CCCBLab: “In recent years we have been witnessing a constant trickle of news on artificial intelligence, machine learning and computer vision. We are told that machines learn, see, create… and all this builds up a discourse based on novelty, on a possible future and on a series of worries and hopes. It is difficult, sometimes, to figure out in this landscape which are real developments, and which are fantasies or warnings. And, undoubtedly, this fog that surrounds it forms part of the power that we grant, both in the present and on credit, to these tools, and of the negative and positive concerns that they arouse in us. Many of these discourses may fall into the field of false debates or, at least, of the return of old debates. Thus, in the classical artistic field, associated with the discourse on creation and authorship, there is discussion regarding the entity to be awarded to the images created with these tools. (Yet wasn’t the argument against photography in art that it was an image created automatically and without human participation? And wasn’t that also an argument in favour of taking it and using it to put an end to a certain idea of art?)
Metaphors are essential in the discourse on all digital tools and the power that they have. Are expressions such as “intelligence”, “vision”, “learning”, “neural” and the entire range of similar words the most adequate for defining these types of tools? Probably not, above all if their metaphorical nature is sidestepped. We would not understand them in the same way if we called them tools of probabilistic classification or if instead of saying that an artificial intelligence “has painted” a Rembrandt, we said that it has produced a statistical reproduction of his style (something which is still surprising, and to be celebrated, of course). These names construct an entity for these tools that endows them with a supposed autonomy and independence upon which their future authority is based.
Because that is what it’s about in many discourses: constructing a characterisation that legitimises an objective or non-human capacity in data analysis….
We now find ourselves in what is, probably, the point of the first cultural reception of these tools. Of their development in fields of research and applications that have already been derived, we are moving on to their presence in the public discourse. It is in this situation and context, where we do not fully know the breadth and characteristics of these technologies (meaning fears are more abstract and diffuse and, thus, more present and powerful), when it is especially important to understand what we are talking about, to appropriate the tools and to intervene in the discourses. Before their possibilities are restricted and solidified until they seem indisputable, it is necessary to experiment with them and reflect on them; taking advantage of the fact that we can still easily perceive them as in creation, malleable and open.
In our projects The Bad Pupil. Critical pedagogy for artificial intelligences and Latent Spaces. Machinic Imaginations we have tried to approach to these tools and their imaginary. In the statement of intentions of the former, we expressed our desire, in the face of the regulatory context and the metaphor of machine learning, to defend the bad pupil as one who escapes the norm. And also how, faced with an artificial intelligence that seeks to replicate the human on inhuman scales, it is necessary to defend and construct a non-mimetic one that produces unexpected relations and images.
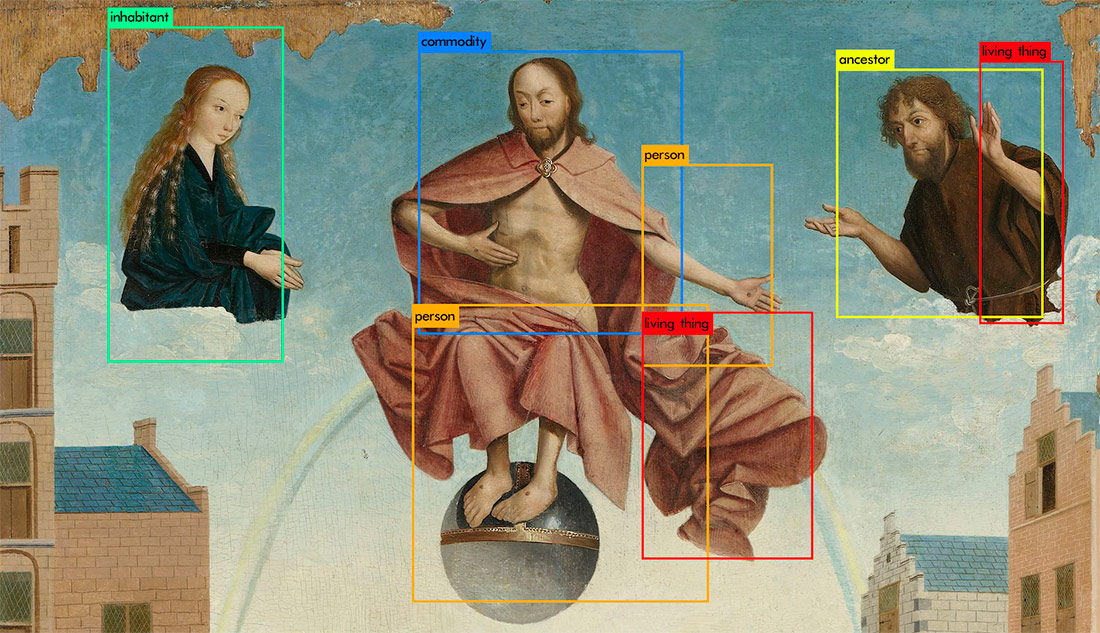
Fragment of De zeven werken van barmhartigheid, Meester van Alkmaar, 1504 (Rijksmuseum, Amsterdam) analysed with YOLO9000 | The Bad Pupil – Estampa
Both projects are also attempts to appropriate these tools, which means, first of all, escaping industrial barriers and their standards. In this field in which mass data are an asset within reach of big companies, employing quantitively poor datasets and non-industrial calculation potentials is not just a need but a demand….(More)”.