Paper by Steven Bird: “How do we roll out language technologies across a world with 7,000 languages? In one story, we scale the successes of NLP further into ‘low-resource’ languages, doing ever more with less. However, this approach does not recognise the fact that – beyond the 500 institutional languages – the remaining languages are oral vernaculars. These speech communities interact with the outside world using a ‘con-
tact language’. I argue that contact languages are the appropriate target for technologies like speech recognition and machine translation, and that the 6,500 oral vernaculars should be approached differently. I share stories from an Indigenous community where local people reshaped an extractive agenda to align with their relational agenda. I describe the emerging paradigm of Relational NLP and explain how it opens the way to non-extractive methods and to solutions that enhance human agency…(More)”
Navigating the AI Frontier: A Primer on the Evolution and Impact of AI Agents
Report by the World Economic Forum: “AI agents are autonomous systems capable of sensing, learning and acting upon their environments. This white paper explores their development and looks at how they are linked to recent advances in large language and multimodal models. It highlights how AI agents can enhance efficiency across sectors including healthcare, education and finance.
Tracing their evolution from simple rule-based programmes to sophisticated entities with complex decision-making abilities, the paper discusses both the benefits and the risks associated with AI agents. Ethical considerations such as transparency and accountability are emphasized, highlighting the need for robust governance frameworks and cross-sector collaboration.
By understanding the opportunities and challenges that AI agents present, stakeholders can responsibly leverage these systems to drive innovation, improve practices and enhance quality of life. This primer serves as a valuable resource for anyone seeking to gain a better grasp of this rapidly advancing field…(More)”.
It Was the Best of Times, It Was the Worst of Times: The Dual Realities of Data Access in the Age of Generative AI
Article by Stefaan Verhulst: “It was the best of times, it was the worst of times… It was the spring of hope, it was the winter of despair.” –Charles Dickens, A Tale of Two Cities
Charles Dickens’s famous line captures the contradictions of the present moment in the world of data. On the one hand, data has become central to addressing humanity’s most pressing challenges — climate change, healthcare, economic development, public policy, and scientific discovery. On the other hand, despite the unprecedented quantity of data being generated, significant obstacles remain to accessing and reusing it. As our digital ecosystems evolve, including the rapid advances in artificial intelligence, we find ourselves both on the verge of a golden era of open data and at risk of slipping deeper into a restrictive “data winter.”

These two realities are concurrent: the challenges posed by growing restrictions on data reuse, and the countervailing potential brought by advancements in privacy-enhancing technologies (PETs), synthetic data, and data commons approaches. It argues that while current trends toward closed data ecosystems threaten innovation, new technologies and frameworks could lead to a “Fourth Wave of Open Data,” potentially ushering in a new era of data accessibility and collaboration…(More)” (First Published in Industry Data for Society Partnership’s (IDSP) 2024 Year in Review).
The AI revolution is running out of data. What can researchers do?
Article by Nicola Jones: “The Internet is a vast ocean of human knowledge, but it isn’t infinite. And artificial intelligence (AI) researchers have nearly sucked it dry.
The past decade of explosive improvement in AI has been driven in large part by making neural networks bigger and training them on ever-more data. This scaling has proved surprisingly effective at making large language models (LLMs) — such as those that power the chatbot ChatGPT — both more capable of replicating conversational language and of developing emergent properties such as reasoning. But some specialists say that we are now approaching the limits of scaling. That’s in part because of the ballooning energy requirements for computing. But it’s also because LLM developers are running out of the conventional data sets used to train their models.
A prominent study1 made headlines this year by putting a number on this problem: researchers at Epoch AI, a virtual research institute, projected that, by around 2028, the typical size of data set used to train an AI model will reach the same size as the total estimated stock of public online text. In other words, AI is likely to run out of training data in about four years’ time (see ‘Running out of data’). At the same time, data owners — such as newspaper publishers — are starting to crack down on how their content can be used, tightening access even more. That’s causing a crisis in the size of the ‘data commons’, says Shayne Longpre, an AI researcher at the Massachusetts Institute of Technology in Cambridge who leads the Data Provenance Initiative, a grass-roots organization that conducts audits of AI data sets.
