Book edited by Sarah B. Macfarlane and Carla AbouZahr: “This handbook compiles methods for gathering, organizing and disseminating data to inform policy and manage health systems worldwide. Contributing authors describe national and international structures for generating data and explain the relevance of ethics, policy, epidemiology, health economics, demography, statistics, geography and qualitative methods to describing population health. The reader, whether a student of global health, public health practitioner, programme manager, data analyst or policymaker, will appreciate the methods, context
Toward an Open Data Bias Assessment Tool Measuring Bias in Open Spatial Data
Working Paper by Ajjit Narayanan and Graham MacDonald: “Data is a critical resource for government decisionmaking, and in recent years, local governments, in a bid for transparency, community engagement, and innovation, have released many municipal datasets on publicly accessible open data portals. In recent years, advocates, reporters, and others have voiced concerns about the bias of algorithms used to guide public decisions and the data that power them.
Although significant progress is being made in developing tools for algorithmic bias and transparency, we could not find any standardized tools available for assessing bias in open data itself. In other words, how can policymakers, analysts, and advocates systematically measure the level of bias in the data that power city decisionmaking, whether an algorithm is used or not?
To fill this gap, we present a prototype of an automated bias assessment tool for geographic data. This new tool will allow city officials, concerned residents, and other stakeholders to quickly assess the bias and representativeness of their data. The tool allows users to upload a file with latitude and longitude coordinates and receive simple metrics of spatial and demographic bias across their city.
The tool is built on geographic and demographic data from the Census and assumes that the population distribution in a city represents the “ground truth” of the underlying distribution in the data uploaded. To provide an illustrative example of the tool’s use and output, we test our bias assessment on three datasets—bikeshare station locations, 311 service request locations, and Low Income Housing Tax Credit (LIHTC) building locations—across a few, hand-selected example cities….(More)”
Circular City Data
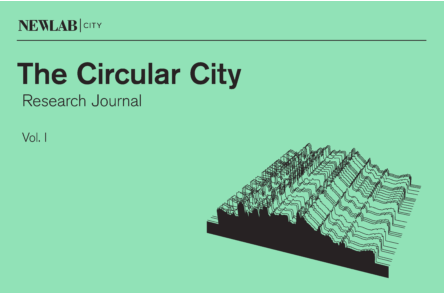
First Volume of Circular City, A Research Journal by New Lab edited by André Corrêa d’Almeida: “…Circular City Data is the topic being explored in the first iteration of New Lab’s The Circular City program, which looks at data and knowledge as the energy, flow, and medium of collaboration. Circular data refers to the collection, production, and exchange of data, and business insights, between a series of collaborators around a shared set of inquiries. In some scenarios, data may be produced by start-ups and of high value to the city; in other cases, data may be produced by the city and of potential value to the public, start-ups, or enterprise companies. The conditions that need to be in place to safely, ethically, and efficiently extrapolate the highest potential value from data are what this program aims to uncover.
Similar to living systems, urban systems can be enhanced if the total pool of data available, i.e., energy, can be democratized and decentralized and data analytics used widely to positively impact quality of life. The abundance of data available, the vast differences in capacity across organizations to handle it, and the growing complexity of urban challenges provides an opportunity to test how principles of circular city data can help establish new forms of public and private partnerships that make cities more economically prosperous, livable, and resilient. Though we talk of an overabundance of data, it is often still not visible or tactically wielded at the local level in a way that benefits people.
Circular City Data is an effort to build a safe environment whereby start-ups, city agencies, and larger firms can collect, produce, access and exchange data, as well as business insights, through transaction mechanisms that do not necessarily require currency, i.e., through reciprocity. Circular data is data that travels across a number of stakeholders, helping to deliver insights and make clearer the opportunities where such stakeholders can work together to improve outcomes. It includes cases where a set of “circular” relationships need to be in place in order to produce such data and business insights. For example, if an AI company lacks access to raw data from the city, they won’t be able to provide valuable insights to the city. Or, Numina required an established relationship with the DBP in order to access infrastructure necessary for them to install their product and begin generating data that could be shared back with them. ***
Next, the case study documents and explains how The Circular City program was conceived, designed, and implemented, with the goal of offering lessons for scalability at New Lab and replicability in other cities around the world. The three papers that follow investigate and methodologically test the value of circular data applied to three different, but related, urban challenges: economic growth, mobility, and resilience.
