Paper by David Autor: “While the utopian vision of the current Information Age was that computerization would flatten economic hierarchies by democratizing information, the opposite has occurred. Information, it turns out, is merely an input into a more consequential economic function, decision-making, which is the province of elite experts. The unique opportunity that AI offers to the labor market is to extend the relevance, reach, and value of human expertise. Because of AI’s capacity to weave information and rules with acquired experience to support decision-making, it can be applied to enable a larger set of workers possessing complementary knowledge to perform some of the higher-stakes decision-making tasks that are currently arrogated to elite experts, e.g., medical care to doctors, document production to lawyers, software coding to computer engineers, and undergraduate education to professors. My thesis is not a forecast but an argument about what is possible: AI, if used well, can assist with restoring the middle-skill, middle-class heart of the US labor market that has been hollowed out by automation and globalization…(More)”.
AI cannot be used to deny health care coverage, feds clarify to insurers
Article by Beth Mole: “Health insurance companies cannot use algorithms or artificial intelligence to determine care or deny coverage to members on Medicare Advantage plans, the Centers for Medicare & Medicaid Services (CMS) clarified in a memo sent to all Medicare Advantage insurers.
The memo—formatted like an FAQ on Medicare Advantage (MA) plan rules—comes just months after patients filed lawsuits claiming that UnitedHealth and Humana have been using a deeply flawed AI-powered tool to deny care to elderly patients on MA plans. The lawsuits, which seek class-action status, center on the same AI tool, called nH Predict, used by both insurers and developed by NaviHealth, a UnitedHealth subsidiary.
According to the lawsuits, nH Predict produces draconian estimates for how long a patient will need post-acute care in facilities like skilled nursing homes and rehabilitation centers after an acute injury, illness, or event, like a fall or a stroke. And NaviHealth employees face discipline for deviating from the estimates, even though they often don’t match prescribing physicians’ recommendations or Medicare coverage rules. For instance, while MA plans typically provide up to 100 days of covered care in a nursing home after a three-day hospital stay, using nH Predict, patients on UnitedHealth’s MA plan rarely stay in nursing homes for more than 14 days before receiving payment denials, the lawsuits allege…(More)”
We urgently need data for equitable personalized medicine
Article by Manuel Corpas: “…As a bioinformatician, I am now focusing my attention on gathering the statistics to show just how biased medical research data are. There are problems across the board, ranging from which research questions get asked in the first place, to who participates in clinical trials, to who gets their genomes sequenced. The world is moving toward “precision medicine,” where any individual can have their DNA analyzed and that information can be used to help prescribe the right drugs in the right dosages. But this won’t work if a person’s genetic variants have never been identified or studied in the first place.
It’s astonishing how powerful our genetics can be in mediating medicines. Take the gene CYP2D6, which is known to play a vital role in how fast humans metabolize 25 percent of all the pharmaceuticals on the market. If you have a genetic variant of CYP2D6 that makes you metabolize drugs more quickly, or less quickly, it can have a huge impact on how well those drugs work and the dangers you face from taking them. Codeine was banned from all of Ethiopia in 2015, for example, because a high proportion of people in the country (perhaps 30 percent) have a genetic variant of CYP2D6 that makes them quickly metabolize that drug into morphine, making it more likely to cause respiratory distress and even death…(More)”
Consumer vulnerability in the digital age
OECD Report: “Protecting consumers when they are most vulnerable has long been a core focus of consumer policy. This report first discusses the nature and scale of consumer vulnerability in the digital age, including its evolving conceptualisation, the role of emerging digital trends, and implications for consumer policy. It finds that in the digital age, vulnerability may be experienced not only by some consumers, but increasingly by most, if not all, consumers. Accordingly, it sets out several measures to address the vulnerability of specific consumer groups and all consumers, and concludes with avenues for more research on the topic…(More)”.
Training Data for the Price of a Sandwich
Article by Stefan Baack: “Common Crawl (henceforth also referred to as CC) is an organization that has been essential to the technological advancements of generative AI, but is largely unknown to the broader public. This California nonprofit with only a handful of employees has crawled billions of web pages since 2008 and it makes this data available without charge via Amazon Web Services (AWS). Because of the enormous size and diversity (in terms of sources and formats) of the data, it has been pivotal as a source for training data for many AI builders. Generative AI in its current form would probably not be possible without Common Crawl, given that the vast majority of data used to train the original model behind OpenAI’s ChatGPT, the generative AI product that set off the current hype, came from it (Brown et al. 2020). The same is true for many models published since then.
Although pivotal, Common Crawl has so far received relatively little attention for its contribution to generative AI…(More)”.
