Paper by Abdelghani Maddi and David Sapinho: “Shadow libraries, also known as ”pirate libraries”, are online collections of copyrighted publications that have been made available for free without the permission of the copyright holders. They have gradually become key players of scientific knowledge dissemination, despite their illegality in most countries of the world. Many publishers and scientist-editors decry such libraries for their copyright infringement and loss of publication usage information, while some scholars and institutions support them, sometimes in a roundabout way, for their role in reducing inequalities of access to knowledge, particularly in low-income countries. Although there is a wealth of literature on shadow libraries, none of this have focused on its potential role in knowledge dissemination, through the open access movement. Here we analyze how shadow libraries can affect researchers’ citation practices, highlighting some counter-intuitive findings about their impact on the Open Access Citation Advantage (OACA). Based on a large randomized sample, this study first shows that OA publications, including those in fully OA journals, receive more citations than their subscription-based counterparts do. However, the OACA has slightly decreased over the seven last years. The introduction of a distinction between those accessible or not via the Scihub platform among subscription-based suggest that the generalization of its use cancels the positive effect of OA publishing. The results show that publications in fully OA journals are victims of the success of Sci-hub. Thus, paradoxically, although Sci-hub may seem to facilitate access to scientific knowledge, it negatively affects the OA movement as a whole, by reducing the comparative advantage of OA publications in terms of visibility for researchers. The democratization of the use of Sci-hub may therefore lead to a vicious cycle, hindering efforts to develop full OA strategies without proposing a credible and sustainable alternative model for the dissemination of scientific knowledge…(More)”.
Open Science and Data Protection: Engaging Scientific and Legal Contexts
Editorial Paper of Special Issue edited by Ludovica Paseri: “This paper analyses the relationship between open science policies and data protection. In order to tackle the research data paradox of the contemporary science, i.e., the tension between the pursuit of data-driven scientific research and the crisis of repeatability or reproducibility of science, a theoretical perspective suggests a potential convergence between open science and data protection. Both fields regard governance mechanisms that shall take into account the plurality of interests at stake. The aim is to shed light on the processing of personal data for scientific research purposes in the context of open science. The investigation supports a threefold need: that of broadening the legal debate; of expanding the territorial scope of the analysis, in addition to the extra-territoriality effects of the European Union’s law; and an interdisciplinary discussion. Based on these needs, four perspectives are then identified, that encompass the challenges related to data processing in the context of open science: (i) the contextual and epistemological perspectives; (ii) the legal coordination perspectives; (iii) the governance perspectives; and (iv) the technical perspectives…(More)”.
Surveys Provide Insight Into Three Factors That Encourage Open Data and Science
Article by Joshua Borycz, Alison Specht and Kevin Crowston: “Open Science is a game changer for researchers and the research community. The UNESCO Open Science recommendations in 2021 suggest that the practice of Open Science is a win-win for researchers as they gain from others’ work while making contributions, which in turn benefits the community, as transparency of conclusions and hence confidence in new knowledge improves.
Over a 10-year period Carol Tenopir of DataONE and her team conducted a global survey of scientists, managers and government workers involved in broad environmental science activities about their willingness to share data and their opinion of the resources available to do so (Tenopir et al., 2011, 2015, 2018, 2020). Comparing the responses over that time shows a general increase in the willingness to share data (and thus engage in open science).
A higher willingness to share data corresponded with a decrease in satisfaction with data sharing resources across nations.
The most surprising result was that a higher willingness to share data corresponded with a decrease in satisfaction with data sharing resources across nations (e.g., skills, tools, training) (Fig.1). That is, researchers who did not want to share data were satisfied with the available resources, and those that did want to share data were dissatisfied. Researchers appear to only discover that the tools are insufficient when they begin the hard work of engaging in open science practices. This indicates that a cultural shift in the attitudes of researchers needs to precede the development of support and tools for data management…(More)”.
