Report by Paris21: “Artificial Intelligence (AI) and its impact on people’s lives is growing rapidly. AI is already leading to significant developments from healthcare to education, which can contribute to the efficient monitoring and achievement of the Sustainable Development Goals (SDGs), a call to action to address the world’s greatest challenges. AI is also raising concerns because, if not addressed carefully, its risks may outweigh its benefits. As a result, AI is garnering increasing attention from National Statistical Offices (NSOs) and the official statistics community as they are challenged to produce more, comprehensive, timely, and highquality data for decision-making with limited resources in a rapidly changing world of data and technologies and in light of complex and converging global issues from pandemics to climate change. This paper has been prepared as an input to the “Data and AI for Sustainable Development: Building a Smarter Future” Conference, organized in partnership with The Partnership in Statistics for Development in the 21st Century (PARIS21), the World Bank and the International Monetary Fund (IMF). Building on case studies that examine the use of AI by NSOs, the paper presents the benefits and risks of AI with a focus on NSO operations related to sustainable development. The objective is to spark discussions and to initiate a dialogue around how AI can be leveraged to inform decisions and take action to better monitor and achieve sustainable development, while mitigating its risks…(More)”.
Generative AI in Journalism
Report by Nicholas Diakopoulos et al: “The introduction of ChatGPT by OpenAI in late 2022 captured the imagination of the public—and the news industry—with the potential of generative AI to upend how people create and consume media. Generative AI is a type of artificial intelligence technology that can create new content, such as text, images, audio, video, or other media, based on the data it has been trained on and according to written prompts provided by users. ChatGPT is the chat-based user interface that made the power and potential of generative AI salient to a wide audience, reaching 100 million users within two months of its launch.
Although similar technology had been around, by late 2022 it was suddenly working, spurring its integration into various products and presenting not only a host of opportunities for productivity and new experiences but also some serious concerns about accuracy, provenance and attribution of source information, and the increased potential for creating misinformation.
This report serves as a snapshot of how the news industry has grappled with the initial promises and challenges of generative AI towards the end of 2023. The sample of participants reflects how some of the more savvy and experienced members of the profession are reacting to the technology.
Based on participants’ responses, they found that generative AI is already changing work structure and organization, even as it triggers ethical concerns around use. Here are some key takeaways:
- Applications in News Production. The most predominant current use cases for generative AI include various forms of textual content production, information gathering and sensemaking, multimedia content production, and business uses.
- Changing Work Structure and Organization. There are a host of new roles emerging to grapple with the changes introduced by generative AI including for leadership, editorial, product, legal, and engineering positions.
- Work Redesign. There is an unmet opportunity to design new interfaces to support journalistic work with generative AI, in particular to enable the human oversight needed for the efficient and confident checking and verification of outputs..(More)”
Data Authenticity, Consent, and Provenance for AI Are All Broken: What Will It Take to Fix Them?
Article by Shayne Longpre et al: “New AI capabilities are owed in large part to massive, widely sourced, and underdocumented training data collections. Dubious collection practices have spurred crises in data transparency, authenticity, consent, privacy, representation, bias, copyright infringement, and the overall development of ethical and trustworthy AI systems. In response, AI regulation is emphasizing the need for training data transparency to understand AI model limitations. Based on a large-scale analysis of the AI training data landscape and existing solutions, we identify the missing infrastructure to facilitate responsible AI development practices. We explain why existing tools for data authenticity, consent, and documentation alone are unable to solve the core problems facing the AI community, and outline how policymakers, developers, and data creators can facilitate responsible AI development, through universal data provenance standards…(More)”.
AI and the Future of Government: Unexpected Effects and Critical Challenges
Policy Brief by Tiago C. Peixoto, Otaviano Canuto, and Luke Jordan: “Based on observable facts, this policy paper explores some of the less- acknowledged yet critically important ways in which artificial intelligence (AI) may affect the public sector and its role. Our focus is on those areas where AI’s influence might be understated currently, but where it has substantial implications for future government policies and actions.
We identify four main areas of impact that could redefine the public sector role, require new answers from it, or both. These areas are the emergence of a new language-based digital divide, jobs displacement in the public administration, disruptions in revenue mobilization, and declining government responsiveness.
This discussion not only identifies critical areas but also underscores the importance of transcending conventional approaches in tackling them. As we examine these challenges, we shed light on their significance, seeking to inform policymakers and stakeholders about the nuanced ways in which AI may quietly, yet profoundly, alter the public sector landscape…(More)”.
AI for Good: Applications in Sustainability, Humanitarian Action, and Health
Book by Juan M. Lavista Ferres and William B. Weeks: “…an insightful and fascinating discussion of how one of the world’s most recognizable software companies is tacking intractable social problems with the power of artificial intelligence (AI). In the book, you’ll learn about how climate change, illness and disease, and challenges to fundamental human rights are all being fought using replicable methods and reusable AI code.
The authors also provide:
- Easy-to-follow, non-technical explanations of what AI is and how it works
- Examinations of how healthcare is being improved, climate change is being addressed, and humanitarian aid is being facilitated around the world with AI
- Discussions of the future of AI in the realm of social benefit organizations and efforts
An essential guide to impactful social change with artificial intelligence, AI for Good is a must-read resource for technical and non-technical professionals interested in AI’s social potential, as well as policymakers, regulators, NGO professionals, and, and non-profit volunteers…(More)”.