Outpacing Pandemics: Solving the First and Last Mile Challenges of Data-Driven Policy Making
Article by Stefaan Verhulst, Daniela Paolotti, Ciro Cattuto, and Alessandro Vespignani: “As society continues to emerge from the legacy of COVID-19, a dangerous complacency seems to be setting in. Amidst recurrent surges of cases, each serving as a reminder of the virus’s persistence, there is a noticeable decline in collective urgency to prepare for future pandemics. This situation represents not just a lapse in memory but a significant shortfall in our approach to pandemic preparedness. It dramatically underscores the urgent need to develop novel and sustainable approaches and responses and to reinvent how we approach public health emergencies.
Among the many lessons learned from previous infectious disease outbreaks, the potential and utility of data, and particularly non-traditional forms of data, are surely among the most important lessons. Among other benefits, data has proven useful in providing intelligence and situational awareness in early stages of outbreaks, empowering citizens to protect their health and the health of vulnerable community members, advancing compliance with non-pharmaceutical interventions to mitigate societal impacts, tracking vaccination rates and the availability of treatment, and more. A variety of research now highlights the particular role played by open source data (and other non-traditional forms of data) in these initiatives.
Although multiple data sources are useful at various stages of outbreaks, we focus on two critical stages proven to be especially challenging: what we call the first mile and the last mile.
We argue that focusing on these two stages (or chokepoints) can help pandemic responses and rationalize resources. In particular, we highlight the role of Data Stewards at both stages and in overall pandemic response effectiveness…(More)”.
Data4Philanthropy
New Resource and Peer-to-Peer Learning Network: “Today’s global challenges have become increasingly complex and interconnected–from a global pandemic to the climate crisis. Solving these complex problems not only require new solutions, they also demand new methods for developing solutions and making decisions. By responsibly analyzing and using data, we can transform our understanding and approach to addressing societal issues and drive impact through our work.
However, many of these data-driven methods have not yet been adopted by the social sector or integrated across the grant-making cycle.
So we asked, how can innovations in data-driven methods and tools from multiple sectors transform decision making within philanthropy & improve the act of grant giving?
DATA4Philanthropy is a peer-to-peer learning network that aims to identify and advance the responsible use and value of data innovations across philanthropic functions.
Philanthropies can learn more about the potential of data for their sector, who to connect with to learn more about data, and how innovations in data-driven methods and tools are increasingly relevant across the stages of strategy to grant making to impact cycles.
The rapid change in both data supply, and now methods can be integrated across the philanthropy, civil society and government decision-making cycles–from developing joint priorities to improving implementation efficacy to evaluating the impact of investments…(More)”
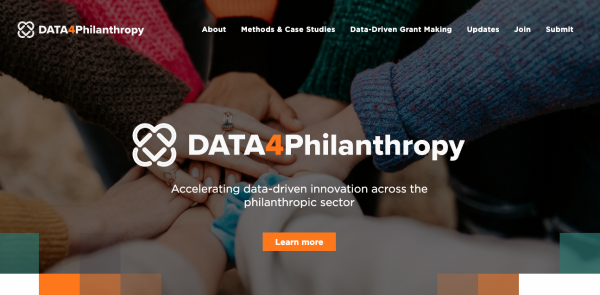
Nobody knows how to audit AI
Axios: “Some legislators and experts are pushing independent auditing of AI systems to minimize risks and build trust, Ryan reports.
Why it matters: Consumers don’t trust big tech to self-regulate and government standards may come slowly or never.
The big picture: Failure to manage risk and articulate values early in the development of an AI system can lead to problems ranging from biased outcomes from unrepresentative data to lawsuits alleging stolen intellectual property.
Driving the news: Sen. John Hickenlooper (D-Colo.) announced in a speech on Monday that he will push for the auditing of AI systems, because AI models are using our data “in ways we never imagined and certainly never consented to.”
- “We need qualified third parties to effectively audit generative AI systems,” Hickenlooper said, “We cannot rely on self-reporting alone. We should trust but verify” claims of compliance with federal laws and regulations, he said.
Catch up quick: The National Institute of Standards and Technology (NIST) developed an AI Risk Management Framework to help organizations think about and measure AI risks, but it does not certify or validate AI products.
- President Biden’s executive order on AI mandated that NIST expand its support for generative AI developers and “create guidance and benchmarks for evaluating and auditing AI capabilities,” especially in risky areas such as cybersecurity and bioweapons.
What’s happening: A growing range of companies provide services that evaluate whether AI models are complying with local regulations or promises made by their developers — but some AI companies remain committed to their own internal risk research and processes.
- NIST is only the “tip of the spear” in AI safety, Hickenlooper said. He now wants to establish criteria and a path to certification for third-party auditors.
The “Big Four” accounting firms — Deloitte, EY, KPMG and PwC — sense business opportunities in applying audit methodologies to AI systems, Nicola Morini Bianzino, EY’s global chief technology officer, tells Axios.
