ESRI: “GIS and the 2020 Census: Modernizing Official Statistics provides statistical organizations with the most recent GIS methodologies and technological tools to support census workers’ needs at all the stages of a census. Learn how to plan and carry out census work with GIS using new technologies for field data collection and operations management. International case studies illustrate concepts in practice….(More)”.
As Surveys Falter Big Data Polling Narrows Our Societal Understanding
Kalev Leetaru at Forbes: “One of the most talked-about stories in the world of polling and survey research in recent years has been the gradual death of survey response rates and the reliability of those insights….
The online world’s perceived anonymity has offered some degree of reprieve in which online polls and surveys have often bested traditional approaches in assessing views towards society’s most controversial issues. Yet, here as well increasing public understanding of phishing and online safety are ever more problematic.
The answer has been the rise of “big data” analysis of society’s digital exhaust to fill in the gaps….
Is it truly the same answer though?
Constructing and conducting a well-designed survey means being able to ask the public exactly the questions of interest. Most importantly, it entails being able to ensure representative demographics of respondents.
An online-only poll is unlikely to accurately capture the perspectives of the three quarters of the earth’s population that the digital revolution has left behind. Even within the US, social media platforms are extraordinarily skewed.
The far greater problem is that society’s data exhaust is rarely a perfect match for the questions of greatest interest to policymakers and public.
Cellphone mobility records can offer an exquisitely detailed look at how the people of a city go about their daily lives, but beneath all that blinding light are the invisible members of society not deemed valuable to advertisers and thus not counted. Even for the urban society members whose phones are their ever-present companions, mobility data only goes so far. It can tell us that occupants of a particular part of the city during the workday spend their evenings in a particular part of the city, allowing us to understand their work/life balance, but it offers few insights into their political leanings.
One of the greatest challenges of today’s “big data” surveying is that it requires us to narrow our gaze to only those questions which can be easily answered from the data at hand.
Much as AI’s crisis of bias comes from the field’s steadfast refusal to pay for quality data, settling for highly biased free data, so too has “big data” surveying limited itself largely to datasets it can freely and easily acquire.
The result is that with traditional survey research, we are free to ask the precise questions we are most interested in. With data exhaust research, we must imperfectly shoehorn our questions into the few available metrics. With sufficient creativity it is typically possible to find some way of proxying the given question, but the resulting proxies may be highly unstable, with little understanding of when and where they may fail.
Much like how the early rise of the cluster computing era caused “big data” researchers to limit the questions they asked of their data to just those they could fit into a set of tiny machines, so too has the era of data exhaust surveying forced us to greatly restrict our understanding of society.
Most dangerously, however, big data surveying implicitly means we are measuring only the portion of society our vast commercial surveillance state cares about.
In short, we are only able to measure those deemed of greatest interest to advertisers and thus the most monetizable.
Putting this all together, the decline of traditional survey research has led to the rise of “big data” analysis of society’s data exhaust. Instead of giving us an unprecedented new view into the heartbeat of daily life, this reliance on the unintended output of our digital lives has forced researchers to greatly narrow the questions they can explore and severely skews them to the most “monetizable” portions of society.
In the end, the shift of societal understanding from precision surveys to the big data revolution has led not to an incredible new understanding of what makes us tick, but rather a far smaller, less precise and less accurate view than ever before, just our need to understand ourselves has never been greater….(More)”.
A weather tech startup wants to do forecasts based on cell phone signals
Douglas Heaven at MIT Technology Review: “On 14 April more snow fell on Chicago than it had in nearly 40 years. Weather services didn’t see it coming: they forecast one or two inches at worst. But when the late winter snowstorm came it caused widespread disruption, dumping enough snow that airlines had to cancel more than 700 flights across all of the city’s airports.
One airline did better than most, however. Instead of relying on the usual weather forecasts, it listened to ClimaCell – a Boston-based “weather tech” start-up that claims it can predict the weather more accurately than anyone else. According to the company, its correct forecast of the severity of the coming snowstorm allowed the airline to better manage its schedules and minimize losses due to delays and diversions.
