an encapsulation of the movement’s purpose,
“An old tradition and a new technology have converged to make possible an unprecedented public good. The old tradition is the willingness of scientists and scholars to publish the fruits of their research in scholarly journals without payment, for the sake of inquiry and knowledge. The new technology is the internet. The public good they make possible is the world-wide electronic distribution of the peer-reviewed journal literature and completely free and unrestricted access to it by all scientists, scholars, teachers, students, and other curious minds.”
Plan S is a more recent attempt to make publicly funded research available to all. Launched by Science Europe in September 2018, Plan S — short for ‘Shock’ — has energized the research community with its resolution to make access to publicly funded knowledge a right to everyone and dissolve the profit-driven ecosystem of research publication. Members of the European Union have vowed to achieve this by 2020.
Plan S has been supported by governments outside Europe as well. China has thrown itself behind it, and the state of California has enacted a law that requires open access to research one year after publishing. It is, of course, not without its challenges: advocacy and ensuring that publishing is not restricted a few venues are two such obstacles. However, the organization behind forming the guidelines, cOAlition S, has agreed to make the guidelines more flexible.
The emergence of this trend is not without its difficulties, however, and numerous obstacles continue to hinder the dissemination of information in a manner that is truly transparent and public. Chief among these are the many gates that continue to keep research as somewhat of exclusive property, besides the fact that the infrastructure and development for such systems are short on funding and staff…..(More)”.
The 100 Questions Initiative: Sourcing 100 questions on key societal challenges that can be answered by data insights

Press Release: “The Governance Lab at the NYU Tandon School of Engineering announced the launch of the 100 Questions Initiative — an effort to identify the most important societal questions whose answers can be found in data and data science if the power of data collaboratives is harnessed.
The initiative, launched with initial support from Schmidt Futures, seeks to address challenges on numerous topics, including migration, climate change, poverty, and the future of work.
For each of these areas and more, the initiative will seek to identify questions that could help unlock the potential of data and data science with the broader goal of fostering positive social, environmental, and economic transformation. These questions will be sourced by leveraging “bilinguals” — practitioners across disciplines from all over the world who possess both domain knowledge and data science expertise.
The 100 Questions Initiative starts by identifying 10 key questions related to migration. These include questions related to the geographies of migration, migrant well-being, enforcement and security, and the vulnerabilities of displaced people. This inaugural effort involves partnerships with the International Organization for Migration (IOM) and the European Commission, both of which will provide subject-matter expertise and facilitation support within the framework of the Big Data for Migration Alliance (BD4M).
“While there have been tremendous efforts to gather and analyze data relevant to many of the world’s most pressing challenges, as a society, we have not taken the time to ensure we’re asking the right questions to unlock the true potential of data to help address these challenges,” said Stefaan Verhulst, co-founder and chief research and development officer of The GovLab. “Unlike other efforts focused on data supply or data science expertise, this project seeks to radically improve the set of questions that, if answered, could transform the way we solve 21st century problems.”
In addition to identifying key questions, the 100 Questions Initiative will also focus on creating new data collaboratives. Data collaboratives are an emerging form of public-private partnership that help unlock the public interest value of previously siloed data. The GovLab has conducted significant research in the value of data collaboration, identifying that inter-sectoral collaboration can both increase access to information (e.g., the vast stores of data held by private companies) as well as unleash the potential of that information to serve the public good….(More)”.
Citizen, Science, and Citizen Science
Introduction by Shun-Ling and Chen Fa-ti Fan to special issue on citizen science: “The term citizen science has become very popular among scholars as well as the general public, and, given its growing presence in East Asia, it is perhaps not a moment too soon to have a special issue of EASTS on the topic. However, the quick expansion of citizen science, as a notion and a practice, has also spawned a mass of blurred meanings. The term is ill-defined and has been used in diverse ways. To avoid confusion, it is necessary to categorize the various and often ambiguous usages of the term and clarify their meanings.
As in any taxonomy, there are as many typologies as the particular perspectives, parameters, and criteria adopted for classification. There have been helpful attempts at classifying different modes of citizen science (Cooper and Lewenstein 2016; Wiggins and Crowston 2012; Haklay 2012). However, they focused primarily on the different approaches or methods in citizen science. Ottinger’s two categories of citizen science—“scientific authority driven” and “social movement based”—foreground the criteria of action and justification, but they unnecessarily juxtapose science and society; in any case, they may be too general and leaving out too much at the same time.1
In contrast, our classification will emphasize the different conceptions of citizen and citizenship in how we think about citizen science. We believe that this move can help us contextualize the ideas and practices of citizen science in the diverse socio-political conditions found in East Asia and beyond (Leach, Scoones, and Wynne 2005). To explain that point, we’ll begin with a few observations. First, the current discourse on citizen science tends to glide over such concepts as state, citizen, and the public and to assume that the reader will understand what they mean. This confidence originates in part from the fact that the default political framework of the discourse is usually Western (particularly Anglo-American). As a result, one often easily accepts a commonsense notion of participatory liberal democracy as the reference framework. However, one cannot assume that that is the de facto political framework for discussion of citizen science….(More)”.
