Book by IAPP: “The Guide to U.S. Government Practice on Global Sharing of Personal Information, Third Edition is a reference tool on U.S. government practice in G2G-sharing arrangements. The third edition contains new agreements, including the U.S.-U.K. Cloud Act Agreement, EU-U.S. Umbrella Agreement, United States-Mexico-Canada Agreement, and EU-U.S. Privacy Shield framework. This book examines those agreements as a way of establishing how practice has evolved. In addition to reviewing past agreements, international privacy principles of the Organization for Economic Co-operation and Development and Asian-Pacific Economic Cooperation will be reviewed for their relevance to G2G sharing. The guide is intended for lawyers, privacy professionals and individuals who wish to understand U.S. practice for sharing personal information across borders….(More)”.
How Billionaires Can Fund Moonshot Efforts to Save the World
Essay by Ivan Amato: “For the past year, since the 50th anniversary of the original moon landing and amid the harsh entrance and unfolding of a pandemic that has affected the entire globe’s citizenry, I have been running a philanthropy-supported publishing experiment on Medium.com titled the Moonshot Catalog. The goal has been to inspire the nation’s more than 2,000 ultrawealthy households to mobilize a smidgeon more — even 1 percent more — of their collective wealth to help solve big problems that threaten our future.
A single percent may seem a small fraction to devote. But when you consider that the richest families have amassed a net worth of more than $4 trillion, that 1 percent tops $40 billion — enough to make a real difference in any number of ways. This truth only magnifies now as we approach a more honest reality-based acknowledgment of the systemic racial and social inequities and injustices that have shunted so much wealth, privilege, and security into such a rarefied micropercentage of the world’s 7.8 billion people.
Such was the simple conceit underlying the Moonshot Catalog, which just came to a close: The deepest pocketed among us would up their philanthropy game if they were more aware of hugely consequential projects they could help usher to the finish line by donating a tad more of the wealth they control….
The first moonshot articles had titles including “Feeding 2050’s Ten Billion People,” “Taming the Diseases of Aging,” and the now tragically premonitional “Ending Pandemic Disease.” Subsequent articles featured achievable solutions for our carbon-emission crisis, including ones replacing current cement and cooling technologies, underappreciated perpetrators of climate change that are responsible for some 16 percent of the world’s carbon emissions; next-generation battery technology, without which much of the potential benefit of renewable energy will remain untapped; advanced nuclear-power plants safe enough to help enable a carbon-neutral economy; and hastening the arrival of fusion energy….
Common to these projects, and others such as the UN’s Sustainability Development Goals, is the huge and difficult commitment each one demands. Many require a unique, creative, and sustained synthesis of science, engineering, entrepreneurship, policy and financial support, and international cooperation.
But there is no magical thinking in the Catalog. The projects are demonstrably doable. What’s more, humanity already has successfully taken on comparably ambitious challenges. Think of the eradication of polio, the development of birth-control technologies, the mitigation of acid rain and the ozone hole, and the great, albeit imperfect, public-health win of municipal water treatment. Oh, and the 1969 moonshot….(More)”.
Statistics, lies and the virus: lessons from a pandemic
Tim Hartford at the Financial Times: “Will this year be 1954 all over again? Forgive me, I have become obsessed with 1954, not because it offers another example of a pandemic (that was 1957) or an economic disaster (there was a mild US downturn in 1953), but for more parochial reasons. Nineteen fifty-four saw the appearance of two contrasting visions for the world of statistics — visions that have shaped our politics, our media and our health. This year confronts us with a similar choice.
The first of these visions was presented in How to Lie with Statistics, a book by a US journalist named Darrell Huff. Brisk, intelligent and witty, it is a little marvel of numerical communication. The book received rave reviews at the time, has been praised by many statisticians over the years and is said to be the best-selling work on the subject ever published. It is also an exercise in scorn: read it and you may be disinclined to believe a number-based claim ever again….