Founded in 2015, ClimaCell has spent the last few years developing the technology and business relationships that allow it to tap into millions of signals from cell phones and other wireless devices around the world. It uses the quality of these signals as a proxy for local weather conditions, such as precipitation and air quality. It also analyzes images from street cameras. It is offering a weather forecasting service to subscribers that it claims is 60 percent more accurate than that of existing providers, such as NOAA.
The internet of weather
The approach makes sense, in principle. Other forecasters use proxies, such as radar signals. But by using information from millions of everyday wireless devices, ClimaCell claims it has a far more fine-grained view of most of the globe than other forecasters get from the existing network of weather sensors, which range from ground-based devices to satellites. (ClimaCell also taps into these, too.)…(More)”.
Introducing the Contractual Wheel of Data Collaboration
Blog by Andrew Young and Stefaan Verhulst: “Earlier this year we launched the Contracts for Data Collaboration (C4DC) initiative — an open collaborative with charter members from The GovLab, UN SDSN Thematic Research Network on Data and Statistics (TReNDS), University of Washington and the World Economic Forum. C4DC seeks to address the inefficiencies of developing contractual agreements for public-private data collaboration by informing and guiding those seeking to establish a data collaborative by developing and making available a shared repository of relevant contractual clauses taken from existing legal agreements. Today TReNDS published “Partnerships Founded on Trust,” a brief capturing some initial findings from the C4DC initiative.
The Contractual Wheel of Data Collaboration [beta]
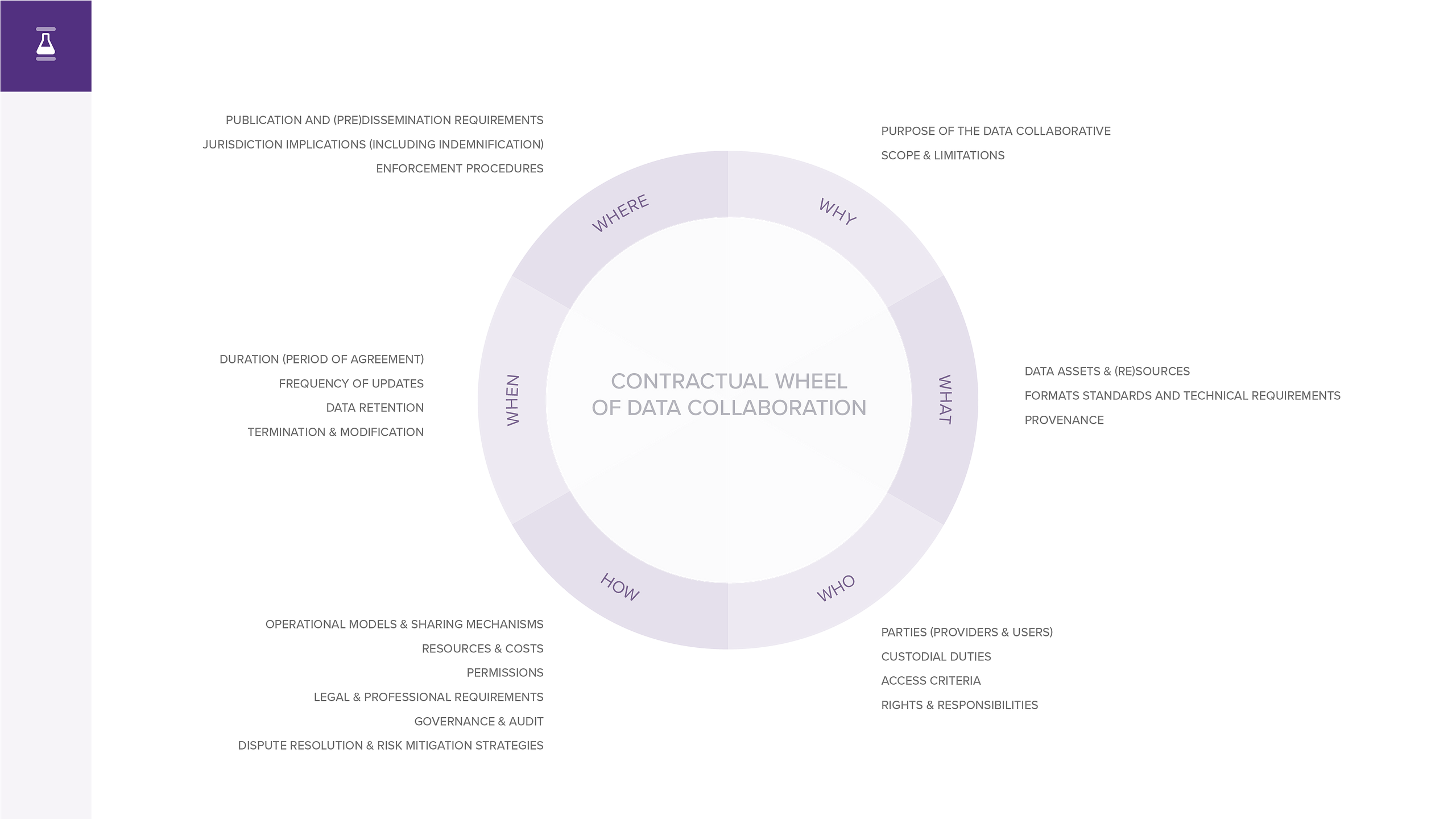
As part of the C4DC effort, and to support Data Stewards in the private sector and decision-makers in the public and civil sectors seeking to establish Data Collaboratives, The GovLab developed the Contractual Wheel of Data Collaboration [beta]. The Wheel seeks to capture key elements involved in data collaboration while demystifying contracts and moving beyond the type of legalese that can create confusion and barriers to experimentation.
The Wheel was developed based on an assessment of existing legal agreements, engagement with The GovLab-facilitated Data Stewards Network, and analysis of the key elements of our Data Collaboratives Methodology. It features 22 legal considerations organized across 6 operational categories that can act as a checklist for the development of a legal agreement between parties participating in a Data Collaborative:…(More)”.
Leveraging Big Data for Social Responsibility
