Article by Henrietta Howells: “Raw neuroimaging data require further processing before they can be used for scientific or clinical research. Traditionally, this could be accomplished with a single powerful computer. However, much greater computing power is required to analyze the large open-access cohorts that are increasingly being released to the community. And processing pipelines are inconsistently scripted, which can hinder reproducibility efforts. This creates a barrier for labs lacking access to sufficient resources or technological support, potentially excluding them from neuroimaging research. A paper by Hayashi and colleagues in Nature Methods offers a solution. They present https://brainlife.io, a freely available, web-based platform for secure neuroimaging data access, processing, visualization and analysis. It leverages ‘opportunistic computing’, which pools processing power from commercial and academic clouds, making it accessible to scientists worldwide. This is a step towards lowering the barriers for entry into big data neuroimaging research…(More)”.
Global Digital Data Governance: Polycentric Perspectives
(Open Access) Book edited by Carolina Aguerre, Malcolm Campbell-Verduyn, and Jan Aart Scholte: “This book provides a nuanced exploration of contemporary digital data governance, highlighting the importance of cooperation across sectors and disciplines in order to adapt to a rapidly evolving technological landscape. Most of the theory around global digital data governance remains scattered and focused on specific actors, norms, processes, or disciplinary approaches. This book argues for a polycentric approach, allowing readers to consider the issue across multiple disciplines and scales.
Polycentrism, this book argues, provides a set of lenses that tie together the variety of actors, issues, and processes intertwined in digital data governance at subnational, national, regional, and global levels. Firstly, this approach uncovers the complex array of power centers and connections in digital data governance. Secondly, polycentric perspectives bridge disciplinary divides, challenging assumptions and drawing together a growing range of insights about the complexities of digital data governance. Bringing together a wide range of case studies, this book draws out key insights and policy recommendations for how digital data governance occurs and how it might occur differently…(More)”.
Google’s Expanded ‘Flood Hub’ Uses AI to Help Us Adapt to Extreme Weather
Article by Jeff Young: “Google announced Tuesday that a tool using artificial intelligence to better predict river floods will be expanded to the U.S. and Canada, covering more than 800 North American riverside communities that are home to more than 12 million people. Google calls it Flood Hub, and it’s the latest example of how AI is being used to help adapt to extreme weather events associated with climate change.
“We see tremendous opportunity for AI to solve some of the world’s biggest challenges, and climate change is very much one of those,” Google’s Chief Sustainability Officer, Kate Brandt, told Newsweek in an interview.
At an event in Brussels on Tuesday, Google announced a suite of new and expanded sustainability initiatives and products. Many of them involve the use of AI, such as tools to help city planners find the best places to plant trees and modify rooftops to buffer against city heat, and a partnership with the U.S. Forest Service to use AI to improve maps related to wildfires.
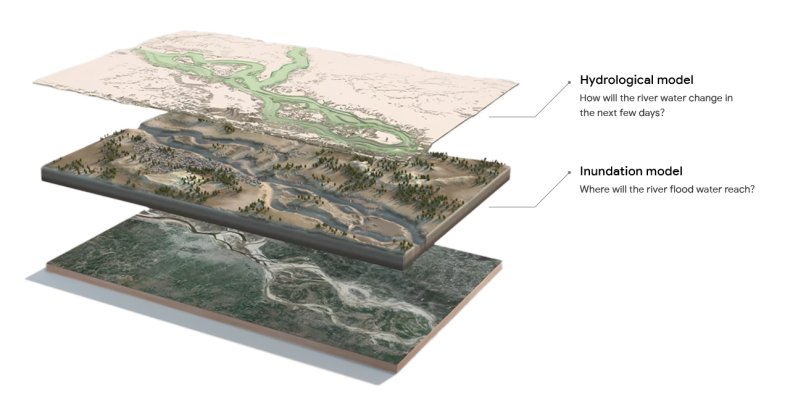
Brandt said Flood Hub’s engineers use advanced AI, publicly available data sources and satellite imagery, combined with hydrologic models of river flows. The results allow flooding predictions with a longer lead time than was previously available in many instances…(More)”.
