Paper by Joshua S. Gans: “Exploitation of disruptive technologies often requires resource deployment that creates conflict if there are divergent beliefs regarding the efficacy of a new technology. This arises when a visionary agent has more optimistic beliefs about a technological opportunity. Exploration in the form of experiments can be persuasive when beliefs differ by mitigating disagreement and its costs. This paper examines experimental choice when experiments need to persuade as well as inform. It is shown that, due to resource constraints, persuasion factors more highly for entrepreneurial than incumbent firms. However, incumbent firms, despite being able to redeploy resources using authority, are constrained in adoption as exploration cannot mitigate the costs of disagreement…(More)”.
The Labor Market Impacts of Technological Change: From Unbridled Enthusiasm to Qualified Optimism to Vast Uncertainty
NBER Working Paper by David Autor: “This review considers the evolution of economic thinking on the relationship between digital technology and inequality across four decades, encompassing four related but intellectually distinct paradigms, which I refer to as the education race, the task polarization model, the automation-reinstatement race, and the era of Artificial Intelligence uncertainty. The nuance of economic understanding has improved across these epochs. Yet, traditional economic optimism about the beneficent effects of technology for productivity and welfare has eroded as understanding has advanced. Given this intellectual trajectory, it would be natural to forecast an even darker horizon ahead. I refrain from doing so because forecasting the “consequences” of technological change treats the future as a fate to be divined rather than an expedition to be undertaken. I conclude by discussing opportunities and challenges that we collectively face in shaping this future….(More)”.
Open data: The building block of 21st century (open) science
Paper by Corina Pascu and Jean-Claude Burgelman: “Given this irreversibility of data driven and reproducible science and the role machines will play in that, it is foreseeable that the production of scientific knowledge will be more like a constant flow of updated data driven outputs, rather than a unique publication/article of some sort. Indeed, the future of scholarly publishing will be more based on the publication of data/insights with the article as a narrative.
For open data to be valuable, reproducibility is a sine qua non (King2011; Piwowar, Vision and Whitlock2011) and—equally important as most of the societal grand challenges require several sciences to work together—essential for interdisciplinarity.
This trend correlates with the already ongoing observed epistemic shift in the rationale of science: from demonstrating the absolute truth via a unique narrative (article or publication), to the best possible understanding what at that moment is needed to move forward in the production of knowledge to address problem “X” (de Regt2017).
Science in the 21st century will be thus be more “liquid,” enabled by open science and data practices and supported or even co-produced by artificial intelligence (AI) tools and services, and thus a continuous flow of knowledge produced and used by (mainly) machines and people. In this paradigm, an article will be the “atomic” entity and often the least important output of the knowledge stream and scholarship production. Publishing will offer in the first place a platform where all parts of the knowledge stream will be made available as such via peer review.
The new frontier in open science as well as where most of future revenue will be made, will be via value added data services (such as mining, intelligence, and networking) for people and machines. The use of AI is on the rise in society, but also on all aspects of research and science: what can be put in an algorithm will be put; the machines and deep learning add factor “X.”
AI services for science 4 are already being made along the research process: data discovery and analysis and knowledge extraction out of research artefacts are accelerated with the use of AI. AI technologies also help to maximize the efficiency of the publishing process and make peer-review more objective5 (Table 1).
Table 1. Examples of AI services for science already being developed
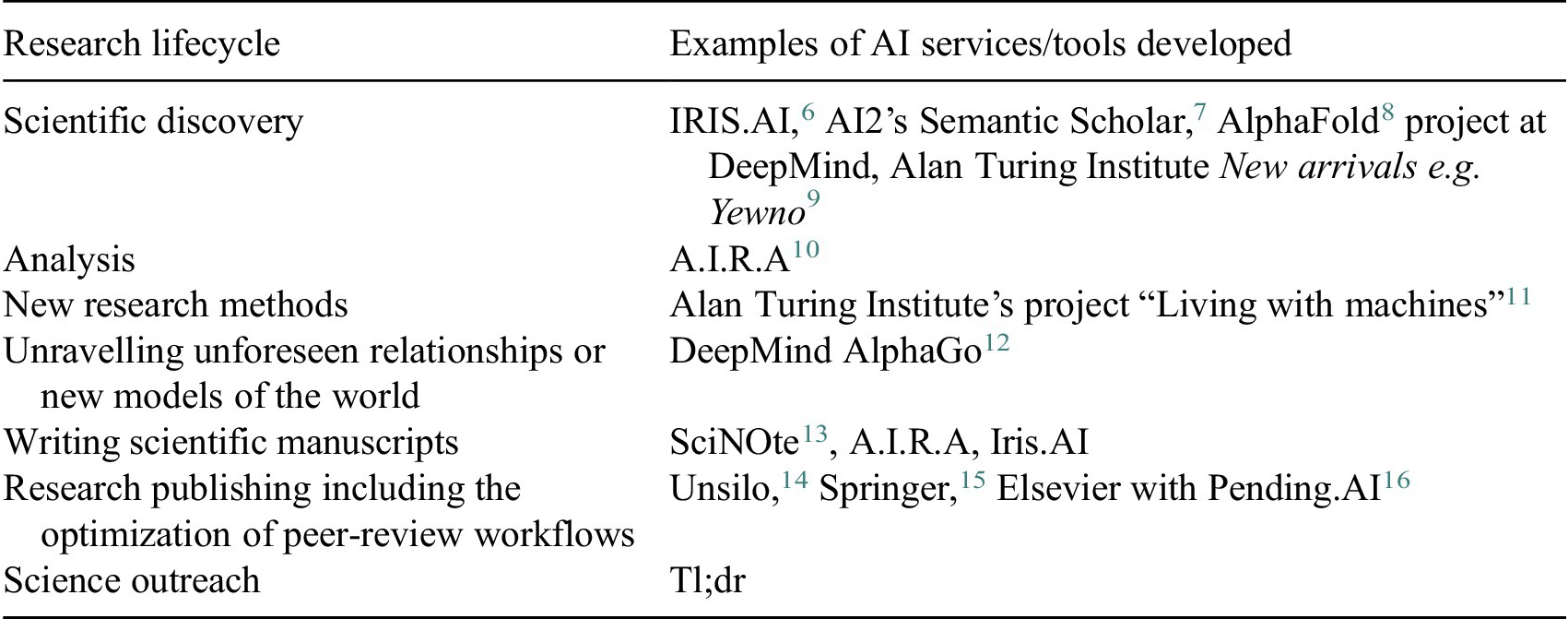
Abbreviation: AI, artificial intelligence.
Source: Authors’ research based on public sources, 2021.