Paper by Cynthia Ann Peterson: “Big data has the potential to revolutionize the way social risks are managed by providing enhanced insight to enable more informed actions to be taken. The objective of this paper is to share the approach taken by PETRONAS to leverage big data to enhance its social performance practice, specifically in social risk assessments and grievance mechanism.
The paper will deliberate on the benefits, challenges and opportunities to improve the management of social risk through analytics, and how PETRONAS has taken those factors into consideration in the enhancement of its social risk assessment and grievance mechanism tools. Key considerations such as disaggregation of data, the appropriate leading and lagging indicators and having a human rights lens to data will also be discussed.
Leveraging on big data is still in its early stages in the social risk space, similar with other areas in the oil and gas industry according to research by Wood Mackenzie. Even so, there are several concerns which include; the aggregation of data may result in risks to minority or vulnerable groups not getting surfaced; privacy breaches which violate human rights and potential discrimination due to prescriptive analysis, such as on a community’s propensity to pose certain social risks to projects or operations. Certainly, there are many challenges ahead which need to be considered, including how best to take a human rights approach to using big data.
Nevertheless, harnessing the power of big data will help social risk practitioners turn a high volume of disparate pieces of raw data from grievance mechanisms and social risk assessments into information that can be used to avoid or mitigate risks now and in the future through predictive technology. Consumer and other industries are benefiting from this leverage now, and social performance practitioners in the oil and gas industry can emulate these proven models….(More)”.
Illuminating Big Data will leave governments in the dark
Robin Wigglesworth in the Financial Times: “Imagine a world where interminable waits for backward-looking, frequently-revised economic data seem as archaically quaint as floppy disks, beepers and a civil internet. This fantasy realm may be closer than you think.
The Bureau of Economic Analysis will soon publish its preliminary estimate for US economic growth in the first three months of the year, finally catching up on its regular schedule after a government shutdown paralysed the agency. But other data are still delayed, and the final official result for US gross domestic product won’t be available until July. Along the way there are likely to be many tweaks.
Collecting timely and accurate data are a Herculean task, especially for an economy as vast and varied as the US’s. But last week’s World Bank-International Monetary Fund’s annual spring meetings offered some clues on a brighter, more digital future for economic data.