Data Stewardship on the map: A study of tasks and roles in Dutch research institutes
Report by Verheul, Ingeborg et al: “Good research requires good data stewardship. Data stewardship encompasses all the different tasks and responsibilities that relate to caring for data during the various phases of the whole research life cycle. The basic assumption is that the researcher himself/herself is primarily responsible for all data.
However, the researcher does need professional support to achieve this. To that end, diverse supportive data stewardship roles and functions have evolved in recent years. Often they have developed over the course of time.
Their functional implementation depends largely on their place in the organization. This comes as no surprise when one considers that data stewardship consists of many facets that are traditionally assigned to different departments. Researchers regularly take on data stewardship tasks as well, not only for themselves but also in a wider context for a research group. This data stewardship work often remains unnoticed….(More)”.
The death of the literature review and the rise of the dynamic knowledge map
Gorgi Krlev at LSE Impact Blog: “Literature reviews are a core part of academic research that are loathed by some and loved by others. The LSE Impact Blog recently presented two proposals on how to deal with the issues raised by literature reviews: Richard P. Phelps argues, due to their numerous flaws, we should simply get rid of them as a requirement in scholarly articles. In contrast, Arnaud Vaganay proposes, despite their flaws, we can save them by means of standardization that would make them more robust. Here, I put forward an alternative that strikes a balance between the two: Let’s build databases that help systemize academic research. There are examples of such databases in evidence-based health-care, why not replicate those examples more widely?
The seed of the thought underlying my proposition of building dynamic knowledge maps in the social sciences and humanities was planted in 2014. I was attending a talk within Oxford’s evidence-based healthcare programme. Jon Brassey, the main speaker of the event and founder of the TRIP database, was explaining his life goal: making systematic reviews and meta-analyses in healthcare research redundant! His argument was that a database containing all available research on treatment of a symptom, migraine for instance, would be able to summarize and display meta-effects within seconds, whereas a thorough meta-analysis would require weeks, if not months, if done by a conventional research team.
Although still imperfect, TRIP has made significant progress in realizing this vision. The most recent addition to the database are “evidence maps” that visualize what we know about effective treatments. Evidence maps compare alternative treatments based on all available studies. They indicate effectiveness of a treatment, the “size” of evidence underscoring the claim and the risk of bias contained in the underlying studies. Here and below is an example based on 943 studies, as of today, dealing with effective treatment of migraine, indicating aggregated study size and risk of bias.
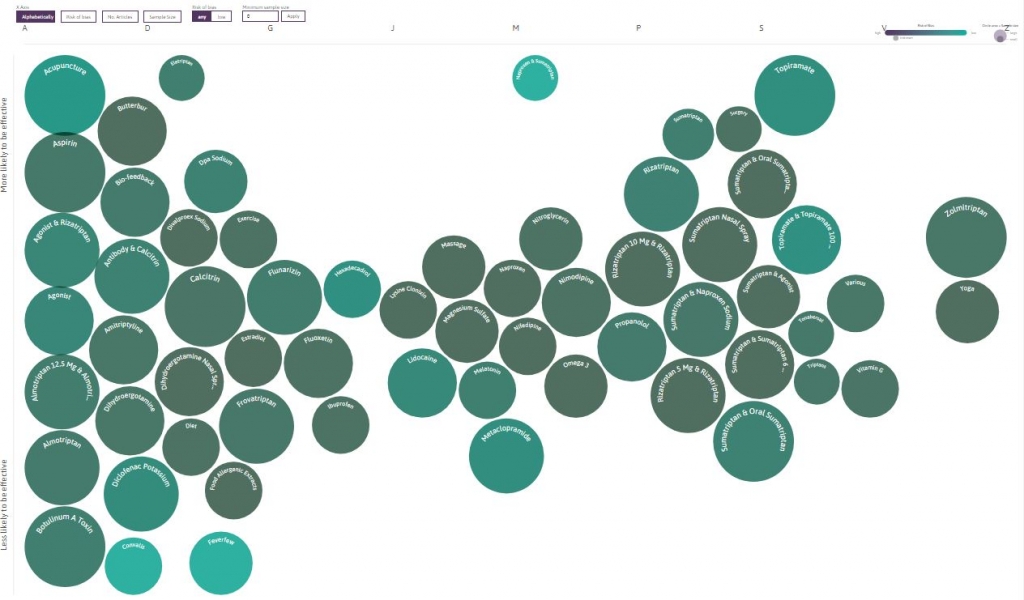
Source: TRIP database
There have been heated debates about the value and relevance of academic research (propositions have centred on intensifying research on global challenges or harnessing data for policy impact), its rigor (for example reproducibility), and the speed of knowledge production, including the “glacial pace of academic publishing”. Literature reviews, for the reasons laid out by Phelps and Vaganay, suffer from imperfections that make them: time consuming, potentially incomplete or misleading, erratic, selective, and ultimately blurry rather than insightful. As a result, conducting literature reviews is arguably not an effective use of research time and only adds to wider inefficiencies in research….(More)”.