The Cambridge Handbook of Facial Recognition in the Modern State
Book edited by Rita Matulionyte and Monika Zalnieriute: “In situations ranging from border control to policing and welfare, governments are using automated facial recognition technology (FRT) to collect taxes, prevent crime, police cities and control immigration. FRT involves the processing of a person’s facial image, usually for identification, categorisation or counting. This ambitious handbook brings together a diverse group of legal, computer, communications, and social and political science scholars to shed light on how FRT has been developed, used by public authorities, and regulated in different jurisdictions across five continents. Informed by their experiences working on FRT across the globe, chapter authors analyse the increasing deployment of FRT in public and private life. The collection argues for the passage of new laws, rules, frameworks, and approaches to prevent harms of FRT in the modern state and advances the debate on scrutiny of power and accountability of public authorities which use FRT…(More)”.
AI Accountability Policy Report
Report by NTIA: “Artificial intelligence (AI) systems are rapidly becoming part of the fabric of everyday American life. From customer service to image generation to manufacturing, AI systems are everywhere.
Alongside their transformative potential for good, AI systems also pose risks of harm. These risks include inaccurate or false outputs; unlawful discriminatory algorithmic decision making; destruction of jobs and the dignity of work; and compromised privacy, safety, and security. Given their influence and ubiquity, these systems must be subject to security and operational mechanisms that mitigate risk and warrant stakeholder trust that they will not cause harm….
The AI Accountability Policy Report conceives of accountability as a chain of inputs linked to consequences. It focuses on how information flow (documentation, disclosures, and access) supports independent evaluations (including red-teaming and audits), which in turn feed into consequences (including liability and regulation) to create accountability. It concludes with recommendations for federal government action, some of which elaborate on themes in the AI EO, to encourage and possibly require accountability inputs…(More)”.
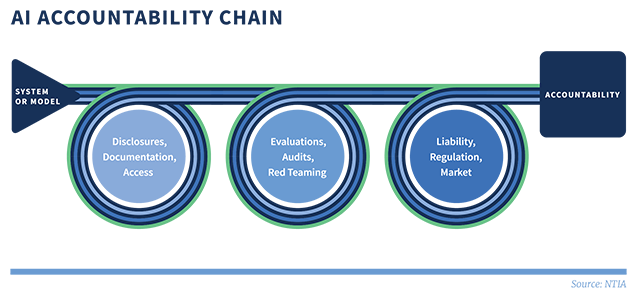
A.I.-Generated Garbage Is Polluting Our Culture
Article by Eric Hoel: “Increasingly, mounds of synthetic A.I.-generated outputs drift across our feeds and our searches. The stakes go far beyond what’s on our screens. The entire culture is becoming affected by A.I.’s runoff, an insidious creep into our most important institutions.
Consider science. Right after the blockbuster release of GPT-4, the latest artificial intelligence model from OpenAI and one of the most advanced in existence, the language of scientific research began to mutate. Especially within the field of A.I. itself.
A study published this month examined scientists’ peer reviews — researchers’ official pronouncements on others’ work that form the bedrock of scientific progress — across a number of high-profile and prestigious scientific conferences studying A.I. At one such conference, those peer reviews used the word “meticulous” more than 34 times as often as reviews did the previous year. Use of “commendable” was around 10 times as frequent, and “intricate,” 11 times. Other major conferences showed similar patterns.
Such phrasings are, of course, some of the favorite buzzwords of modern large language models like ChatGPT. In other words, significant numbers of researchers at A.I. conferences were caught handing their peer review of others’ work over to A.I. — or, at minimum, writing them with lots of A.I. assistance. And the closer to the deadline the submitted reviews were received, the more A.I. usage was found in them.
If this makes you uncomfortable — especially given A.I.’s current unreliability — or if you think that maybe it shouldn’t be A.I.s reviewing science but the scientists themselves, those feelings highlight the paradox at the core of this technology: It’s unclear what the ethical line is between scam and regular usage. Some A.I.-generated scams are easy to identify, like the medical journal paper featuring a cartoon rat sporting enormous genitalia. Many others are more insidious, like the mislabeled and hallucinated regulatory pathway described in that same paper — a paper that was peer reviewed as well (perhaps, one might speculate, by another A.I.?)…(More)”.
AI Is Building Highly Effective Antibodies That Humans Can’t Even Imagine
Article by Amit Katwala: “Robots, computers, and algorithms are hunting for potential new therapies in ways humans can’t—by processing huge volumes of data and building previously unimagined molecules. At an old biscuit factory in South London, giant mixers and industrial ovens have been replaced by robotic arms, incubators, and DNA sequencing machines.
James Field and his company LabGenius aren’t making sweet treats; they’re cooking up a revolutionary, AI-powered approach to engineering new medical antibodies. In nature, antibodies are the body’s response to disease and serve as the immune system’s front-line troops. They’re strands of protein that are specially shaped to stick to foreign invaders so that they can be flushed from the system. Since the 1980s, pharmaceutical companies have been making synthetic antibodies to treat diseases like cancer, and to reduce the chance of transplanted organs being rejected. But designing these antibodies is a slow process for humans—protein designers must wade through the millions of potential combinations of amino acids to find the ones that will fold together in exactly the right way, and then test them all experimentally, tweaking some variables to improve some characteristics of the treatment while hoping that doesn’t make it worse in other ways. “If you want to create a new therapeutic antibody, somewhere in this infinite space of potential molecules sits the molecule you want to find,” says Field, the founder and CEO of LabGenius…(More)”.
Market Power in Artificial Intelligence
Paper by Joshua S. Gans: “This paper surveys the relevant existing literature that can help researchers and policy makers understand the drivers of competition in markets that constitute the provision of artificial intelligence products. The focus is on three broad markets: training data, input data, and AI predictions. It is shown that a key factor in determining the emergence and persistence of market power will be the operation of markets for data that would allow for trading data across firm boundaries…(More)”.