Paper by Hannah Bloch-Wehba: “A consensus seems to be emerging that algorithmic governance is too opaque and ought to be made more accountable and transparent. But algorithmic governance underscores the limited capacity of transparency law—the Freedom of Information Act and its state equivalents—to promote accountability. Drawing on the critical literature on “open government,” this Essay shows that algorithmic governance reflects and amplifies systemic weaknesses in the transparency regime, including privatization, secrecy, private sector cooptation, and reactive disclosure. These deficiencies highlight the urgent need to reorient transparency and accountability law toward meaningful public engagement in ongoing oversight. This shift requires rethinking FOIA’s core commitment to public disclosure of agency records, exploring instead alternative ways to empower the public and to shed light on decisionmaking. The Essay argues that new approaches to transparency and accountability for algorithmic governance should be independent of private vendors, and ought to adequately represent the interests of affected individuals and communities. These considerations, of vital importance for the oversight of automated systems, also hold broader lessons for efforts to recraft open government obligations in the public interest….(More)”
Facial Recognition Technology: Federal Law Enforcement Agencies Should Better Assess Privacy and Other Risks
Report by the U.S. Government Accountability Office: “GAO surveyed 42 federal agencies that employ law enforcement officers about their use of facial recognition technology. Twenty reported owning systems with facial recognition technology or using systems owned by other entities, such as other federal, state, local, and non-government entities (see figure).
Ownership and Use of Facial Recognition Technology Reported by Federal Agencies that Employ Law Enforcement Officers
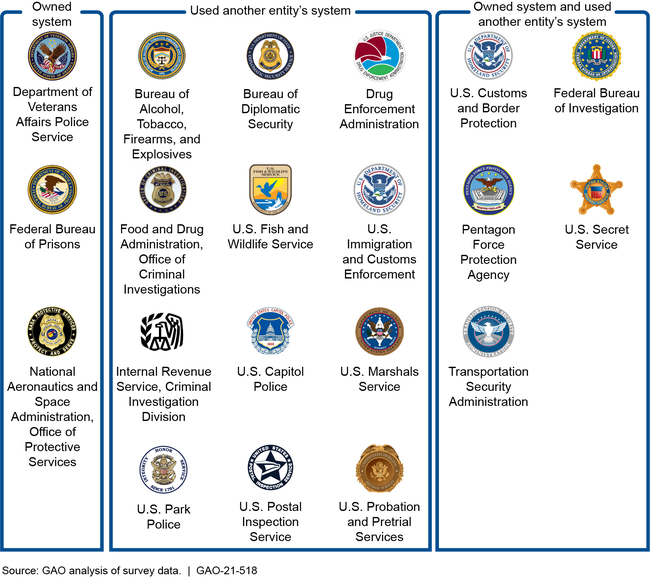
Note: For more details, see figure 2 in GAO-21-518.
Agencies reported using the technology to support several activities (e.g., criminal investigations) and in response to COVID-19 (e.g., verify an individual’s identity remotely). Six agencies reported using the technology on images of the unrest, riots, or protests following the death of George Floyd in May 2020. Three agencies reported using it on images of the events at the U.S. Capitol on January 6, 2021. Agencies said the searches used images of suspected criminal activity.
All fourteen agencies that reported using the technology to support criminal investigations also reported using systems owned by non-federal entities. However, only one has awareness of what non-federal systems are used by employees. By having a mechanism to track what non-federal systems are used by employees and assessing related risks (e.g., privacy and accuracy-related risks), agencies can better mitigate risks to themselves and the public….GAO is making two recommendations to each of 13 federal agencies to implement a mechanism to track what non-federal systems are used by employees, and assess the risks of using these systems. Twelve agencies concurred with both recommendations. U.S. Postal Service concurred with one and partially concurred with the other. GAO continues to believe the recommendation is valid, as described in the report….(More)”.