New Report Examines Reproducibility and Replicability in Science, Recommends Ways to Improve Transparency and Rigor in Research
National Academies of Sciences: “While computational reproducibility in scientific research is generally expected when the original data and code are available, lack of ability to replicate a previous study — or obtain consistent results looking at the same scientific question but with different data — is more nuanced and occasionally can aid in the process of scientific discovery, says a new congressionally mandated report from the National Academies of Sciences, Engineering, and Medicine. Reproducibility and Replicability in Science recommends ways that researchers, academic institutions, journals, and funders should help strengthen rigor and transparency in order to improve the reproducibility and replicability of scientific research.
Defining Reproducibility and Replicability
The terms “reproducibility” and “replicability” are often used interchangeably, but the report uses each term to refer to a separate concept. Reproducibility means obtaining consistent computational results using the same input data, computational steps, methods, code, and conditions of analysis. Replicability means obtaining consistent results across studies aimed at answering the same scientific question, each of which has obtained its own data.
Reproducing research involves using the original data and code, while replicating research involves new data collection and similar methods used in previous studies, the report says. Even when a study was rigorously conducted according to best practices, correctly analyzed, and transparently reported, it may fail to be replicated.
“Being able to reproduce the computational results of another researcher starting with the same data and replicating a previous study to test its results facilitate the self-correcting nature of science, and are often cited as hallmarks of good science,” said Harvey Fineberg, president of the Gordon and Betty Moore Foundation and chair of the committee that conducted the study. “However, factors such as lack of transparency of reporting, lack of appropriate training, and methodological errors can prevent researchers from being able to reproduce or replicate a study. Research funders, journals, academic institutions, policymakers, and scientists themselves each have a role to play in improving reproducibility and replicability by ensuring that scientists adhere to the highest standards of practice, understand and express the uncertainty inherent in their conclusions, and continue to strengthen the interconnected web of scientific knowledge — the principal driver of progress in the modern world.”….(More)”.
As Surveys Falter Big Data Polling Narrows Our Societal Understanding
Kalev Leetaru at Forbes: “One of the most talked-about stories in the world of polling and survey research in recent years has been the gradual death of survey response rates and the reliability of those insights….
The online world’s perceived anonymity has offered some degree of reprieve in which online polls and surveys have often bested traditional approaches in assessing views towards society’s most controversial issues. Yet, here as well increasing public understanding of phishing and online safety are ever more problematic.
The answer has been the rise of “big data” analysis of society’s digital exhaust to fill in the gaps….
Is it truly the same answer though?
Constructing and conducting a well-designed survey means being able to ask the public exactly the questions of interest. Most importantly, it entails being able to ensure representative demographics of respondents.
An online-only poll is unlikely to accurately capture the perspectives of the three quarters of the earth’s population that the digital revolution has left behind. Even within the US, social media platforms are extraordinarily skewed.
The far greater problem is that society’s data exhaust is rarely a perfect match for the questions of greatest interest to policymakers and public.
Cellphone mobility records can offer an exquisitely detailed look at how the people of a city go about their daily lives, but beneath all that blinding light are the invisible members of society not deemed valuable to advertisers and thus not counted. Even for the urban society members whose phones are their ever-present companions, mobility data only goes so far. It can tell us that occupants of a particular part of the city during the workday spend their evenings in a particular part of the city, allowing us to understand their work/life balance, but it offers few insights into their political leanings.
One of the greatest challenges of today’s “big data” surveying is that it requires us to narrow our gaze to only those questions which can be easily answered from the data at hand.
Much as AI’s crisis of bias comes from the field’s steadfast refusal to pay for quality data, settling for highly biased free data, so too has “big data” surveying limited itself largely to datasets it can freely and easily acquire.
The result is that with traditional survey research, we are free to ask the precise questions we are most interested in. With data exhaust research, we must imperfectly shoehorn our questions into the few available metrics. With sufficient creativity it is typically possible to find some way of proxying the given question, but the resulting proxies may be highly unstable, with little understanding of when and where they may fail.
Much like how the early rise of the cluster computing era caused “big data” researchers to limit the questions they asked of their data to just those they could fit into a set of tiny machines, so too has the era of data exhaust surveying forced us to greatly restrict our understanding of society.
Most dangerously, however, big data surveying implicitly means we are measuring only the portion of society our vast commercial surveillance state cares about.
In short, we are only able to measure those deemed of greatest interest to advertisers and thus the most monetizable.
Putting this all together, the decline of traditional survey research has led to the rise of “big data” analysis of society’s data exhaust. Instead of giving us an unprecedented new view into the heartbeat of daily life, this reliance on the unintended output of our digital lives has forced researchers to greatly narrow the questions they can explore and severely skews them to the most “monetizable” portions of society.
In the end, the shift of societal understanding from precision surveys to the big data revolution has led not to an incredible new understanding of what makes us tick, but rather a far smaller, less precise and less accurate view than ever before, just our need to understand ourselves has never been greater….(More)”.