New report from the National Academy of Sciences: “Data mining of massive data sets is transforming the way we think about crisis response, marketing, entertainment, cybersecurity and national intelligence. Collections of documents, images, videos, and networks are being thought of not merely as bit strings to be stored, indexed, and retrieved, but as potential sources of discovery and knowledge, requiring sophisticated analysis techniques that go far beyond classical indexing and keyword counting, aiming to find relational and semantic interpretations of the phenomena underlying the data.
Frontiers in Massive Data Analysis examines the frontier of analyzing massive amounts of data, whether in a static database or streaming through a system. Data at that scale–terabytes and petabytes–is increasingly common in science (e.g., particle physics, remote sensing, genomics), Internet commerce, business analytics, national security, communications, and elsewhere. The tools that work to infer knowledge from data at smaller scales do not necessarily work, or work well, at such massive scale. New tools, skills, and approaches are necessary, and this report identifies many of them, plus promising research directions to explore. Frontiers in Massive Data Analysis discusses pitfalls in trying to infer knowledge from massive data, and it characterizes seven major classes of computation that are common in the analysis of massive data. Overall, this report illustrates the cross-disciplinary knowledge–from computer science, statistics, machine learning, and application disciplines–that must be brought to bear to make useful inferences from massive data.”
New! Humanitarian Computing Library
Patrick Meier at iRevolution: “The field of “Humanitarian Computing” applies Human Computing and Machine Computing to address major information-based challengers in the humanitarian space. Human Computing refers to crowdsourcing and microtasking, which is also referred to as crowd computing. In contrast, Machine Computing draws on natural language processing and machine learning, amongst other disciplines. The Next Generation Humanitarian Technologies we are prototyping at QCRI are powered by Humanitarian Computing research and development (R&D).
My QCRI colleagues and I just launched the first ever Humanitarian Computing Library which is publicly available here. The purpose of this library, or wiki, is to consolidate existing and future research that relate to Humanitarian Computing in order to support the development of next generation humanitarian tech. The repository currently holds over 500 publications that span topics such as Crisis Management, Trust and Security, Software and Tools, Geographical Analysis and Crowdsourcing. These publications are largely drawn from (but not limited to) peer-reviewed papers submitted at leading conferences around the world. We invite you to add your own research on humanitarian computing to this growing collection of resources.”
Radical Abundance: How a Revolution in Nanotechnology Will Change Civilization
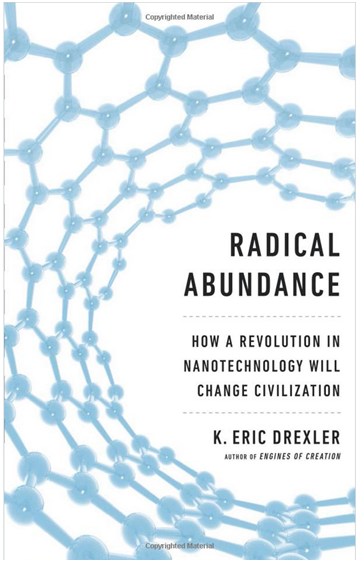
This paper established fundamental principles of molecular engineering and outlined development paths to advanced nanotechnologies.
He popularized the idea of nanotechnology in his 1986 book, Engines of Creation: The Coming Era of Nanotechnology, where he introduced a broad audience to a fundamental technology objective: using machines that work at the molecular scale to structure matter from the bottom up.
He went on to continue his PhD thesis at MIT, under the guidance of AI-pioneer Marvin Minsky, and published it in a modified form as a book in 1992 as Nanosystems: Molecular Machinery, Manufacturing, and Computation.
Drexler’s new book, Radical Abundance: How a Revolution in Nanotechnology Will Change Civilization, tells the story of nanotechnology from its small beginnings, then moves quickly towards a big future, explaining what it is and what it is not, and enlightening about what we can do with it for the benefit of humanity.
In his pioneering 1986 book, Engines of Creation, he defined nanotechnology as a potential technology with these features: “manufacturing using machinery based on nanoscale devices, and products built with atomic precision.”