Ethics and governance of artificial intelligence for health
The WHO guidance…”on Ethics & Governance of Artificial Intelligence for Health is the product of eighteen months of deliberation amongst leading experts in ethics, digital technology, law, human rights, as well as experts from Ministries of Health. While new technologies that use artificial intelligence hold great promise to improve diagnosis, treatment, health research and drug development and to support governments carrying out public health functions, including surveillance and outbreak response, such technologies, according to the report, must put ethics and human rights at the heart of its design, deployment, and use.
The report identifies the ethical challenges and risks with the use of artificial intelligence of health, six consensus principles to ensure AI works to the public benefit of all countries. It also contains a set of recommendations that can ensure the governance of artificial intelligence for health maximizes the promise of the technology and holds all stakeholders – in the public and private sector – accountable and responsive to the healthcare workers who will rely on these technologies and the communities and individuals whose health will be affected by its use…(More)”
National strategies on Artificial Intelligence: A European perspective
Report by European Commission’s Joint Research Centre (JRC) and the OECD’s Science Technology and Innovation Directorate: “Artificial intelligence (AI) is transforming the world in many aspects. It is essential for Europe to consider how to make the most of the opportunities from this transformation and to address its challenges. In 2018 the European Commission adopted the Coordinated Plan on Artificial Intelligence that was developed together with the Member States to maximise the impact of investments at European Union (EU) and national levels, and to encourage synergies and cooperation across the EU.
One of the key actions towards these aims was an encouragement for the Member States to develop their national AI strategies.The review of national strategies is one of the tasks of AI Watch launched by the European Commission to support the implementation of the Coordinated Plan on Artificial Intelligence.
Building on the 2020 AI Watch review of national strategies, this report presents an updated review of national AI strategies from the EU Member States, Norway and Switzerland. By June 2021, 20 Member States and Norway had published national AI strategies, while 7 Member States were in the final drafting phase. Since the 2020 release of the AI Watch report, additional Member States – i.e. Bulgaria, Hungary, Poland, Slovenia, and Spain – published strategies, while Cyprus, Finland and Germany have revised the initial strategies.
This report provides an overview of national AI policies according to the following policy areas: Human capital, From the lab to the market, Networking, Regulation, and Infrastructure. These policy areas are consistent with the actions proposed in the Coordinated Plan on Artificial Intelligence and with the policy recommendations to governments contained in the OECD Recommendation on AI. The report also includes a section on AI policies to address societal challenges of the COVID-19 pandemic and climate change….(More)”.
To regulate AI, try playing in a sandbox
Article by Dan McCarthy: “For an increasing number of regulators, researchers, and tech developers, the word “sandbox” is just as likely to evoke rulemaking and compliance as it is to conjure images of children digging, playing, and building. Which is kinda the point.
That’s thanks to the rise of regulatory sandboxes, which allow organizations to develop and test new technologies in a low-stakes, monitored environment before rolling them out to the general public.
Supporters, from both the regulatory and the business sides, say sandboxes can strike the right balance of reining in potentially harmful technologies without kneecapping technological progress. They can also help regulators build technological competency and clarify how they’ll enforce laws that apply to tech. And while regulatory sandboxes originated in financial services, there’s growing interest in using them to police artificial intelligence—an urgent task as AI is expanding its reach while remaining largely unregulated.
Even for all of its promise, experts told us, the approach should be viewed not as a silver bullet for AI regulation, but instead as a potential step in the right direction.
Rashida Richardson, an AI researcher and visiting scholar at Rutgers Law School, is generally critical of AI regulatory sandboxes, but still said “it’s worth testing out ideas like this, because there is not going to be any universal model to AI regulation, and to figure out the right configuration of policy, you need to see theoretical ideas in practice.”
But waiting for the theoretical to become concrete will take time. For example, in April, the European Union proposed AI regulation that would establish regulatory sandboxes to help the EU achieve its aim of responsible AI innovation, mentioning the word “sandbox” 38 times, compared to related terms like “impact assessment” (13 mentions) and “audit” (four). But it will likely take years for the EU’s proposal to become law.