Contents
- Introduction to The Circular City Research Program (André Corrêa d’Almeida)
- The Circular City Program: The Case Study (André Corrêa d’Almeida and Caroline McHeffey)
- Circular Data for a Circular City: Value Propositions for Economic Development (Stefaan G. Verhulst, Andrew Young, and Andrew J. Zahuranec)
- Circular Data for a Circular City: Value Propositions for Mobility (Arnaud Sahuguet)
- Circular Data for a Circular City: Value Propositions for Resilience and Sustainability (Nilda Mesa)
Conclusio (André Corrêa d’Almeida)
Africa Data Revolution Report 2018
Report by Jean-Paul Van Belle et al: ” The Africa Data Revolution Report 2018 delves into the recent evolution and current state of open data – with an emphasis on Open Government Data – in the African data communities. It explores key countries across the continent, researches a wide range of open data initiatives, and benefits from global thematic expertise. This second edition improves on process, methodology and collaborative partnerships from the first edition.
It draws from country reports, existing global and continental initiatives, and key experts’ input, in order to provide a deep analysis of the
actual impact of open data in the African context. In particular, this report features a dedicated Open Data Barometer survey as well as a special 2018
Africa Open Data Index regional edition surveying the status and impact of open data and dataset availability in 30 African countries. The research is complemented with six in-depth qualitative case studies featuring the impact of open data in Kenya, South Africa (Cape Town), Ghana, Rwanda, Burkina Faso
Findings: In some governments, there is a slow iterative cycle between innovation, adoption, resistance
The role of open data intermediaries is crucial and has been insufficiently recognized in the African context. Open data in Africa needs a vibrant, dynamic, open and multi-tier data ecosystem if the datasets are to make a real impact. Citizens are rarely likely to access open data themselves. But the democratization of information and communication platforms has opened up opportunities among a large and diverse set of intermediaries to explore and combine relevant data sources, sometimes with private or leaked data. The news media, NGOs and advocacy groups, and to a much lesser extent academics and social or profit-driven entrepreneurs have shown that OGD can create
The report encourages national
data in Africa on the basis of this research. Other stakeholders working with or for open data can
Data Trusts: Ethics, Architecture and Governance for Trustworthy Data Stewardship
Web Science Institute Paper by Kieron O’Hara: “In their report on the development of the UK AI industry, Wendy Hall and Jérôme Pesenti
recommend the establishment of data trusts, “proven and trusted frameworks and agreements” that will “ensure exchanges [of data] are secure and mutually beneficial” by promoting trust in the use of data for AI. Hall and Pesenti leave the structure of data trusts open, and the purpose of this paper is to explore the questions of (a) what existing structures can data trusts exploit, and (b) what relationship do data trusts have to
trusts as they are understood in law?
The paper defends the following thesis: A data trust works within the law to provide ethical, architectural and governance support for trustworthy data processing
Data trusts are therefore both constraining and liberating. They constrain: they respect current law, so they cannot render currently illegal actions legal. They are intended to increase trust, and so they will typically act as
further constraints on data processors, adding the constraints of trustworthiness to those of law. Yet they also liberate: if data processors
are perceived as trustworthy, they will get improved access to data.
Most work on data trusts has up to now focused on gaining and supporting the trust of data subjects in data processing. However, all actors involved in AI – data consumers, data providers
Furthermore, it is not only personal data that creates trust issues; the same may be true of any dataset whose release might involve an
Big data needs big governance: best practices from Brain-CODE, the Ontario Brain Institute’s neuroinformatics platform
Shannon C. Lefaivre et al in Frontiers of Genetics: “The Ontario Brain Institute (OBI) has begun to catalyze scientific discovery in the field of neuroscience through its large-scale informatics platform, known as Brain-CODE. The platform supports the capture, storage, federation, sharing and analysis of different data types across several brain disorders. Underlying the platform is a robust and scalable data governance structure which allows for the flexibility to advance scientific understanding, while protecting the privacy of research participants.
Recognizing the value of an open science approach to enabling discovery, the governance structure was designed not only to support collaborative research programs, but also to support open science by making all data open and accessible in the future. OBI’s rigorous approach to data sharing maintains the accessibility of research data for big discoveries without compromising privacy and security. Taking a Privacy by Design approach to both data sharing and development of the platform has allowed OBI to establish some best practices related to large scale data sharing within Canada. The aim of this report is to highlight these best practices and develop a key open resource which may be referenced during the development of similar open science initiatives….(More)”.
Balancing information governance obligations when accessing social care data for collaborative research
Paper by Malkiat Thiarai, Sarunkorn Chotvijit
The paper documents the process involved in identifying, accessing and using data held in Birmingham City Council’s social care system for collaborative research with a partner
The findings will include approaches to understanding the data, its structure and accessibility tasks involved in addressing ethical and legal obligations and requirements of the ethical and governance processes….(More)”.