The imminent bottleneck in training data could be starting to pinch. “I strongly suspect that’s already happening,” says Longpre…(More)”
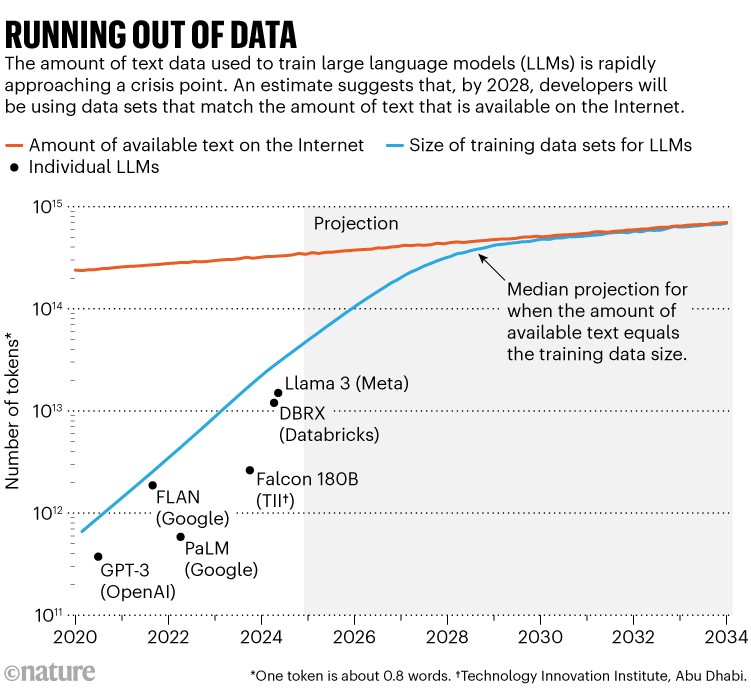
My Voice, Your Voice, Our Voice: Attitudes Towards Collective Governance of a Choral AI Dataset
Paper by Jennifer Ding, Eva Jäger, Victoria Ivanova, and Mercedes Bunz: “Data grows in value when joined and combined; likewise the power of voice grows in ensemble. With 15 UK choirs, we explore opportunities for bottom-up data governance of a jointly created Choral AI Dataset. Guided by a survey of chorister attitudes towards generative AI models trained using their data, we explore opportunities to create empowering governance structures that go beyond opt in and opt out. We test the development of novel mechanisms such as a Trusted Data Intermediary (TDI) to enable governance of the dataset amongst the choirs and AI developers. We hope our findings can contribute to growing efforts to advance collective data governance practices and shape a more creative, empowering future for arts communities in the generative AI ecosystem…(More)”.
AI could help scale humanitarian responses. But it could also have big downsides
Article by Thalia Beaty: “As the International Rescue Committee copes with dramatic increases in displaced people in recent years, the refugee aid organization has looked for efficiencies wherever it can — including using artificial intelligence.
Since 2015, the IRC has invested in Signpost — a portfolio of mobile apps and social media channels that answer questions in different languages for people in dangerous situations. The Signpost project, which includes many other organizations, has reached 18 million people so far, but IRC wants to significantly increase its reach by using AI tools — if they can do so safely.
Conflict, climate emergencies and economic hardship have driven up demand for humanitarian assistance, with more than 117 million people forcibly displaced in 2024, according to the United Nations refugee agency. The turn to artificial intelligence technologies is in part driven by the massive gap between needs and resources.
To meet its goal of reaching half of displaced people within three years, the IRC is testing a network of AI chatbots to see if they can increase the capacity of their humanitarian officers and the local organizations that directly serve people through Signpost. For now, the pilot project operates in El Salvador, Kenya, Greece and Italy and responds in 11 languages. It draws on a combination of large language models from some of the biggest technology companies, including OpenAI, Anthropic and Google.
The chatbot response system also uses customer service software from Zendesk and receives other support from Google and Cisco Systems.
If they decide the tools work, the IRC wants to extend the technical infrastructure to other nonprofit humanitarian organizations at no cost. They hope to create shared technology resources that less technically focused organizations could use without having to negotiate directly with tech companies or manage the risks of deployment…(More)”.
Digital surveillance capitalism and cities: data, democracy and activism
Paper by Ashish Makanadar: “The rapid convergence of urbanization and digital technologies is fundamentally reshaping city governance through data-driven systems. This transformation, however, is largely controlled by surveillance capitalist entities, raising profound concerns for democratic values and citizen rights. As private interests extract behavioral data from public spaces without adequate oversight, the principles of transparency and civic participation are increasingly threatened. This erosion of data sovereignty represents a critical juncture in urban development, demanding urgent interdisciplinary attention. This comment proposes a paradigm shift in urban data governance, advocating for the reclamation of data sovereignty to prioritize community interests over corporate profit motives. The paper explores socio-technical pathways to achieve this goal, focusing on grassroots approaches that assert ‘data dignity’ through privacy-enhancing technologies and digital anonymity tools. It argues for the creation of distributed digital commons as viable alternatives to proprietary data silos, thereby democratizing access to and control over urban data. The discussion extends to long-term strategies, examining the potential of blockchain technologies and decentralized autonomous organizations in enabling self-sovereign data economies. These emerging models offer a vision of ‘crypto-cities’ liberated from extractive data practices, fostering environments where residents retain autonomy over their digital footprints. By critically evaluating these approaches, the paper aims to catalyze a reimagining of smart city technologies aligned with principles of equity, shared prosperity, and citizen empowerment. This realignment is essential for preserving democratic values in an increasingly digitized urban landscape…(More)”.