The IMF hosted a series of seminars and discussions exploring how the hot new world of Big Data could be harnessed to produce more timely economic figures — and improve economic forecasts. Jiaxiong Yao, an IMF official in its African department, explained how it could use satellites to measure the intensity of night-time lights, and derive a real-time gauge of economic health.
“If a country gets brighter over time, it is growing. If it is getting darker then it probably needs an IMF programme,” he noted. Further sessions explored how the IMF could use machine learning — a popular field of artificial intelligence — to improve its influential but often faulty economic forecasts; and real-time shipping data to map global trade flows.
Sophisticated hedge funds have been mining some of these new “alternative” data sets for some time, but statistical agencies, central banks and multinational organisations such as the IMF and the World Bank are also starting to embrace the potential.
The amount of digital data around the world is already unimaginably vast. As more of our social and economic activity migrates online, the quantity and quality is going to increase exponentially. The potential is mind-boggling. Setting aside the obvious and thorny privacy issues, it is likely to lead to a revolution in the world of economic statistics. …
Yet the biggest issues are not the weaknesses of these new data sets — all statistics have inherent flaws — but their nature and location.
Firstly, it depends on the lax regulatory and personal attitudes towards personal data continuing, and there are signs of a (healthy) backlash brewing.
Secondly, almost all of this alternative data is being generated and stored in the private sector, not by government bodies such as the Bureau of Economic Analysis, Eurostat or the UK’s Office for National Statistics.
Public bodies are generally too poorly funded to buy or clean all this data themselves, meaning hedge funds will benefit from better economic data than the broader public. We might, in fact, need legislation mandating that statistical agencies receive free access to any aggregated private sector data sets that might be useful to their work.
That would ensure that our economic officials and policymakers don’t fly blind in an increasingly illuminated world….(More)”.
Synthetic data: innovation for public good
Blog Post by Catrin Cheung: “What is synthetic data, and how can it be used for public good? ….Synthetic data are artificially generated data that have the look and structure of real data, but do not contain any information on individuals. They also contain more general characteristics that are used to find patterns in the data.
They are modelled on real data, but designed in a way which safeguards the legal, ethical and confidentiality requirements of the original data. Given their resemblance to the original data, synthetic data are useful in a range of situations, for example when data is sensitive or missing. They are used widely as teaching materials, to test code or mathematical models, or as training data for machine learning models….
There’s currently a wealth of research emerging from the health sector, as the nature of data published is often sensitive. Public Health England have synthesised cancer data which can be freely accessed online. NHS Scotland are making advances in cutting-edge machine learning methods such as Variational Auto Encoders and Generative Adversarial Networks (GANs).
There is growing interest in this area of research, and its influence extends beyond the statistical community. While the Data Science Campus have also used GANs to generate synthetic data in their latest research, its power is not limited to data generation. It can be trained to construct features almost identical to our own across imagery, music, speech and text. In fact, GANs have been used to create a painting of Edmond de Belamy, which sold for $432,500 in 2018!
Within the ONS, a pilot to create synthetic versions of securely held Labour Force Survey data has been carried out using a package in R called “synthpop”. This synthetic dataset can be shared with approved researchers to de-bug codes, prior to analysis of data held in the Secure Research Service….
Although much progress is done in this field, one challenge that persists is guaranteeing the accuracy of synthetic data. We must ensure that the statistical properties of synthetic data match properties of the original data.
Additional features, such as the presence of non-numerical data, add to this difficult task. For example, if something is listed as “animal” and can take the possible values “dog”,”cat” or “elephant”, it is difficult to convert this information into a format suitable for precise calculations. Furthermore, given that datasets have different characteristics, there is no straightforward solution that can be applied to all types of data….particular focus was also placed on the use of synthetic data in the field of privacy, following from the challenges and opportunities identified by the National Statistician’s Quality Review of privacy and data confidentiality methods published in December 2018….(More)”.