In his 2013 sequel, Radical Abundance, Drexler expands on his prior thinking, corrects many of the misconceptions about nanotechnology, and dismisses fears of dystopian futures replete with malevolent nanobots and gray goo…
His new book clearly identifies nanotechnology with atomically precise manufacturing (APM)…Drexler makes many comparisons between the information revolution and what he now calls the “APM revolution.” What the first did with bits, the second will do with atoms: “Image files today will be joined by product files tomorrow. Today one can produce an image of the Mona Lisa without being able to draw a good circle; tomorrow one will be able to produce a display screen without knowing how to manufacture a wire.”
Civilization, he says, is advancing from a world of scarcity toward a world of abundance — indeed, radical abundance.”
On our best behaviour
Paper by Hector J. Levesque: “The science of AI is concerned with the study of intelligent forms of behaviour in computational terms. But what does it tell us when a good semblance of a behaviour can be achieved using cheap tricks that seem to have little to do with what we intuitively imagine intelligence to be? Are these intuitions wrong, and is intelligence really just a bag of tricks? Or are the philosophers right, and is a behavioural understanding of intelligence simply too weak? I think both of these are wrong. I suggest in the context of question-answering that what matters when it comes to the science of AI is not a good semblance of intelligent behaviour at all, but the behaviour itself, what it depends on, and how it can be achieved. I go on to discuss two major hurdles that I believe will need to be cleared.”
Big data, crowdsourcing and machine learning tackle Parkinson’s
Successful Workingplace: “Parkinson’s is a very tough disease to fight. People suffering from the disease often have significant tremors that keep them from being able to create accurate records of their daily challenges. Without this information, doctors are unable to fine tune drug dosages and other treatment regimens that can significantly improve the lives of sufferers.
It was a perfect catch-22 situation until recently, when the Michael J. Fox Foundation announced that LIONsolver, a company specializing in machine learning software, was able to differentiate Parkinson’s patients from healthy individuals and to also show the trend in symptoms of the disease over time.…
To set up the competition, the Foundation worked with Kaggle, an organization that specializes in crowdsourced big data analysis competitions. The use of crowdsourcing as a way to get to the heart of very difficult Big Data problems works by allowing people the world over from a myriad of backgrounds and with diverse experiences to devote time on personally chosen challenges where they can bring the most value. It’s a genius idea for bringing some of the scarcest resources together with the most intractable problems.”
Data Science for Social Good
Data Science for Social Good: “By analyzing data from police reports to website clicks to sensor signals, governments are starting to spot problems in real-time and design programs to maximize impact. More nonprofits are measuring whether or not they’re helping people, and experimenting to find interventions that work.
None of this is inevitable, however.
We’re just realizing the potential of using data for social impact and face several hurdles to it’s widespread adoption:
- Most governments and nonprofits simply don’t know what’s possible yet. They have data – but often not enough and maybe not the right kind.
- There are too few data scientists out there – and too many spending their days optimizing ads instead of bettering lives.
To make an impact, we need to show social good organizations the power of data and analytics. We need to work on analytics projects that have high social impact. And we need to expose data scientists to the problems that really matter.
The fellowship
That’s exactly why we’re doing the Eric and Wendy Schmidt Data Science for Social Good summer fellowship at the University of Chicago.
We want to bring three dozen aspiring data scientists to Chicago, and have them work on data science projects with social impact.
Working closely with governments and nonprofits, fellows will take on real-world problems in education, health, energy, transportation, and more.
Over the next three months, they’ll apply their coding, machine learning, and quantitative skills, collaborate in a fast-paced atmosphere, and learn from mentors in industry, academia, and the Obama campaign.
The program is led by a strong interdisciplinary team from the Computation institute and the Harris School of Public Policy at the University of Chicago.”