Ultimately, actionable knowledge and translation of its benefits to society will be handled by humans in the “machine era” for decades to come. But as computers are indispensable research assistants, we need to make what we publish understandable to them.
The availability of data that are “FAIR by design” and shared Application Programming Interfaces (APIs) will allow new ways of collaboration between scientists and machines to make the best use of research digital objects of any kind. The more findable, accessible, interoperable, and reusable (FAIR) data resources will become available, the more it will be possible to use AI to extract and analyze new valuable information. The main challenge is to master the interoperability and quality of research data…(More)”.
The Impact of Public Transparency Infrastructure on Data Journalism: A Comparative Analysis between Information-Rich and Information-Poor Countries
Paper by Lindita Camaj, Jason Martin & Gerry Lanosga: “This study surveyed data journalists from 71 countries to compare how public transparency infrastructure influences data journalism practices around the world. Emphasizing cross-national differences in data access, results suggest that technical and economic inequalities that affect the implementation of the open data infrastructures can produce unequal data access and widen the gap in data journalism practices between information-rich and information-poor countries. Further, while journalists operating in open data infrastructure are more likely to exhibit a dependency on pre-processed public data, journalists operating in closed data infrastructures are more likely to use Access to Information legislation. We discuss the implications of our results for understanding the development of data journalism models in cross-national contexts…(More)”
“Co-construction” in Deliberative Democracy: Lessons from the French Citizens’ Convention for Climate
Paper by L.G. Giraudet et al: “Launched in 2019, the French Citizens’ Convention for Climate (CCC) tasked 150 randomly-chosen citizens with proposing fair and effective measures to fight climate change. This was to be fulfilled through an “innovative co-construction procedure,” involving some unspecified external input alongside that from the citizens. Did inputs from the steering bodies undermine the citizens’ accountability for the output? Did co-construction help the output resonate with the general public, as is expected from a citizens’ assembly? To answer these questions, we build on our unique experience in observing the CCC proceedings and documenting them with qualitative and quantitative data. We find that the steering bodies’ input, albeit significant, did not impair the citizens’ agency, creativity and freedom of choice. While succeeding in creating consensus among the citizens who were involved, this co-constructive approach however failed to generate significant support among the broader public. These results call for a strengthening of the commitment structure that determines how follow-up on the proposals from a citizens’ assembly should be conducted…(More)”.
The Bare Minimum of Theory: A Definitional Definition for the Social Sciences
Paper by Chitu Okoli: “The ongoing debates in the information systems (IS) discipline on the nature of theory are implicitly rooted in different epistemologies of the social sciences and in a lack of consensus on a definition of theory. Thus, we focus here on the much-neglected topic of what constitutes the bare minimum of what can possibly be considered theory—only by carefully understanding the bare minimum can we really understand the essence of what makes a theory a theory. We definitionally define a theory in the social sciences as an explanation of the relationship between two or more measurable concepts. (“Measurable” refers to qualitative coding and inference of mechanisms, as well as quantitative magnitudes.) The rigorous justification of each element of this definition helps to resolve issues such as providing a consistent basis of determining what qualifies as theory; the value of other knowledge contributions that are not theory; how to identify theories regardless of if they are named; and a call to recognize diverse forms of theorizing across the social science epistemologies of positivism, interpretivism, critical social theory, critical realism, and pragmatism. Although focused on IS, most of these issues are pertinent to any scholarly discipline within the social sciences…(More)”.