The Age of Prediction: Algorithms, AI, and the Shifting Shadows of Risk
Book by Igor Tulchinsky and Christopher E. Mason: “… about two powerful, and symbiotic, trends: the rapid development and use of artificial intelligence and big data to enhance prediction, as well as the often paradoxical effects of these better predictions on our understanding of risk and the ways we live. Beginning with dramatic advances in quantitative investing and precision medicine, this book explores how predictive technology is quietly reshaping our world in fundamental ways, from crime fighting and warfare to monitoring individual health and elections.
As prediction grows more robust, it also alters the nature of the accompanying risk, setting up unintended and unexpected consequences. The Age of Prediction details how predictive certainties can bring about complacency or even an increase in risks—genomic analysis might lead to unhealthier lifestyles or a GPS might encourage less attentive driving. With greater predictability also comes a degree of mystery, and the authors ask how narrower risks might affect markets, insurance, or risk tolerance generally. Can we ever reduce risk to zero? Should we even try? This book lays an intriguing groundwork for answering these fundamental questions and maps out the latest tools and technologies that power these projections into the future, sometimes using novel, cross-disciplinary tools to map out cancer growth, people’s medical risks, and stock dynamics…(More)”.
Ethical Considerations Towards Protestware
Paper by Marc Cheong, Raula Gaikovina Kula, and Christoph Treude: “A key drawback to using a Open Source third-party library is the risk of introducing malicious attacks. In recently times, these threats have taken a new form, when maintainers turn their Open Source libraries into protestware. This is defined as software containing political messages delivered through these libraries, which can either be malicious or benign. Since developers are willing to freely open-up their software to these libraries, much trust and responsibility are placed on the maintainers to ensure that the library does what it promises to do. This paper takes a look into the possible scenarios where developers might consider turning their Open Source Software into protestware, using an ethico-philosophical lens. Using different frameworks commonly used in AI ethics, we explore the different dilemmas that may result in protestware. Additionally, we illustrate how an open-source maintainer’s decision to protest is influenced by different stakeholders (viz., their membership in the OSS community, their personal views, financial motivations, social status, and moral viewpoints), making protestware a multifaceted and intricate matter…(More)”
From LogFrames to Logarithms – A Travel Log
Article by Karl Steinacker and Michael Kubach: “..Today, authorities all over the world are experimenting with predictive algorithms. That sounds technical and innocent but as we dive deeper into the issue, we realise that the real meaning is rather specific: fraud detection systems in social welfare payment systems. In the meantime, the hitherto banned terminology had it’s come back: welfare or social safety nets are, since a couple of years, en vogue again. But in the centuries-old Western tradition, welfare recipients must be monitored and, if necessary, sanctioned, while those who work and contribute must be assured that there is no waste. So it comes at no surprise that even today’s algorithms focus on the prime suspect, the individual fraudster, the undeserving poor.
Fraud detection systems promise that the taxpayer will no longer fall victim to fraud and efficiency gains can be re-directed to serve more people. The true extent of welfare fraud is regularly exaggerated while the costs of such systems is routinely underestimated. A comparison of the estimated losses and investments doesn’t take place. It is the principle to detect and punish the fraudsters that prevail. Other issues don’t rank high either, for example on how to distinguish between honest mistakes and deliberate fraud. And as case workers spent more time entering and analysing data and in front of a computer screen, the less they have time and inclination to talk to real people and to understand the context of their life at the margins of society.
Thus, it can be said that routinely hundreds of thousands of people are being scored. Example Denmark: Here, a system called Udbetaling Danmark was created in 2012 to streamline the payment of welfare benefits. Its fraud control algorithms can access the personal data of millions of citizens, not all of whom receive welfare payments. In contrast to the hundreds of thousands affected by this data mining, the number of cases referred to the Police for further investigation are minute.