But they can — and back in 1954, the alternative perspective was embodied in the publication of an academic paper by the British epidemiologists Richard Doll and Austin Bradford Hill. They marshalled some of the first compelling evidence that smoking cigarettes dramatically increases the risk of lung cancer. The data they assembled persuaded both men to quit smoking and helped save tens of millions of lives by prompting others to do likewise. This was no statistical trickery, but a contribution to public health that is almost impossible to exaggerate…
As described in books such as Merchants of Doubt by Erik Conway and Naomi Oreskes, this industry perfected the tactics of spreading uncertainty: calling for more research, emphasising doubt and the need to avoid drastic steps, highlighting disagreements between experts and funding alternative lines of inquiry. The same tactics, and sometimes even the same personnel, were later deployed to cast doubt on climate science. These tactics are powerful in part because they echo the ideals of science.
It is a short step from the Royal Society’s motto, “nullius in verba” (take nobody’s word for it), to the corrosive nihilism of “nobody knows anything”. So will 2020 be another 1954? From the point of view of statistics, we seem to be standing at another fork in the road.
The disinformation is still out there, as the public understanding of Covid-19 has been muddied by conspiracy theorists, trolls and government spin doctors. Yet the information is out there too. The value of gathering and rigorously analysing data has rarely been more evident. Faced with a complete mystery at the start of the year, statisticians, scientists and epidemiologists have been working miracles. I hope that we choose the right fork, because the pandemic has lessons to teach us about statistics — and vice versa — if we are willing to learn…(More)”.
Monitoring global digital gender inequality using the online populations of Facebook and Google
Paper by Ridhi Kashyap, Masoomali Fatehkia, Reham Al Tamime, and Ingmar Weber: “Background: In recognition of the empowering potential of digital technologies, gender equality in internet access and digital skills is an important target in the United Nations (UN) Sustainable Development Goals (SDGs). Gender-disaggregated data on internet use are limited, particularly in less developed countries.
Objective: We leverage anonymous, aggregate data on the online populations of Google and Facebook users available from their advertising platforms to fill existing data gaps and measure global digital gender inequality.
Methods: We generate indicators of country-level gender gaps on Google and Facebook. Using these online indicators independently and in combination with offline development indicators, we build regression models to predict gender gaps in internet use and digital skills computed using available survey data from the International Telecommunications Union (ITU).
Results: We find that women are significantly underrepresented in the online populations of Google and Facebook in South Asia and sub-Saharan Africa. These platform-specific gender gaps are a strong predictor that women lack internet access and basic digital skills in these populations. Comparing platforms, we find Facebook gender gap indicators perform better than Google indicators at predicting ITU internet use and low-level digital-skill gender gaps. Models using these online indicators outperform those using only offline development indicators. The best performing models, however, are those that combine Facebook and Google online indicators with a country’s development indicators such as the Human Development Index….(More)”.
Building Capacity for Evidence-Informed Policy-Making
OECD Report: “This report analyses the skills and capacities governments need to strengthen evidence-informed policy-making (EIPM) and identifies a range of possible interventions that are available to foster greater uptake of evidence. Increasing governments’ capacity for evidence-informed is a critical part of good public governance. However, an effective connection between the supply and the demand for evidence in the policy-making process remains elusive. This report offers concrete tools and a set of good practices for how the public sector can support senior officials, experts and advisors working at the political/administrative interface. This support entails investing in capability, opportunity and motivation and through behavioral changes. The report identifies a core skillset for EIPM at the individual level, including the capacity for understanding, obtaining, assessing, using, engaging with stakeholders, and applying evidence, which was developed in collaboration with the European Commission Joint Research Centre. It also identifies a set of capacities at the organisational level that can be put in place across the machinery of government, throughout the role of interventions, strategies and tools to strengthen these capacities. The report concludes with a set of recommendations to assist governments in building their capacities…(More)”.