The new ecosystem of trust: How data trusts, collaboratives and coops can help govern data for the maximum public benefit
Paper by Geoff Mulgan and Vincent Straub: The world is struggling to govern data. The challenge is to reduce abuses of all kinds, enhance accountability and improve ethical standards, while also ensuring that the maximum public and private value can also be derived from data.
Despite many predictions to the contrary the world of commercial data is dominated by powerful organisations. By contrast, there are few institutions to protect the public interest and those that do exist remain relatively weak. This paper argues that new institutions—an ecosystem of trust—are needed to ensure that uses of data are trusted and trustworthy. It advocates the creation of different kinds of data trust to fill this gap. It argues:
- That we need, but currently lack, institutions that are good at thinking through, discussing, and explaining the often complex trade-offs that need to be made about data.
- That the task of creating trust is different in different fields. Overly generic solutions will be likely to fail.
- That trusts need to be accountable—in some cases to individual members where there is a direct relationship with individuals giving consent, in other cases to the broader public.
- That we should expect a variety of types of data trust to form—some sharing data; some managing synthetic data; some providing a research capability; some using commercial data and so on. The best analogy is finance which over time has developed a very wide range of types of institution and governance.
This paper builds on a series of Nesta think pieces on data and knowledge commons published over the last decade and current practical projects that explore how data can be mobilised to improve healthcare, policing, the jobs market and education. It aims to provide a framework for designing a new family of institutions under the umbrella title of data trusts, tailored to different conditions of consent, and different patterns of private and public value. It draws on the work of many others (including the work of GovLab and the Open Data Institute).
Introduction
The governance of personal data of all kinds has recently moved from being a very marginal specialist issue to one of general concern. Too much data has been misused, lost, shared, sold or combined with little involvement of the people most affected, and little ethical awareness on the part of the organisations in charge.
The most visible responses have been general ones—like the EU’s GDPR. But these now need to be complemented by new institutions that can be generically described as ‘data trusts’.
In current practice the term ‘trust’ is used to describe a very wide range of institutions. These include private trusts, a type of legal structure that holds and makes decisions about assets, such as property or investments, and involves trustors, trustees, and beneficiaries. There are also public trusts in fields like education with a duty to provide a public benefit. Examples include the Nesta Trust and the National Trust. There are trusts in business (e.g. to manage pension funds). And there are trusts in the public sector, such as the BBC Trust and NHS Foundation Trusts with remits to protect the public interest, at arms length from political decisions.
It’s now over a decade since the first data trusts were set up as private initiatives in response to anxieties about abuse. These were important pioneers though none achieved much scale or traction.
Now a great deal of work is underway around the world to consider what other types of trust might be relevant to data, so as to fill the governance vacuum—handling everything from transport data to personalised health, the internet of things to school records, and recognising the very different uses of data—by the state for taxation or criminal justice etc.; by academia for research; by business for use and resale; and to guide individual choices. This paper aims to feed into that debate.
1. The twin problems: trust and value
Two main clusters of problem are coming to prominence. The first cluster of problems involve misuseand overuse of data; the second set of problems involves underuse of data.
1.1. Lack of control fuels distrust
The first problem is a lack of control and agency—individuals feel unable to control data about their own lives (from Facebook links and Google searches to retail behaviour and health) and communities are unable to control their own public data (as in Sidewalk labs and other smart city projects that attempted to privatise public data). Lack of control leads to the risk of abuses of privacy, and a wider problem of decreasing trust—which survey evidence from the Open Data Institute (ODI) shows is key in determining the likelihood consumers will share their personal data (although this varies across countries). The lack of transparency regarding how personal data is then used to train algorithms making decisions only adds to the mistrust.
1.2 Lack of trust leads to a deficit of public value
The second, mirror cluster of problems concern value. Flows of data promise a lot: better ways to assess problems, understand options, and make decisions. But current arrangements make it hard for individuals to realise the greatest value from their own data, and they make it even harder for communities to safely and effectively aggregate, analyse and link data to solve pressing problems, from health and crime to mobility. This is despite the fact that many consumers are prepared to make trade-offs: to share data if it benefits themselves and others—a 2018 Nesta poll found, for example, that 73 per cent of people said they would share their personal data in an effort to improve public services if there was a simple and secure way of doing it. A key reason for the failure to maximise public value is the lack of institutions that are sufficiently trusted to make judgements in the public interest.