Setting the Standard: Statistical Agencies’ Unique Role in Building Trustworthy AI
Article by Corinna Turbes: “As our national statistical agencies grapple with new challenges posed by artificial intelligence (AI), many agencies face intense pressure to embrace generative AI as a way to reach new audiences and demonstrate technological relevance. However, the rush to implement generative AI applications risks undermining these agencies’ fundamental role as authoritative data sources. Statistical agencies’ foundational mission—producing and disseminating high-quality, authoritative statistical information—requires a more measured approach to AI adoption.
Statistical agencies occupy a unique and vital position in our data ecosystem, entrusted with creating the reliable statistics that form the backbone of policy decisions, economic planning, and social research. The work of these agencies demands exceptional precision, transparency, and methodological rigor. Implementation of generative AI interfaces, while technologically impressive, could inadvertently compromise the very trust and accuracy that make these agencies indispensable.
While public-facing interfaces play a valuable role in democratizing access to statistical information, statistical agencies need not—and often should not—rely on generative AI to be effective in that effort. For statistical agencies, an extractive AI approach – which retrieves and presents existing information from verified databases rather than generating new content – offers a more appropriate path forward. By pulling from verified, structured datasets and providing precise, accurate responses, extractive AI systems can maintain the high standards of accuracy required while making statistical information more accessible to users who may find traditional databases overwhelming. An extractive, rather than generative, approach allows agencies to modernize data delivery while preserving their core mission of providing reliable, verifiable statistical information…(More)”
Revealed: bias found in AI system used to detect UK benefits fraud
Article by Robert Booth: “An artificial intelligence system used by the UK government to detect welfare fraud is showing bias according to people’s age, disability, marital status and nationality, the Guardian can reveal.
An internal assessment of a machine-learning programme used to vet thousands of claims for universal credit payments across England found it incorrectly selected people from some groups more than others when recommending whom to investigate for possible fraud.
The admission was made in documents released under the Freedom of Information Act by the Department for Work and Pensions (DWP). The “statistically significant outcome disparity” emerged in a “fairness analysis” of the automated system for universal credit advances carried out in February this year.
The emergence of the bias comes after the DWP this summer claimed the AI system “does not present any immediate concerns of discrimination, unfair treatment or detrimental impact on customers”.
This assurance came in part because the final decision on whether a person gets a welfare payment is still made by a human, and officials believe the continued use of the system – which is attempting to help cut an estimated £8bn a year lost in fraud and error – is “reasonable and proportionate”.
But no fairness analysis has yet been undertaken in respect of potential bias centring on race, sex, sexual orientation and religion, or pregnancy, maternity and gender reassignment status, the disclosures reveal.
Campaigners responded by accusing the government of a “hurt first, fix later” policy and called on ministers to be more open about which groups were likely to be wrongly suspected by the algorithm of trying to cheat the system…(More)”.
Predictability, AI, And Judicial Futurism: Why Robots Will Run The Law And Textualists Will Like It
Paper by Jack Kieffaber: “The question isn’t whether machines are going to replace judges and lawyers—they are. The question is whether that’s a good thing. If you’re a textualist, you have to answer yes. But you won’t—which means you’re not a textualist. Sorry.
Hypothetical: The year is 2030. AI has far eclipsed the median federal jurist as a textual interpreter. A new country is founded; it’s a democratic republic that uses human legislators to write laws and programs a state-sponsored Large Language Model called “Judge.AI” to apply those laws to facts. The model makes judicial decisions as to conduct on the back end, but can also provide advisory opinions on the front end; if a citizen types in his desired action and hits “enter,” Judge.AI will tell him, ex ante, exactly what it would decide ex post if the citizen were to perform the action and be prosecuted. The primary result is perfect predictability; secondary results include the abolition of case law, the death of common law, and the replacement of all judges—indeed, all lawyers—by a single machine. Don’t fight the hypothetical, assume it works. This article poses the question: Is that a utopia or a dystopia?
If you answer dystopia, you cannot be a textualist. Part I of this article establishes why: Because predictability is textualism’s only lodestar, and Judge.AI is substantially more predictable than any regime operating today. Part II-A dispatches rebuttals premised on positive nuances of the American system; such rebuttals forget that my hypothetical presumes a new nation and take for granted how much of our nation’s founding was premised on mitigating exactly the kinds of human error that Judge.AI would eliminate. And Part II-B dispatches normative rebuttals, which ultimately amount to moral arguments about objective good—which are none of the textualist’s business.
When the dust clears, you have only two choices: You’re a moralist, or you’re a formalist. If you’re the former, you’ll need a complete account of the objective good—which has evaded man for his entire existence. If you’re the latter, you should relish the fast-approaching day when all laws and all lawyers are usurped by a tin box. But you’re going to say you’re something in between. And you’re not…(More)”