MIT Technology Review: “Weather forecasting is impressively accurate given how changeable and chaotic Earth’s climate can be. It’s not unusual to get 10-day forecasts with a reasonable level of accuracy.
But there is still much to be done. One challenge for meteorologists is to improve their “nowcasting,” the ability to forecast weather in the next six hours or so at a spatial resolution of a square kilometer or less.
In areas where the weather can change rapidly, that is difficult. And there is much at stake. Agricultural activity is increasingly dependent on nowcasting, and the safety of many sporting events depends on it too. Then there is the risk that sudden rainfall could lead to flash flooding, a growing problem in many areas because of climate change and urbanization. That has implications for infrastructure, such as sewage management, and for safety, since this kind of flooding can kill.
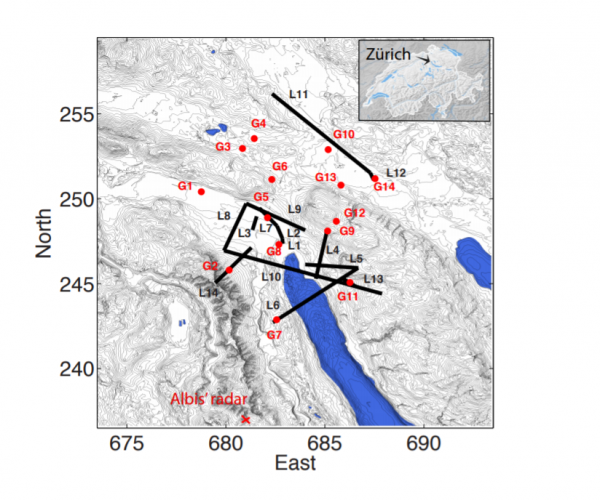
So meteorologists would dearly love to have a better way to make their nowcasts.
Enter Blandine Bianchi from EPFL in Lausanne, Switzerland, and a few colleagues, who have developed a method for combining meteorological data from several sources to produce nowcasts with improved accuracy. Their work has the potential to change the utility of this kind of forecasting for everyone from farmers and gardeners to emergency services and sewage engineers.
Current forecasting is limited by the data and the scale on which it is gathered and processed. For example, satellite data has a spatial resolution of 50 to 100 km and allows the tracking and forecasting of large cloud cells over a time scale of six to nine hours. By contrast, radar data is updated every five minutes, with a spatial resolution of about a kilometer, and leads to predictions on the time scale of one to three hours. Another source of data is the microwave links used by telecommunications companies, which are degraded by rainfall….(More)”