Attempts to answer these problems sometimes point in opposite directions—the one towards less free flow, less linking of data, the other towards more linking and combination. But any credible policy responses have to address both simultaneously.
2. The current landscape
The governance field was largely empty earlier this decade. It is now full of activity, albeit at an early stage. Some is legislative—like GDPR and equivalents being considered around the world. Some is about standards—like Verify, IHAN and other standards intended to handle secure identity. Some is more entrepreneurial—like the many Personal Data Stores launched over the last decade, from Mydexto SOLID, Citizen-me to digi.me. Some are experiments like the newly launched Amsterdam Data Exchange (Amdex) and the UK government’s recently announced efforts to fund data trust pilots to tackle wildlife conservation, working with the ODI. Finally, we are now beginning to see new institutions within government to guide and shape activity, notably the new Centre for Data Ethics and Innovation.
Many organisations have done pioneering work, including the ODI in the UK and NYU GovLab with its work on data collaboratives. At Nesta, as part of the Europe-wide DECODE consortium, we are helping to develop new tools to give people control of their personal data while the Next Generation Internet (NGI) initiative is focused on creating a more inclusive, human-centric and resilient internet—with transparency and privacy as two of the guiding pillars.
The task of governing data better brings together many elements, from law and regulation to ethics and standards. We are just beginning to see more serious discussion about tax and data—from the proposals to tax digital platforms turnover to more targeted taxes of data harvesting in public places or infrastructures—and more serious debate around regulation. This paper deals with just one part of this broader picture: the role of institutions dedicated to curating data in the public interest
Tomorrow’s Data Heroes
Article by Florian Gröne, Pierre Péladeau, and Rawia Abdel Samad: “Telecom companies are struggling to find a profitable identity in today’s digital sphere. What about helping customers control their information?…
By 2025, Alex had had enough. There no longer seemed to be any distinction between her analog and digital lives. Everywhere she went, every purchase she completed, and just about every move she made, from exercising at the gym to idly surfing the Web, triggered a vast flow of data. That in turn meant she was bombarded with personalized advertising messages, targeted more and more eerily to her. As she walked down the street, messages appeared on her phone about the stores she was passing. Ads popped up on her all-purpose tablet–computer–phone pushing drugs for minor health problems she didn’t know she had — until the symptoms appeared the next day. Worse, she had recently learned that she was being reassigned at work. An AI machine had mastered her current job by analyzing her use of the firm’s productivity software.
It was as if the algorithms of global companies knew more about her than she knew herself — and they probably did. How was it that her every action and conversation, even her thoughts, added to the store of data held about her? After all, it was her data: her preferences, dislikes, interests, friendships, consumer choices, activities, and whereabouts — her very identity — that was being collected, analyzed, profited from, and even used to manage her. All these companies seemed to be making money buying and selling this information. Why shouldn’t she gain some control over the data she generated, and maybe earn some cash by selling it to the companies that had long collected it free of charge?
So Alex signed up for the “personal data manager,” a new service that promised to give her control over her privacy and identity. It was offered by her U.S.-based connectivity company (in this article, we’ll call it DigiLife, but it could be one of many former telephone companies providing Internet services in 2025). During the previous few years, DigiLife had transformed itself into a connectivity hub: a platform that made it easier for customers to join, manage, and track interactions with media and software entities across the online world. Thanks to recently passed laws regarding digital identity and data management, including the “right to be forgotten,” the DigiLife data manager was more than window dressing. It laid out easy-to-follow choices that all Web-based service providers were required by law to honor
Today, in 2019, personal data management applications like the one Alex used exist only in nascent form, and consumers have yet to demonstrate that they trust these services. Nor can they yet profit by selling their data. But the need is great, and so is the opportunity for companies that fulfill it. By 2025, the total value of the data economy as currently structured will rise to more than US$400 billion, and by monetizing the vast amounts of data they produce, consumers can potentially recapture as much as a quarter of that total.
Given the critical role of telecom operating companies within the digital economy — the central position of their data networks, their networking capabilities, their customer relationships, and their experience in government affairs — they are in a good position to seize this business opportunity. They might not do it alone; they are likely to form consortia with software companies or other digital partners. Nonetheless, for legacy connectivity companies, providing this type of service may be the most sustainable business option. It may also be the best option for the rest of us, as we try to maintain control in a digital world flooded with our personal data….(More)”.
Open-Data: A Solution When Data Constitutes an Essential Facility?
Chapter by Claire Borsenberger, Mathilde Hoang and Denis Joram: “Thanks to appropriate data algorithms, firms, especially those