Blog by Paul Atherton and Alasdair Mackintosh:”Sierra Leone has made significant progress towards educational targets in recent years, but is still struggling to ensure equitable access to quality teachers for all its learners. The government is exploring innovative solutions to tackle this problem. In support of this, Fab Inc. has brought their expertise in data science and education systems, merging the two to use spatial analysis to unpack and explore this challenge….
Figure 1: Pupil-teacher ratio for primary education by district (left); and within Kailahun district, Sierra Leone, by chiefdom (right), 2020.
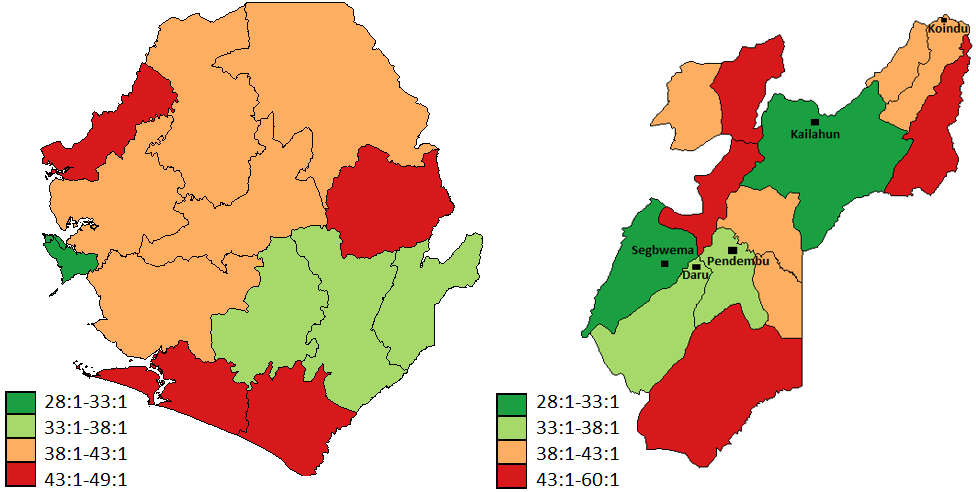
Source: Mackintosh, A., A. Ramirez, P. Atherton, V. Collis, M. Mason-Sesay, & C. Bart-Williams. 2019. Education Workforce Spatial Analysis in Sierra Leone. Research and Policy Paper. Education Workforce Initiative. The Education Commission.
…Spatial analysis, also referred to as geospatial analysis, is a set of techniques to explain patterns and behaviours in terms of geography and locations. It uses geographical features, such as distances, travel times and school neighbourhoods, to identify relationships and patterns.
Our team, using its expertise in both data science and education systems, examined issues linked to remoteness to produce a clearer picture of Sierra Leone’s teacher shortage. To see how the current education workforce was distributed across the country, and how well it served local populations, we drew on geo-processed population data from the Grid-3 initiative and the Government of Sierra Leone’s Education Data Hub. The project benefited from close collaboration with the Ministry and Teaching Service Commission (TSC).
Our analysis focused on teacher development, training and the deployment of new teachers across regions, drawing on exam data. Surveys of teacher training colleges (TTCs) were conducted to assess how many future teachers will need to be trained to make up for shortages. Gender and subject speciality were analysed to better address local imbalances. The team developed a matching algorithm for teacher deployment, to illustrate how schools’ needs, including aspects of qualifications and subject specialisms, can be matched to teachers’ preferences, including aspects of language and family connections, to improve allocation of both current and future teachers….(More)”