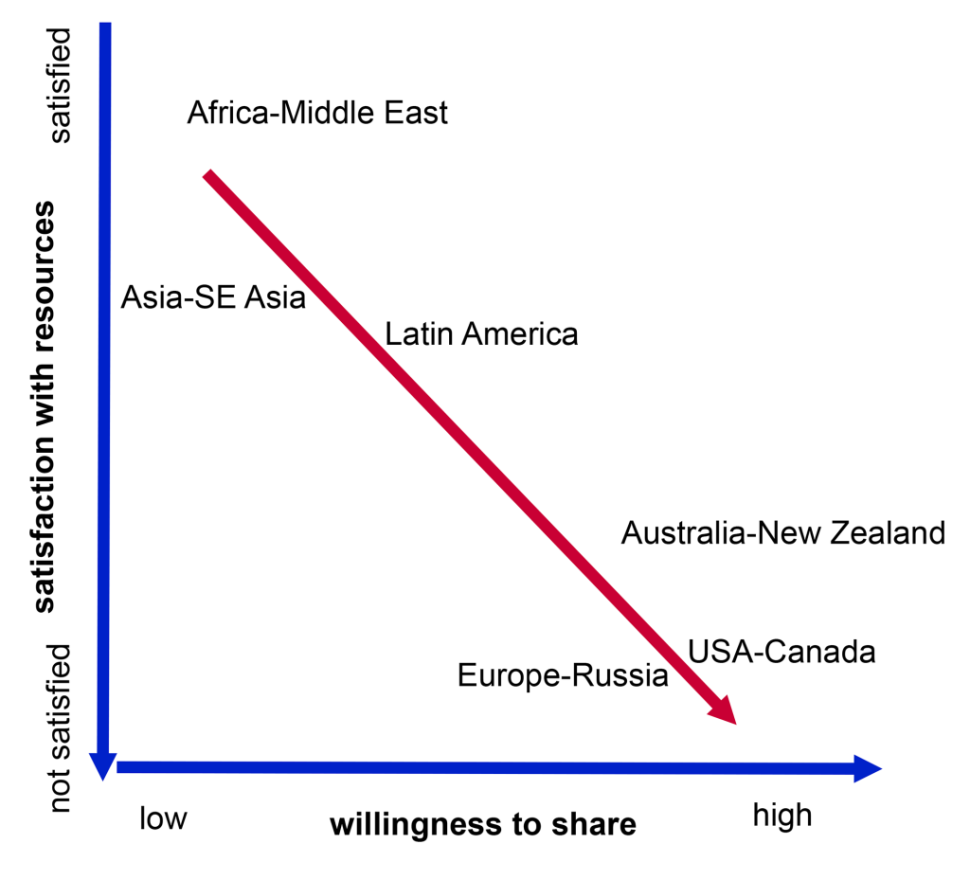
Private sector access to public sector personal data: exploring data value and benefit sharing
Literature review for the Scottish Government: “The aim of this review is to enable the Scottish Government to explore the issues relevant to the access of public sector personal data (as defined by the European Union General Data Protection Regulation, GDPR) with or by the private sector in publicly trusted ways, to unlock the public benefit of this data. This literature review will specifically enable the Scottish Government to establish whether there are
(I) models/approaches of costs/benefits/data value/benefit-sharing, and
(II) intellectual property rights or royalties schemes regarding the use of public sector personal data with or by the private sector both in the UK and internationally.
In conducting this literature review, we used an adapted systematic review, and undertook thematic analysis of the included literature to answer several questions central to the aim of this research. Such questions included:
- Are there any models of costs and/or benefits regarding the use of public sector personal data with or by the private sector?
- Are there any models of valuing data regarding the use of public sector personal data with or by the private sector?
- Are there any models for benefit-sharing in respect of the use of public sector personal data with or by the private sector?
- Are there any models in respect of the use of intellectual property rights or royalties regarding the use of public sector personal data with or by the private sector?..(More)”.
Creating public sector value through the use of open data
Summary paper prepared as part of data.europa.eu: “This summary paper provides an overview of the different stakeholder activities undertaken, ranging from surveys to a focus group, and presents the key insights from this campaign regarding data reuse practices, barriers to data reuse in the public sector and suggestions to overcome these barriers. The following recommendations are made to help data.europa.eu support public administrations to boost open data value creation.
- When it comes to raising awareness and communication, any action should also contain examples of data reuse by the public sector. Gathering and communicating such examples and use cases greatly helps in understanding the importance of the role of the public sector as a data reuser
- When it comes to policy and regulation, it would be beneficial to align the ‘better regulation’ activities and roadmaps of the European Commission with the open data publication activities, in order to better explore the internal data needs. Furthermore, it would be helpful to facilitate a similar alignment and data needs analysis for all European public administrations. For example, this could be done by providing examples, best practices and methodologies on how to map data needs for policy and regulatory purposes.
- Existing monitoring activities, such as surveys, should be revised to ensure that data reuse by the public sector is included. It would be useful to create a panel of users, based on the existing wide community, that could be used for further surveys.
- The role of data stewards remains central to favouring reuse. Therefore, examples, best practices and methodologies on the role of data stewards should be included in the support activities – not specifically for public sector reusers, but in general…(More)”.
Philosophy of Open Science
Book by Sabina Leonelli: “The Open Science [OS] movement aims to foster the wide dissemination, scrutiny and re-use of research components for the good of science and society. This Element examines the role played by OS principles and practices within contemporary research and how this relates to the epistemology of science. After reviewing some of the concerns that have prompted calls for more openness, it highlights how the interpretation of openness as the sharing of resources, so often encountered in OS initiatives and policies, may have the unwanted effect of constraining epistemic diversity and worsening epistemic injustice, resulting in unreliable and unethical scientific knowledge. By contrast, this Element proposes to frame openness as the effort to establish judicious connections among systems of practice, predicated on a process-oriented view of research as a tool for effective and responsible agency…(More)”.