- Morini Bianzino cautions that AI audits might “look more like risk management for a financial institution, as opposed to audit as a certifying mark. Because, honestly, I don’t know technically how we would do that.”
- Laura Newinski, KPMG’s COO, tells Axios the firm is developing AI auditing services and “attestation about whether data sets are accurate and follow certain standards.”
Established players such as IBM and startups such as Credo provide AI governance dashboards that tell clients in real time where AI models could be causing problems — around data privacy, for example.
- Anthropic believes NIST should focus on “building a robust and standardized benchmark for generative AI systems” that all private AI companies can adhere to.
Market leader OpenAI announced in October that it’s creating a “risk-informed development policy” and has invited experts to apply to join its OpenAI Red Teaming Network.
- OpenAI also released a paper Jan. 31 purporting to examine whether its models increase the risk of bioweapons. The company’s answer: not really.
- NYU professor Gary Marcus argues the paper is misleading. “The more I look at the results, the more worried I become,” Marcus wrote in his blog. “Company white papers are not peer-reviewed articles,” he notes.
Yes, but: An AI audit industry without clear standards could be a recipe for confusion, both for corporate customers and consumers using AI…(More)”.
Data Is What Data Does: Regulating Based on Harm and Risk Instead of Sensitive Data
Paper by Daniel J. Solove: “Heightened protection for sensitive data is becoming quite trendy in privacy laws around the world. Originating in European Union (EU) data protection law and included in the EU’s General Data Protection Regulation, sensitive data singles out certain categories of personal data for extra protection. Commonly recognized special categories of sensitive data include racial or ethnic origin, political opinions, religious or philosophical beliefs, trade union membership, health, sexual orientation and sex life, and biometric and genetic data.
Although heightened protection for sensitive data appropriately recognizes that not all situations involving personal data should be protected uniformly, the sensitive data approach is a dead end. The sensitive data categories are arbitrary and lack any coherent theory for identifying them. The borderlines of many categories are so blurry that they are useless. Moreover, it is easy to use nonsensitive data as a proxy for certain types of sensitive data.
Personal data is akin to a grand tapestry, with different types of data interwoven to a degree that makes it impossible to separate out the strands. With Big Data and powerful machine learning algorithms, most nonsensitive data give rise to inferences about sensitive data. In many privacy laws, data giving rise to inferences about sensitive data is also protected as sensitive data. Arguably, then, nearly all personal data can be sensitive, and the sensitive data categories can swallow up everything. As a result, most organizations are currently processing a vast amount of data in violation of the laws.
This Article argues that the problems with the sensitive data approach make it unworkable and counterproductive as well as expose a deeper flaw at the root of many privacy laws. These laws make a fundamental conceptual mistake—they embrace the idea that the nature of personal data is a sufficiently useful focal point for the law. But nothing meaningful for regulation can be determined solely by looking at the data itself. Data is what data does.
To be effective, privacy law must focus on harm and risk rather than on the nature of personal data…(More)”.
The story of the R number: How an obscure epidemiological figure took over our lives
Article by Gavin Freeguard: “Covid-19 did not only dominate our lives in April 2020. It also dominated the list of new words entered into the Oxford English Dictionary.
Alongside Covid-19 itself (noun, “An acute respiratory illness in humans caused by a coronavirus”), the vocabulary of the virus included “self-quarantine”, “social distancing”, “infodemic”, “flatten the curve”, “personal protective equipment”, “elbow bump”, “WFH” and much else. But nestled among this pantheon of new pandemic words was a number, one that would shape our conversations, our politics, our lives for the next 18 months like no other: “Basic reproduction number (R0): The average number of cases of an infectious disease arising by transmission from a single infected individual, in a population that has not previously encountered the disease.”

“There have been many important figures in this pandemic,” wrote The Times in January 2021, “but one has come to tower over the rest: the reproduction rate. The R number, as everyone calls it, has been used by the government to justify imposing and lifting lockdowns. Indeed while there are many important numbers — gross domestic product, parliamentary majorities, interest rates — few can compete right now with R” (tinyurl.com/v7j6cth9).
Descriptions of it at the start of the pandemic made R the star of the disaster movie reality we lived through. And it wasn’t just a breakout star of the UK’s coronavirus press conferences; in Germany, (then) Chancellor Angela Merkel made the most of her scientific background to explain the meaning of R and its consequences to the public (tinyurl.com/mva7urw5).
But for others, the “obsession” (Professor Linda Bauld, University of Edinburgh) with “the pandemic’s misunderstood metric” (Nature: tinyurl.com/y3sr6n6m) has been “a distraction”, an “unhelpful focus”; as the University of Edinburgh’s Professor Mark Woolhouse told one parliamentary select committee, “we’ve created a monster”.
How did this epidemiological number come to dominate our discourse? How useful is it? And where does it come from?…(More)”.