Can politicians and citizens deliberate together? Evidence from a local deliberative mini-public
Paper by Kimmo Grönlund, Kaisa Herne, Maija Jäske, and Mikko Värttö: “In a deliberative mini-public, a representative number of citizens receive information and discuss given policy topics in facilitated small groups. Typically, mini-publics are most effective politically and can have the most impact on policy-making when they are connected to democratic decision-making processes. Theorists have put forward possible mechanisms that may enhance this linkage, one of which is involving politicians within mini-publics with citizens. However, although much research to date has focussed on mini-publics with many citizen participants, there is little analysis of mini-publics with politicians as coparticipants. In this study, we ask how involving politicians in mini-publics influences both participating citizens’ opinions and citizens’ and politicians’ perceptions of the quality of the mini-public deliberations. We organised an online mini-public, together with the City of Turku, Finland, on the topic of transport planning. The participants (n = 171) were recruited from a random sample and discussed the topic in facilitated small groups (n = 21). Pre- and postdeliberation surveys were collected. The effect of politicians on mini-publics was studied using an experimental intervention: in half of the groups, local politicians (two per group) participated, whereas in the other half, citizens deliberated among themselves. Although we found that the participating citizens’ opinions changed, no trace of differences between the two treatment groups was reported. We conclude that politicians, at least when they are in a clear minority in the deliberating small groups, can deliberate with citizens without negatively affecting internal inclusion and the quality of deliberation within mini-publics….(More)”.
Mobile phone data reveal the effects of violence on internal displacement in Afghanistan
Paper by Nearly 50 million people globally have been internally displaced due to conflict, persecution and human rights violations. However, the study of internally displaced persons—and the design of policies to assist them—is complicated by the fact that these people are often underrepresented in surveys and official statistics. We develop an approach to measure the impact of violence on internal displacement using anonymized high-frequency mobile phone data. We use this approach to quantify the short- and long-term impacts of violence on internal displacement in Afghanistan, a country that has experienced decades of conflict. Our results highlight how displacement depends on the nature of violence. High-casualty events, and violence involving the Islamic State, cause the most displacement. Provincial capitals act as magnets for people fleeing violence in outlying areas. Our work illustrates the potential for non-traditional data sources to facilitate research and policymaking in conflict settings….(More)”.
Automating the Analysis of Online Deliberation? Comparing computational analyses of polarized discussions on climate change to established content analysis
Paper by Lisa Oswald: “High-quality discussions can help people acquire an adequate understanding of issues and alleviate mechanisms of opinion polarization. However, the extent to which the quality of the online public discourse contributes is contested. Facing the importance and the sheer volume of online discussions, reliable computational approaches to assess the deliberative quality of online discussions at scale would open a new era of deliberation research. But is it possible to automate the assessment of deliberative quality? I compare structural features of discussion threads and simple text-based measures to established manual content analysis by applying all measures to online discussions on ‘Reddit’ that deal with the 2020 wildfires in Australia and California. I further com pare discussions between two ideologically opposite online communities, one featuring discussions in line with the scientific consensus and one featuring climate change skepticism. While no single computational measure can capture the multidimensional concept of deliberative quality, I find that (1) measures of structural complexity capture engagement and participation as preconditions for deliberation, (2) the length of comments is correlated with manual measures of argumentation, and (3) automated toxicity scores are correlated with manual measures of respect. While the presented computational approaches cannot replace indepth content coding, the findings imply that selected automated measures can be useful, scalable additions to the measurement repertoire for specific dimensions of online deliberation. I discuss implications for communication research and platform regulation and suggest interdisciplinary research to synthesize past content coding efforts using machine learning….(More)”.
GDPR and the Lost Generation of Innovative Apps
Paper by Rebecca Janßen, Reinhold Kesler, Michael E. Kummer & Joel Waldfogel: “Using data on 4.1 million apps at the Google Play Store from 2016 to 2019, we document that GDPR induced the exit of about a third of available apps; and in the quarters following implementation, entry of new apps fell by half. We estimate a structural model of demand and entry in the app market. Comparing long-run equilibria with and without GDPR, we find that GDPR reduces consumer surplus and aggregate app usage by about a third. Whatever the privacy benefits of GDPR, they come at substantial costs in foregone innovation…(More)”.