In the US, some well-known AI experts are working on an AI sandbox prototype, but regulators are not yet in the picture. However, the world’s first and (so far) only AI-specific regulatory sandbox did roll out in Norway this March, as a way to help companies comply with AI-specific provisions of the EU’s General Data Protection Regulation (GDPR). The project provides an early window into how the approach can work in practice.
“It’s a place for mutual learning—if you can learn earlier in the [product development] process, that is not only good for your compliance risk, but it’s really great for building a great product,” according to Erlend Andreas Gjære, CEO and cofounder of Secure Practice, an information security (“infosec”) startup that is one of four participants in Norway’s new AI regulatory sandbox….(More)”
How Does Artificial Intelligence Work?
BuiltIn: “Less than a decade after breaking the Nazi encryption machine Enigma and helping the Allied Forces win World War II, mathematician Alan Turing changed history a second time with a simple question: “Can machines think?”
Turing’s paper “Computing Machinery and Intelligence” (1950), and its subsequent Turing Test, established the fundamental goal and vision of artificial intelligence.
At its core, AI is the branch of computer science that aims to answer Turing’s question in the affirmative. It is the endeavor to replicate or simulate human intelligence in machines.
The expansive goal of artificial intelligence has given rise to many questions and debates. So much so, that no singular definition of the field is universally accepted.
The major limitation in defining AI as simply “building machines that are intelligent” is that it doesn’t actually explain what artificial intelligence is? What makes a machine intelligent?
In their groundbreaking textbook Artificial Intelligence: A Modern Approach, authors Stuart Russell and Peter Norvig approach the question by unifying their work around the theme of intelligent agents in machines. With this in mind, AI is “the study of agents that receive percepts from the environment and perform actions.” (Russel and Norvig viii)
Norvig and Russell go on to explore four different approaches that have historically defined the field of AI:
- Thinking humanly
- Thinking rationally
- Acting humanly
- Acting rationally
The first two ideas concern thought processes and reasoning, while the others deal with behavior. Norvig and Russell focus particularly on rational agents that act to achieve the best outcome, noting “all the skills needed for the Turing Test also allow an agent to act rationally.” (Russel and Norvig 4).
Patrick Winston, the Ford professor of artificial intelligence and computer science at MIT, defines AI as “algorithms enabled by constraints, exposed by representations that support models targeted at loops that tie thinking, perception and action together.”…(More)”.
Tasks, Automation, and the Rise in US Wage Inequality
Paper by Daron Acemoglu & Pascual Restrepo: “We document that between 50% and 70% of changes in the US wage structure over the last four decades are accounted for by the relative wage declines of worker groups specialized in routine tasks in industries experiencing rapid automation. We develop a conceptual framework where tasks across a number of industries are allocated to different types of labor and capital. Automation technologies expand the set of tasks performed by capital, displacing certain worker groups from employment opportunities for which they have comparative advantage. This framework yields a simple equation linking wage changes of a demographic group to the task displacement it experiences.
We report robust evidence in favor of this relationship and show that regression models incorporating task displacement explain much of the changes in education differentials between 1980 and 2016. Our task displacement variable captures the effects of automation technologies (and to a lesser degree offshoring) rather than those of rising market power, markups or deunionization, which themselves do not appear to play a major role in US wage inequality. We also propose a methodology for evaluating the full general equilibrium effects of task displacement (which include induced changes in industry composition and ripple effects as tasks are reallocated across different groups). Our quantitative evaluation based on this methodology explains how major changes in wage inequality can go hand-in-hand with modest productivity gains….(More)”.