In the city of Rotterdam in the Netherlands every year, data of 30,000 welfare recipients is investigated in order to flag suspected welfare cheats. However, an analysis of its scoring system based on machine learning and algorithms showed systemic discrimination with regard to ethnicity, age, gender, and parenthood. It revealed evidence of other fundamental flaws making the system both inaccurate and unfair. What might appear to a caseworker as a vulnerability is treated by the machine as grounds for suspicion. Despite the scale of data used to calculate risk scores, the output of the system is not better than random guesses. However, the consequences of being flagged by the “suspicion machine” can be drastic, with fraud controllers empowered to turn the lives of suspects inside out.
As reported by the World Bank, the recent Covid-19 pandemic provided a great push to implement digital social welfare systems in the global South. In fact, for the World Bank the so-called Digital Public Infrastructure (DPI), enabling “Digitizing Government to Person Payments (G2Px)”, are as fundamental for social and economic development today as physical infrastructure was for previous generations. Hence, the World Bank is finances globally systems modelled after the Indian Aadhaar system, where more than a billion persons have been registered biometrically. Aadhaar has become, for all intents and purposes, a pre-condition to receive subsidised food and other assistance for 800 million Indian citizens.
Important international aid organisations are not behaving differently from states. The World Food Programme alone holds data of more than 40 million people on its Scope data base. Unfortunately, WFP like other UN organisations, is not subject to data protection laws and the jurisdiction of courts. This makes the communities they have worked with particularly vulnerable.
In most places, the social will become the metric, where logarithms determine the operational conduit for delivering, controlling and withholding assistance, especially welfare payments. In other places, the power of logarithms may go even further, as part of trust systems, creditworthiness, and social credit. These social credit systems for individuals are highly controversial as they require mass surveillance since they aim to track behaviour beyond financial solvency. The social credit score of a citizen might not only suffer from incomplete, or inaccurate data, but also from assessing political loyalties and conformist social behaviour…(More)”.
Big data for whom? Data-driven estimates to prioritize the recovery needs of vulnerable populations after a disaster
Blog and paper by Sabine Loos and David Lallemant: “For years, international agencies have been effusing the benefits of big data for sustainable development. Emerging technology–such as crowdsourcing, satellite imagery, and machine learning–have the power to better inform decision-making, especially those that support the 17 Sustainable Development Goals. When a disaster occurs, overwhelming amounts of big data from emerging technology are produced with the intention to support disaster responders. We are seeing this now with the recent earthquakes in Turkey and Syria: space agencies are processing satellite imagery to map faults and building damage or digital humanitarians are crowdsourcing baseline data like roads and buildings.
Eight years ago, the Nepal 2015 earthquake was no exception–emergency managers received maps of shaking or crowdsourced maps of affected people’s needs from diverse sources. A year later, I began research with a team of folks involved during the response to the earthquake, and I was determined to understand how big data produced after disasters were connected to the long-term effects of the earthquake. Our research team found that a lot of data that was used to guide the recovery focused on building damage, which was often viewed as a proxy for population needs. While building damage information is useful, it does not capture the full array of social, environmental, and physical factors that will lead to disparities in long-term recovery. I assumed information would have been available immediately after the earthquake that was aimed at supporting vulnerable populations. However, as I spent time in Nepal during the years after the 2015 earthquake, speaking with government officials and nongovernmental organizations involved in the response and recovery, I found they lacked key information about the needs of the most vulnerable households–those who would face the greatest obstacles during the recovery from the earthquake. While governmental and nongovernmental actors prioritized the needs of vulnerable households as best as possible with the information available, I was inspired to pursue research that could provide better information more quickly after an earthquake, to inform recovery efforts.
In our paper published in Communications Earth and Environment [link], we develop a data-driven approach to rapidly estimate which areas are likely to fall behind during recovery due to physical, environmental, and social obstacles. This approach leverages survey data on recovery progress combined with geospatial datasets that would be readily available after an event that represent factors expected to impede recovery. To identify communities with disproportionate needs long after a disaster, we propose focusing on those who fall behind in recovery over time, or non-recovery. We focus on non-recovery since it places attention on those who do not recover rather than delineating the characteristics of successful recovery. In addition, in speaking to several groups in Nepal involved in the recovery, they understood vulnerability–a concept that is place-based and can change over time–as those who would not be able to recover due to the earthquake…(More)”
Big Data and Public Policy
Book by Rebecca Moody and Victor Bekkers: “This book provides a comprehensive overview of how the course, content and outcome of policy making is affected by big data. It scrutinises the notion that big and open data makes policymaking a more rational process, in which policy makers are able to predict, assess and evaluate societal problems. It also examines how policy makers deal with big data, the problems and limitations they face, and how big data shapes policymaking on the ground. The book considers big data from various perspectives, not just the political, but also the technological, legal, institutional and ethical dimensions. The potential of big data use in the public sector is also assessed, as well as the risks and dangers this might pose. Through several extended case studies, it demonstrates the dynamics of big data and public policy. Offering a holistic approach to the study of big data, this book will appeal to students and scholars of public policy, public administration and data science, as well as those interested in governance and politics…(More)”.