Setting data free: The politics of open data for food and agriculture
Paper by M. Fairbairn, and Z. Kish: “Open data is increasingly being promoted as a route to achieve food security and agricultural development. This article critically examines the promotion of open agri-food data for development through a document-based case study of the Global Open Data for Agriculture and Nutrition (GODAN) initiative as well as through interviews with open data practitioners and participant observation at open data events. While the concept of openness is striking for its ideological flexibility, we argue that GODAN propagates an anti-political, neoliberal vision for how open data can enhance agricultural development. This approach centers values such as private innovation, increased production, efficiency, and individual empowerment, in contrast to more political and collectivist approaches to openness practiced by some agri-food social movements. We further argue that open agri-food data projects, in general, have a tendency to reproduce elements of “data colonialism,” extracting data with minimal consideration for the collective harms that may result, and embedding their own values within universalizing information infrastructures…(More)”.
Open data for AI: what now?
UNESCO Report: “…A vast amount of data on environment, industry, agriculture health about the world is now being collected through automatic processes, including sensors. Such data may be readily available, but also are potentially too big for humans to handle or analyse effectively, nonetheless they could serve as input to AI systems. AI and data science techniques have demonstrated great capacity to analyse large amounts of data, as currently illustrated by generative AI systems, and help uncover formerly unknown hidden patterns to deliver actionable information in real-time. However, many contemporary AI systems run on proprietary datasets, but data that fulfil the criteria of open data would benefit AI systems further and mitigate potential hazards of the systems such as lacking fairness, accountability, and transparency.
The aim of these guidelines is to apprise Member States of the value of open data, and to outline how data are curated and opened. Member States are encouraged not only to support openness of high-quality data, but also to embrace the use of AI technologies and facilitate capacity building, training and education in this regard, including inclusive open data as well as AI literacy…(More)”.
How data helped Mexico City reduce high-impact crime by more than 50%
Article by Alfredo Molina Ledesma: “When Claudia Sheimbaum Pardo became Mayor of Mexico City 2018, she wanted a new approach to tackling the city’s most pressing problems. Crime was at the very top of the agenda – only 7% of the city’s inhabitants considered it a safe place. New policies were needed to turn this around.
Data became a central part of the city’s new strategy. The Digital Agency for Public Innovation was created in 2019 – tasked with using data to help transform the city. To put this into action, the city administration immediately implemented an open data policy and launched their official data platform, Portal de Datos Abiertos. The policy and platform aimed to make data that Mexico City collects accessible to anyone: municipal agencies, businesses, academics, and ordinary people.
“The main objective of the open data strategy of Mexico City is to enable more people to make use of the data generated by the government in a simple and interactive manner,” said Jose Merino, Head of the Digital Agency for Public Innovation. “In other words, what we aim for is to democratize the access and use of information.” To achieve this goal a new tool for interactive data visualization called Sistema Ajolote was developed in open source and integrated into the Open Data Portal…
Information that had never been made public before, such as street-level crime from the Attorney General’s Office, is now accessible to everyone. Academics, businesses and civil society organizations can access the data to create solutions and innovations that complement the city’s new policies. One example is the successful “Hoyo de Crimen” app, which proposes safe travel routes based on the latest street-level crime data, enabling people to avoid crime hotspots as they walk or cycle through the city.
Since the introduction of the open data policy – which has contributed to a comprehensive crime reduction and social support strategy – high-impact crime in the city has decreased by 53%, and 43% of Mexico City residents now consider the city to be a safe place…(More)”.
Open Data on GitHub: Unlocking the Potential of AI
Paper by Anthony Cintron Roman, Kevin Xu, Arfon Smith, Jehu Torres Vega, Caleb Robinson, Juan M Lavista Ferres: “GitHub is the world’s largest platform for collaborative software development, with over 100 million users. GitHub is also used extensively for open data collaboration, hosting more than 800 million open data files, totaling 142 terabytes of data. This study highlights the potential of open data on GitHub and demonstrates how it can accelerate AI research. We analyze the existing landscape of open data on GitHub and the patterns of how users share datasets. Our findings show that GitHub is one of the largest hosts of open data in the world and has experienced an accelerated growth of open data assets over the past four years. By examining the open data landscape on GitHub, we aim to empower users and organizations to leverage existing open datasets and improve their discoverability — ultimately contributing to the ongoing AI revolution to help address complex societal issues. We release the three datasets that we have collected to support this analysis as open datasets at this https URL…(More)”