NIST Proposes Method for Evaluating User Trust in Artificial Intelligence Systems
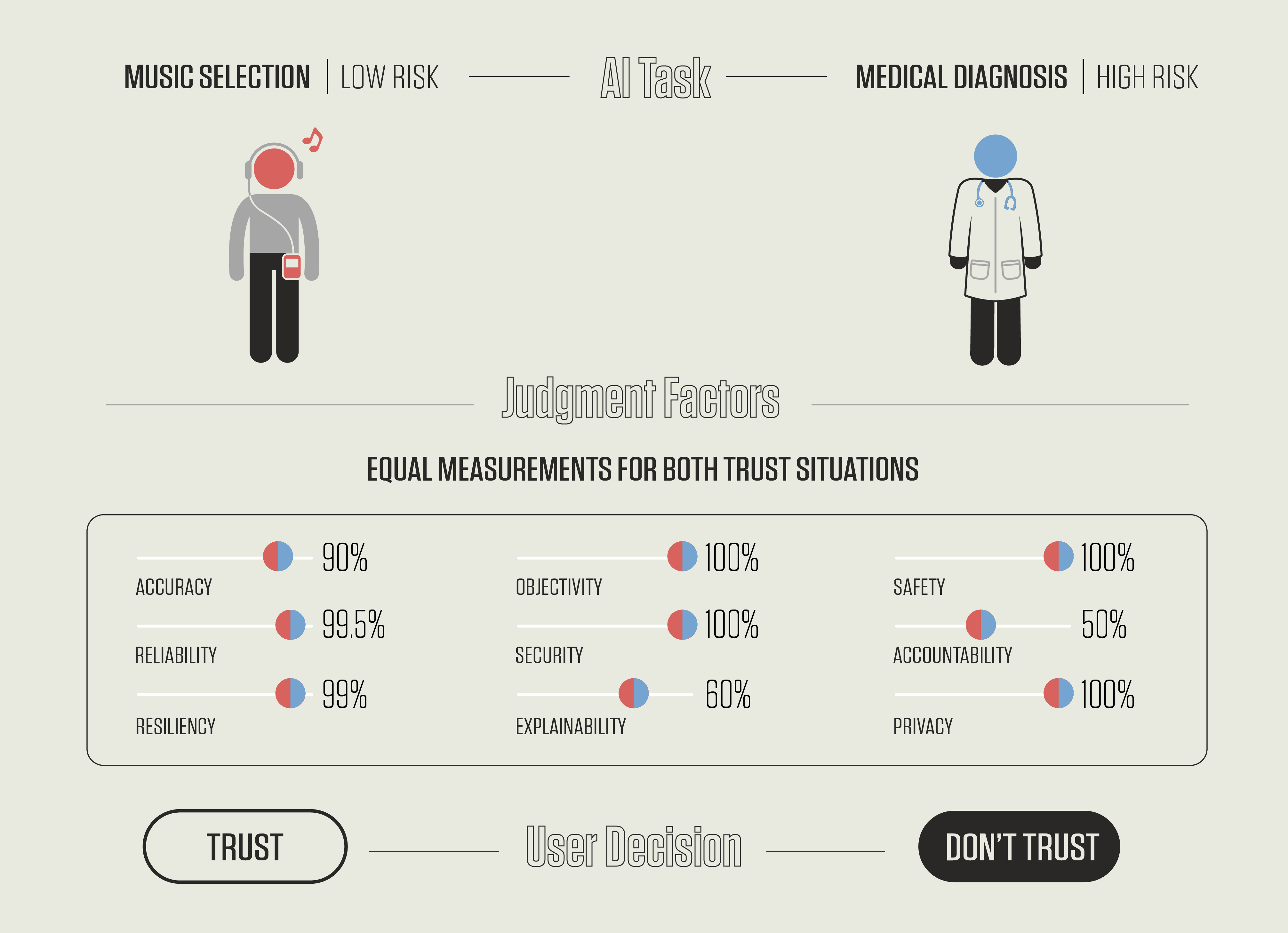
National Institute of Standards and Technology (NIST): ” Every time you speak to a virtual assistant on your smartphone, you are talking to an artificial intelligence — an AI that can, for example, learn your taste in music and make song recommendations that improve based on your interactions. However, AI also assists us with more risk-fraught activities, such as helping doctors diagnose cancer. These are two very different scenarios, but the same issue permeates both: How do we humans decide whether or not to trust a machine’s recommendations?