The Razor’s Edge: Liberalizing the Digital Surveillance Ecosystem
Report by CNAS: “The COVID-19 pandemic is accelerating global trends in digital surveillance. Public health imperatives, combined with opportunism by autocratic regimes and authoritarian-leaning leaders, are expanding personal data collection and surveillance. This tendency toward increased surveillance is taking shape differently in repressive regimes, open societies, and the nation-states in between.
China, run by the Chinese Communist Party (CCP), is leading the world in using technology to enforce social control, monitor populations, and influence behavior. Part of maximizing this control depends on data aggregation and a growing capacity to link the digital and physical world in real time, where online offenses result in brisk repercussions. Further, China is increasing investments in surveillance technology and attempting to influence the patterns of technology’s global use through the export of authoritarian norms, values, and governance practices. For example, China champions its own technology standards to the rest of the world, while simultaneously peddling legislative models abroad that facilitate access to personal data by the state. Today, the COVID-19 pandemic offers China and other authoritarian nations the opportunity to test and expand their existing surveillance powers internally, as well as make these more extensive measures permanent.
Global swing states are already exhibiting troubling trends in their use of digital surveillance, including establishing centralized, government-held databases and trading surveillance practices with authoritarian regimes. Amid the pandemic, swing states like India seem to be taking cues from autocratic regimes by mandating the download of government-enabled contact-tracing applications. Yet, for now, these swing states appear responsive to their citizenry and sensitive to public agitation over privacy concerns.
Today, the COVID-19 pandemic offers China and other authoritarian nations the opportunity to test and expand their existing surveillance powers internally, as well as make these more extensive measures permanent.
Open societies and democracies can demonstrate global surveillance trends similar to authoritarian regimes and swing states, including the expansion of digital surveillance in the name of public safety and growing private sector capabilities to collect and analyze data on individuals. Yet these trends toward greater surveillance still occur within the context of pluralistic, open societies that feature ongoing debates about the limits of surveillance. However, the pandemic stands to shift the debate in these countries from skepticism over personal data collection to wider acceptance. Thus far, the spectrum of responses to public surveillance reflects the diversity of democracies’ citizenry and processes….(More)”.
The open source movement takes on climate data
Article by Heather Clancy: “…many companies are moving to disclose “climate risk,” although far fewer are moving to actually minimize it. And as those tasked with preparing those reports can attest, the process of gathering the data for them is frustrating and complex, especially as the level of detail desired and required by investors becomes deeper.
That pain point was the inspiration for a new climate data project launched this week that will be spearheaded by the Linux Foundation, the nonprofit host organization for thousands of the most influential open source software and data initiatives in the world such as GitHub. The foundation is central to the evolution of the Linux software that runs in the back offices of most major financial services firms.
There are four powerful founding members for the new group, the LF Climate Finance Foundation (LFCF): Insurance and asset management company Allianz, cloud software giants Amazon and Microsoft, and data intelligence powerhouse S&P Global. The foundation’s “planning team” includes World Wide Fund for Nature (WWF), Ceres and the Sustainability Account Standards Board (SASB).
The group’s intention is to collaborate on an open source project called the OS-Climate platform, which will include economic and physical risk scenarios that investors, regulators, companies, financial analysts and others can use for their analysis.
The idea is to create a “public service utility” where certain types of climate data can be accessed easily, then combined with other, more proprietary information that someone might be using for risk analysis, according to Truman Semans, CEO of OS-Climate, who was instrumental in getting the effort off the ground. “There are a whole lot of initiatives out there that address pieces of the puzzle, but no unified platform to allow those to interoperate,” he told me. There are a whole lot of initiatives out there that address pieces of the puzzle, but no unified platform to allow those to interoperate.
