Blogpost by Laura McGorman and Alex Pompe at Facebook: “Small businesses and people around the world are suffering devastating financial losses due to the ongoing COVID-19 pandemic, and public institutions need real time information to help. Today Facebook is launching new datasets and insights to help support economic recovery through our Data for Good program.
Researchers estimate that over the next five years, the global economy could suffer over $80 trillion in losses due to COVID-19. Small businesses in particular are being hit hard — our Global State of Small Business Report found that over one in four had closed their doors in 2020. Governments around the world are looking to effectively distribute financial aid as well as accurately forecast when and how economies will recover. These four datasets — Business Activity Trends, Commuting Zones, Economic Insights from the Symptom Survey and the latest Future of Business Survey results — will help researchers, nonprofits and local officials identify which areas and businesses may need the most support.
Business Activity Trends
Many factors influence the pandemic’s impact on local economies around the world. However, real time information on business activity is scarce, leaving institutions seeking to provide economic aid with limited information on how to distribute it. To address these information gaps, we partnered with the University of Bristol to aggregate information from Facebook Business Pages to estimate the change in activity among local businesses around the world and how they respond and recover from crises over time.
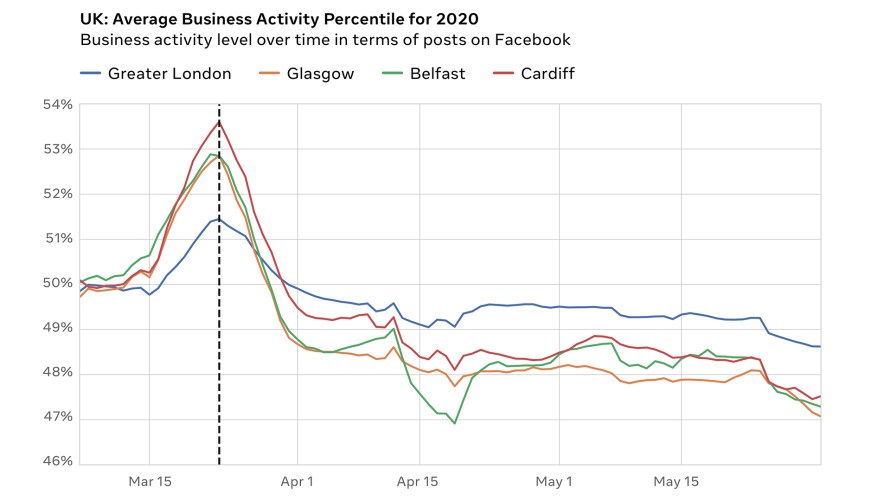
“Determining whether small and medium businesses are open is very important to assess the recovery after events like mandatory stay-at-home orders,” said Dr. Flavia De Luca, Senior Lecturer in Structural and Earthquake Engineering at the University of Bristol. “The traditional way of collecting this information, such as surveys and interviews, are usually costly, time consuming, and do not scale. By using real time information from Facebook, we hope to make it easier for public institutions to better respond to these events.”…(More)”.