Data Free Disney
Essay by Janet Vertesy: “…Once upon a time, you could just go to Disneyland. You could get tickets at the gates, stand in line for rides, buy food and tchotchkes, even pick up copies of your favorite Disney movies at a local store. It wasn’t even that long ago. The last time I visited, in 2010, the company didn’t record what I ate for dinner or detect that I went on Pirates of the Caribbean five times. It was none of their business.
But sometime in the last few years, tracking and tracing became their business. Like many corporations out there, Walt Disney Studios spent the last decade transforming into a data company.
The theme parks alone are a data scientist’s dream. Just imagine: 50,000 visitors a day, most equipped with cell phones and a specialized app. Millions of location traces, along with rides statistics, lineup times, and food-order preferences. Thousands and thousands of credit card swipes, each populating a database with names and addresses, each one linking purchases across the park grounds.1 A QR-code scavenger hunt that records the path people took through Star Wars: Galaxy’s Edge. Hotel keycards with entrance times, purchases, snack orders, and more. Millions of photos snapped on rides and security cameras throughout the park, feeding facial-recognition systems. Tickets with names, birthdates, and portraits attached. At Florida’s Disney World, MagicBands—bracelets using RFID (radio-frequency identification) technology—around visitors’ wrists gather all that information plus fingerprints in one place, while sensors ambiently detect their every move. What couldn’t you do with all that data?…(More)”.
Big Data and the Law of War
Essay by Paul Stephan: “Big data looms large in today’s world. Much of the tech sector regards the building up of large sets of searchable data as part (sometimes the greater part) of its business model. Surveillance-oriented states, of which China is the foremost example, use big data to guide and bolster monitoring of their own people as well as potential foreign threats. Many other states are not far behind in the surveillance arms race, notwithstanding the attempts of the European Union to put its metaphorical finger in the dike. Finally, ChatGPT has revived popular interest in artificial intelligence (AI), which uses big data as a means of optimizing the training and algorithm design on which it depends, as a cultural, economic, and social phenomenon.
If big data is growing in significance, might it join territory, people, and property as objects of international conflict, including armed conflict? So far it has not been front and center in Russia’s invasion of Ukraine, the war that currently consumes much of our attention. But future conflicts could certainly feature attacks on big data. China and Taiwan, for example, both have sophisticated technological infrastructures that encompass big data and AI capabilities. The risk that they might find themselves at war in the near future is larger than anyone would like. What, then, might the law of war have to say about big data? More generally, if existing law does not meet our needs, how might new international law address the issue?
In a recent essay, part of an edited volume on “The Future Law of Armed Conflict,” I argue that big data is a resource and therefore a potential target in an armed conflict. I address two issues: Under the law governing the legality of war (jus ad bellum), what kinds of attacks on big data might justify an armed response, touching off a bilateral (or multilateral) armed conflict (a war)? And within an existing armed conflict, what are the rules (jus in bello, also known as international humanitarian law, or IHL) governing such attacks?
The distinction is meaningful. If cyber operations rise to the level of an armed attack, then the targeted state has, according to Article 51 of the U.N. Charter, an “inherent right” to respond with armed force. Moreover, the target need not confine its response to a symmetrical cyber operation. Once attacked, a state may use all forms of armed force in response, albeit subject to the restrictions imposed by IHL. If the state regards, say, a takedown of its financial system as an armed attack, it may respond with missiles…(More)”.