Article by Priceonomics Data Studio: “For all the talk of how data is the new oil and the most valuable resource of any enterprise, there is a deep dark secret companies are reluctant to share — most of the data collected by businesses simply goes unused.
This unknown and unused data, known as dark data comprises more than half the data collected by companies. Given that some estimates indicate that 7.5 septillion (7,700,000,000,000,000,000,000) gigabytes of data are generated every single day, not using most of it is a considerable issue.
In this article, we’ll look at this dark data. Just how much of it is created by companies, what are the reasons this data isn’t being analyzed, and what are the costs and implications of companies not using the majority of the data they collect.
Before diving into the analysis, it’s worth spending a moment clarifying what we mean by the term “dark data.” Gartner defines dark data as:
“The information assets organizations collect, process and store during regular business activities, but generally fail to use for other purposes (for example, analytics, business relationships and direct monetizing).
To learn more about this phenomenon, Splunk commissioned a global survey of 1,300+ business leaders to better understand how much data they collect, and how much is dark. Respondents were from IT and business roles, and were located in Australia, China, France, Germany, Japan, the United States, and the United Kingdom. across various industries. For the report, Splunk defines dark data as: “all the unknown and untapped data across an organization, generated by systems, devices and interactions.”
While the costs of storing data has decreased overtime, the cost of saving septillions of gigabytes of wasted data is still significant. What’s more, during this time the strategic importance of data has increased as companies have found more and more uses for it. Given the cost of storage and the value of data, why does so much of it go unused?
The following chart shows the reasons why dark data isn’t currently being harnessed:
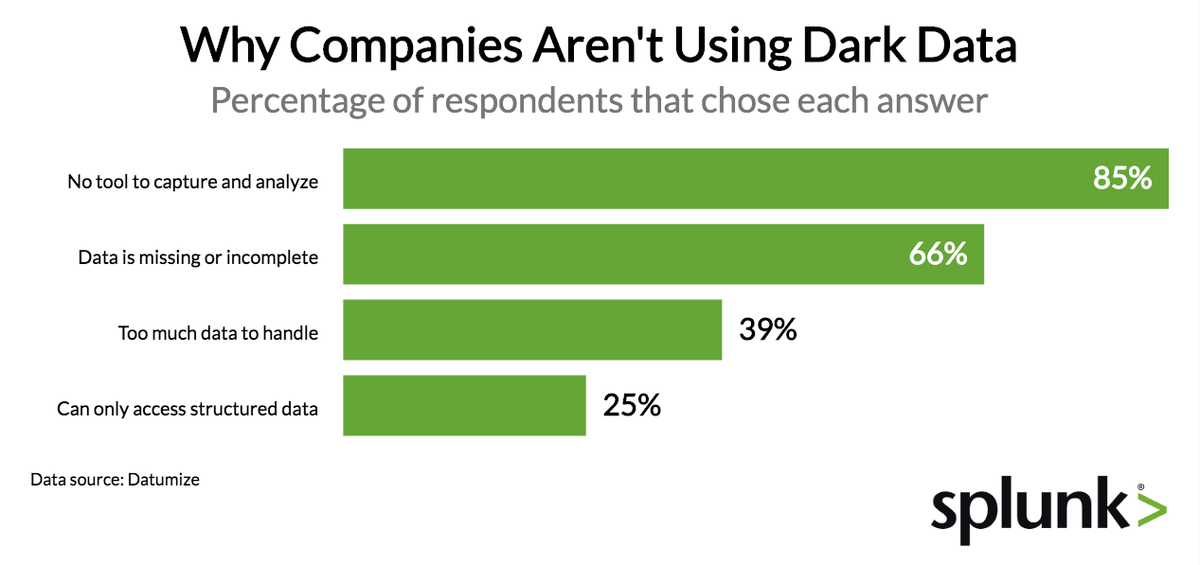
By a large margin, the number one reason given for not using dark data is that companies lack a tool to capture or analyze the data. Companies accumulate data from server logs, GPS networks, security tools, call records, web traffic and more. Companies track everything from digital transactions to the temperature of their server rooms to the contents of retail shelves. Most of this data lies in separate systems, is unstructured, and cannot be connected or analyzed.
Second, the data captured just isn’t good enough. You might have important customer information about a transaction, but it’s missing location or other important metadata because that information sits somewhere else or was never captured in useable format.
Additionally, dark data exists because there is simply too much data out there and a lot of is unstructured. The larger the dataset (or the less structured it is), the more sophisticated the tool required for analysis. Additionally, these kinds of datasets often time require analysis by individuals with significant data science expertise who are often is short supply.
The implications of the prevalence are vast. As a result of the data deluge, companies often don’t know where all the sensitive data is stored and can’t be confident they are complying with consumer data protection measures like GDPR. …(More)”.