Predictive Big Data Analytics using the UK Biobank Data
Paper by Ivo D Dinov et al: “The UK Biobank is a rich national health resource that provides enormous opportunities for international researchers to examine, model, and analyze census-like multisource healthcare data. The archive presents several challenges related to aggregation and harmonization of complex data elements, feature heterogeneity and salience, and health analytics. Using 7,614 imaging, clinical, and phenotypic features of 9,914 subjects we performed deep computed phenotyping using unsupervised clustering and derived two distinct sub-cohorts. Using parametric and nonparametric tests, we determined the top 20 most salient features contributing to the cluster separation. Our approach generated decision rules to predict the presence and progression of depression or other mental illnesses by jointly representing and modeling the significant clinical and demographic variables along with the derived salient neuroimaging features. We reported consistency and reliability measures of the derived computed phenotypes and the top salient imaging biomarkers that contributed to the unsupervised clustering. This clinical decision support system identified and utilized holistically the most critical biomarkers for predicting mental health, e.g., depression. External validation of this technique on different populations may lead to reducing healthcare expenses and improving the processes of diagnosis, forecasting, and tracking of normal and pathological aging….(More)”.
Statistics Estonia to coordinate data governance
Article by Miriam van der Sangen at CBS: “In 2018, Statistics Estonia launched a new strategy for the period 2018-2022. This strategy addresses the organisation’s aim to produce statistics more quickly while minimising the response burden on both businesses and citizens. Another element in the strategy is addressing the high expectations in Estonian society regarding the use of data. ‘We aim to transform Statistics Estonia into a national data agency,’ says Director General Mägi. ‘This means our role as a producer of official statistics will be enlarged by data governance responsibilities in the public sector. Taking on such responsibilities requires a clear vision of the whole public data ecosystem and also agreement to establish data stewards in most public sector institutions.’…
the Estonian Parliament passed new legislation that effectively expanded the number of official tasks for Statistics Estonia. Mägi elaborates: ‘Most importantly, we shall be responsible for coordinating data governance. The detailed requirements and conditions of data governance will be specified further in the coming period.’ Under the new Act, Statistics Estonia will also have more possibilities to share data with other parties….
Statistics Estonia is fully committed to producing statistics which are based on big data. Mägi explains: ‘At the moment, we are actively working on two big data projects. One project involves the use of smart electricity meters. In this project, we are looking into ways to visualise business and household electricity consumption information. The second project involves web scraping of prices and enterprise characteristics. This project is still in an initial phase, but we can already see that the use of web scraping can improve the efficiency of our production process.’ We are aiming to extend the web scraping project by also identifying e-commerce and innovation activities of enterprises.’
Yet another ambitious goal for Statistics Estonia lies in the field of data science. ‘Similarly to Statistics Netherlands, we established experimental statistics and data mining activities years ago. Last year, we developed a so-called think-tank service, providing insights from data into all aspects of our lives. Think of birth, education, employment, et cetera. Our key clients are the various ministries, municipalities and the private sector. The main aim in the coming years is to speed up service time thanks to visualisations and data lake solutions.’ …(More)”.
Big Data Applications in Governance and Policy
Introduction to Special Issue of Politics and Governance by Sarah Giest and Reuben Ng: ” Recent literature has been trying to grasp the extent as to which big data applications affect the governance and policymaking of countries and regions (Boyd & Crawford, 2012; Giest, 2017; Höchtl, Parycek, & Schöllhammer, 2015; Poel, Meyer, & Schroeder, 2018). The discussion includes the comparison to e-government and evidence-based policymaking developments that existed long before the idea of big data entered the policy realm. The theoretical extent of this discussion however lacks some of the more practical consequences that come with the active use of data-driven applications. In fact, much of the work focuses on the input-side of policymaking, looking at which data and technology enters the policy process, however very little is dedicated to the output side.
In short, how has big data shaped data governance and policymaking? The contributions to this thematic issue shed light on this question by looking at a range of factors, such as campaigning in the US election (Trish, 2018) or local government data projects (Durrant, Barnett, & Rempel, 2018). The goal is to unpack the mixture of big data applications and existing policy processes in order to understand whether these new tools and applications enhance or hinder policymaking….(More)”.