Why does this matter? It helps to understand the history of open source software, which was once a thing that many powerful software companies, notably Microsoft, abhorred because they were worried about the financial hit on their intellectual property. Flash forward to today and the open source software movement, “staffed” by literally millions of software developers, is credited with accelerating the creation of common system-level elements so that companies can focus their own resources on solving problems directly related to their business.
In short, this budding effort could make the right data available more quickly, so that businesses — particularly financial institutions — can make better informed decisions.
Or, as Microsoft’s chief intellectual property counsel, Jennifer Yokoyama, observed in the announcement press release: “Addressing climate issues in a meaningful way requires people and organizations to have access to data to better understand the impact of their actions. Opening up and sharing our contribution of significant and relevant sustainability data through the LF Climate Finance Foundation will help advance the financial modeling and understanding of climate change impact — an important step in affecting political change. We’re excited to collaborate with the other founding members and hope additional organizations will join.”…(More)”
UK’s National Data Strategy
DCMS (UK): “…With the increasing ascendance of data, it has become ever-more important that the government removes the unnecessary barriers that prevent businesses and organisations from accessing such information.
The importance of data sharing was demonstrated during the first few months of the coronavirus pandemic, when government departments, local authorities, charities and the private sector came together to provide essential services. One notable example is the Vulnerable Person Service, which in a very short space of time enabled secure data-sharing across the public and private sectors to provide millions of food deliveries and access to priority supermarket delivery slots for clinically extremely vulnerable people.
Aggregation of data from different sources can also lead to new insights that otherwise would not have been possible. For example, the Connected Health Cities project anonymises and links data from different health and social care services, providing new insights into the way services are used.
Vitally, data sharing can also fuel growth and innovation.20 For new and innovating organisations, increasing data availability will mean that they, too, will be able to gain better insights from their work and access new markets – from charities able to pool beneficiary data to better evaluate the effectiveness of interventions, to new entrants able to access new markets. Often this happens as part of commercial arrangements; in other instances government has sought to intervene where there are clear consumer benefits, such as in relation to Open Banking and Smart Data. Government has also invested in the research and development of new mechanisms for better data sharing, such as the Office for AI and Innovate UK’s partnership with the Open Data Institute to explore data trusts.21
However, our call for evidence, along with engagement with stakeholders, has identified a range of barriers to data availability, including:
- a culture of risk aversion
- issues with current licensing regulations
- market barriers to greater re-use, including data hoarding and differential market power
- inconsistent formatting of public sector data
- issues pertaining to the discoverability of data
- privacy and security concerns
- the benefits relating to increased data sharing not always being felt by the organisation incurring the cost of collection and maintenance
This is a complex environment, and heavy-handed intervention may have the unwanted effect of reducing incentives to collect, maintain and share data for the benefit of the UK. It is clear that any way forward must be carefully considered to avoid unintended negative consequences. There is a balance to be struck between maintaining appropriate commercial incentives to collect data, while ensuring that data can be used widely for the benefit of the UK. For personal data, we must also take account of the balance between individual rights and public benefit.
This is a new issue for all digital economies that has come to the fore as data has become a significant modern, economic asset. Our approach will take account of those incentives, and consider how innovation can overcome perceived barriers to availability. For example, it can be limited to users with specific characteristics, by licence or regulator accreditation; it can be shared within a collaborating group of organisations; there may also be value in creating and sharing synthetic data to support research and innovation, as well as other privacy-enhancing technologies and techniques….(More)”.
The Pandemic Is No Excuse to Surveil Students
Zeynep Tufekci in the Atlantic: “In Michigan, a small liberal-arts college is requiring students to install an app called Aura, which tracks their location in real time, before they come to campus. Oakland University, also in Michigan, announced a mandatory wearable that would track symptoms, but, facing a student-led petition, then said it would be optional. The University of Missouri, too, has an app that tracks when students enter and exit classrooms. This practice is spreading: In an attempt to open during the pandemic, many universities and colleges around the country are forcing students to download location-tracking apps, sometimes as a condition of enrollment. Many of these apps function via Bluetooth sensors or Wi-Fi networks. When students enter a classroom, their phone informs a sensor that’s been installed in the room, or the app checks the Wi-Fi networks nearby to determine the phone’s location.