This is the question that a new draft publication from the National Institute of Standards and Technology (NIST) poses, with the goal of stimulating a discussion about how humans trust AI systems. The document, Artificial Intelligence and User Trust (NISTIR 8332), is open for public comment until July 30, 2021.
The report contributes to the broader NIST effort to help advance trustworthy AI systems. The focus of this latest publication is to understand how humans experience trust as they use or are affected by AI systems….(More)”.
Experts Doubt Ethical AI Design Will Be Broadly Adopted as the Norm Within the Next Decade
Report by Pew Research Center: “Artificial intelligence systems “understand” and shape a lot of what happens in people’s lives. AI applications “speak” to people and answer questions when the name of a digital voice assistant is called out. They run the chatbots that handle customer-service issues people have with companies. They help diagnose cancer and other medical conditions. They scour the use of credit cards for signs of fraud, and they determine who could be a credit risk.
They help people drive from point A to point B and update traffic information to shorten travel times. They are the operating system of driverless vehicles. They sift applications to make recommendations about job candidates. They determine the material that is offered up in people’s newsfeeds and video choices.
They recognize people’s faces, translate languages and suggest how to complete people’s sentences or search queries. They can “read” people’s emotions. They beat them at sophisticated games. They write news stories, paint in the style of Vincent Van Gogh and create music that sounds quite like the Beatles and Bach.
Corporations and governments are charging evermore expansively into AI development. Increasingly, nonprogrammers can set up off-the-shelf, pre-built AI tools as they prefer.
As this unfolds, a number of experts and advocates around the world have become worried about the long-term impact and implications of AI applications. They have concerns about how advances in AI will affect what it means to be human, to be productive and to exercise free will. Dozens of convenings and study groups have issued papers proposing what the tenets of ethical AI design should be, and government working teams have tried to address these issues. In light of this, Pew Research Center and Elon University’s Imagining the Internet Center asked experts where they thought efforts aimed at creating ethical artificial intelligence would stand in the year 2030….(More)”
Citizens ‘on mute’ in digital public service delivery
Blog by Sarah Giest at Data and Policy: “Various countries are digitalizing their welfare system in the larger context of austerity considerations and fraud detection goals, but these changes are increasingly under scrutiny. In short, digitalization of the welfare system means that with the help of mathematical models, data and/or the combination of different administrative datasets, algorithms issue a decision on, for example, an application for social benefits (Dencik and Kaun 2020).
Several examples exist where such systems have led to unfair treatment of welfare recipients. In Europe, the Dutch SyRI system has been banned by court, due to human rights violations in the profiling of welfare recipients, and the UK has found errors in the automated processes leading to financial hardship among citizens. In the United States and Canada, automated systems led to false underpayment or denial of benefits. A recent UN report (2019) even warns that countries are ‘stumbling zombie-like into a digital welfare dystopia’. Further, studies raise alarm that this process of digitalization is done in a way that it not only creates excessive information asymmetry among government and citizens, but also disadvantages certain groups more than others.
A closer look at the Dutch Childcare Allowance case highlights this. In this example, low-income parents were regarded as fraudsters by the Tax Authorities if they had incorrectly filled out any documents. An automated and algorithm-based procedure then also singled out dual-nationality families. The victims lost their allowance without having been given any reasons. Even worse, benefits already received were reclaimed. This led to individual hardship, where financial troubles and the categorization as a fraudster by government led for citizens to a chain of events from unpaid healthcare insurance and the inability to visit a doctor to job loss, potential home loss and mental health concerns (Volkskrant 2020)….(More)”.