Courtney Linder at Popular Mechanics: “Several prominent academic mathematicians want to sever ties with police departments across the U.S., according to a letter submitted to Notices of the American Mathematical Society on June 15. The letter arrived weeks after widespread protests against police brutality, and has inspired over 1,500 other researchers to join the boycott.
These mathematicians are urging fellow researchers to stop all work related to predictive policing software, which broadly includes any data analytics tools that use historical data to help forecast future crime, potential offenders, and victims. The technology is supposed to use probability to help police departments tailor their neighborhood coverage so it puts officers in the right place at the right time….
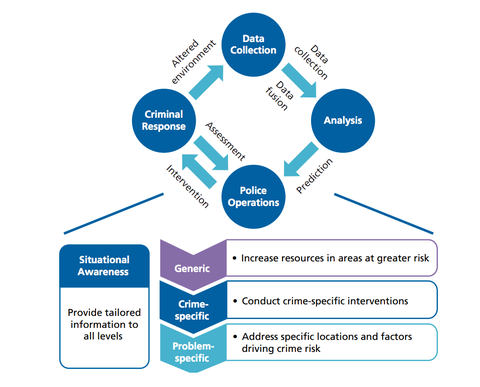
RAND
According to a 2013 research briefing from the RAND Corporation, a nonprofit think tank in Santa Monica, California, predictive policing is made up of a four-part cycle (shown above). In the first two steps, researchers collect and analyze data on crimes, incidents, and offenders to come up with predictions. From there, police intervene based on the predictions, usually taking the form of an increase in resources at certain sites at certain times. The fourth step is, ideally, reducing crime.
“Law enforcement agencies should assess the immediate effects of the intervention to ensure that there are no immediately visible problems,” the authors note. “Agencies should also track longer-term changes by examining collected data, performing additional analysis, and modifying operations as needed.”
In many cases, predictive policing software was meant to be a tool to augment police departments that are facing budget crises with less officers to cover a region. If cops can target certain geographical areas at certain times, then they can get ahead of the 911 calls and maybe even reduce the rate of crime.
But in practice, the accuracy of the technology has been contested—and it’s even been called racist….(More)”.