As a university professor, I’ve seen surveillance like this before. Many of these apps replicate the tracking system sometimes installed on the phones of student athletes, for whom it is often mandatory. That system tells us a lot about what we can expect with these apps.
There is a widespread charade in the United States that university athletes, especially those who play high-profile sports such as football and basketball, are just students who happen to be playing sports as amateurs “in their free time.” The reality is that these college athletes in high-level sports, who are aggressively recruited by schools, bring prestige and financial resources to universities, under a regime that requires them to train like professional athletes despite their lack of salary. However, making the most of one’s college education and training at that level are virtually incompatible, simply because the day is 24 hours long and the body, even that of a young, healthy athlete, can only take so much when training so hard. Worse, many of these athletes are minority students, specifically Black men, who were underserved during their whole K–12 education and faced the same challenge then as they do now: Train hard in hopes of a scholarship and try to study with what little time is left, often despite being enrolled in schools with mediocre resources. Many of them arrive at college with an athletic scholarship but not enough academic preparation compared with their peers who went to better schools and could also concentrate on schooling….(More)”
How Competition Impacts Data Privacy
Paper by Aline Blankertz: “A small number of large digital platforms increasingly shape the space for most online interactions around the globe and they often act with hardly any constraint from competing services. The lack of competition puts those platforms in a powerful position that may allow them to exploit consumers and offer them limited choice. Privacy is increasingly considered one area in which the lack of competition may create harm. Because of these concerns, governments and other institutions are developing proposals to expand the scope for competition authorities to intervene to limit the power of the large platforms and to revive competition.
The first case that has explicitly addressed anticompetitive harm to privacy is the German Bundeskartellamt’s case against Facebook in which the authority argues that imposing bad privacy terms can amount to an abuse of dominance. Since that case started in 2016, more cases deal with the link between competition and privacy. For example, the proposed Google/Fitbit merger has raised concerns about sensitive health data being merged with existing Google profiles and Apple is under scrutiny for not sharing certain personal data while using it for its own services.
However, addressing bad privacy outcomes through competition policy is effective only if those outcomes are caused, at least partly, by a lack of competition. Six distinct mechanisms can be distinguished through which competition may affect privacy, as summarized in Table 1. These mechanisms constitute different hypotheses through which less competition may influence privacy outcomes and lead either to worse privacy in different ways (mechanisms 1-5) or even better privacy (mechanism 6). The table also summarizes the available evidence on whether and to what extent the hypothesized effects are present in actual markets….(More)”.
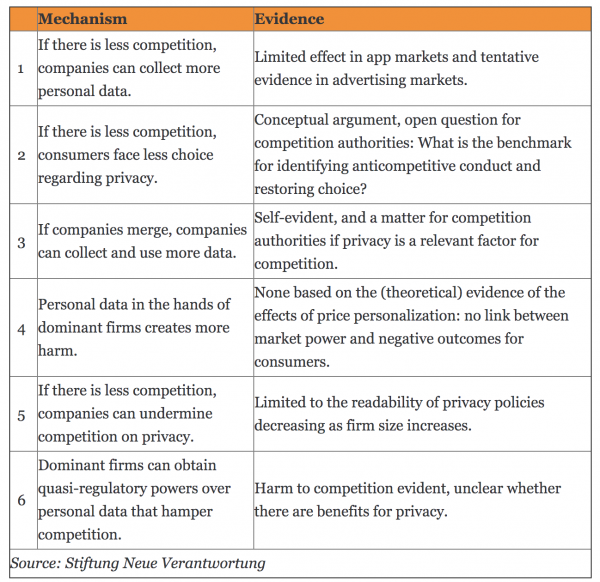