City Data: Big, Open and Linked
Working Paper by Mark S. Fox (University of Toronto): “Cities are moving towards policymaking based on data. They are publishing data using Open Data standards, linking data from disparate sources, allowing the crowd to update their data with Smart Phone Apps that use Open APIs, and applying “Big Data” Techniques to discover relationships that lead to greater efficiencies.
One Big City Data example is from New York City (Schönberger & Cukier, 2013). Building owners were illegally converting their buildings into rooming houses that contained 10 times the number people they were designed for. These buildings posed a number of problems, including fire hazards, drugs, crime, disease and pest infestations. There are over 900,000 properties in New York City and only 200 inspectors who received over 25,000 illegal conversion complaints per year. The challenge was to distinguish nuisance complaints from those worth investigating where current methods were resulting in only 13% of the inspections resulting in vacate orders.
New York’s Analytics team created a dataset that combined data from 19 agencies including buildings, preservation, police, fire, tax, and building permits. By combining data analysis with expertise gleaned from inspectors (e.g., buildings that recently received a building permit were less likely to be a problem as they were being well maintained), the team was able to develop a rating system for complaints. Based on their analysis of this data, they were able to rate complaints such that in 70% of their visits, inspectors issued vacate orders; a fivefold increase in efficiency…
This paper provides an introduction to the concepts that underlie Big City Data. It explains the concepts of Open, Unified, Linked and Grounded data that lie at the heart of the Semantic Web. It then builds on this by discussing Data Analytics, which includes Statistics, Pattern Recognition and Machine Learning. Finally we discuss Big Data as the extension of Data Analytics to the Cloud where massive amounts of computing power and storage are available for processing large data sets. We use city data to illustrate each.”
Analyzing the Analyzers

We used dimensionality reduction techniques to divide potential data scientists into five categories based on their self-ranked skill sets (Statistics, Math/Operations Research, Business, Programming, and Machine Learning/Big Data), and four categories based on their self-identification (Data Researchers, Data Businesspeople, Data Engineers, and Data Creatives). Further examining the respondents based on their division into these categories provided additional insights into the types of professional activities, educational background, and even scale of data used by different types of Data Scientists.
In this report, we combine our results with insights and data from others to provide a better understanding of the diversity of practitioners, and to argue for the value of clearer communication around roles, teams, and careers.”
OGP Report: "Opening Government"
Open Gov Blog: “In 2011, the Transparency and Accountability Initiative (T/AI) published “Opening Government” – a guide for civil society organisations, and governments, to support them to develop and update ambitious and targeted action plans for the Open Government Partnership.
This year, T/AI is working with a number of expert organisations and participants in the Open Government Partnership to update and expand the guide into a richer online resource, which will include new topic areas and more lessons and updates from ongoing experience….
Below you’ll find an early draft of the section in GoogleDocs, where we invite you to edit and comment on it and help to develop it further. In particular, we’d value your thoughts on the following:
-
Are the headline illustrative commitments realistic and stretching at each of the levels? If not, please suggest how they should be changed.
-
Are there any significant gaps in the illustrative commitments? Please suggest any additional commitments you feel should be included – and better yet, write it!
-
Are the recommendations clear and useful? Please suggest any alterations you feel should be made.
-
Are there particular country experiences that should be expanded on? Please suggest any good examples you are aware of (preferably linking to a write-up of the project).
-
Are there any particularly useful resources missing? If so, please point us towards them.
This draft – which is very much a work in progress – is open for comments and edits, so please contribute as you wish. You can also send any thoughts to me via: [email protected]”
Policy Modeling through Collaboration and Simulation
New paper on “Bridging narrative scenario texts and formal policy modeling through conceptual policy modeling” in Artificial Intelligence and Law.
Abstract: “Engaging stakeholders in policy making and supporting policy development with advanced information and communication technologies including policy simulation is currently high on the agenda of research. In order to involve stakeholders in providing their input to policy modeling via online means, simple techniques need to be employed such as scenario technique. Scenarios enable stakeholders to express their views in narrative text. At the other end of policy development, a frequently used approach to policy modeling is agent-based simulation. So far, effective support to transform narrative text input to formal simulation statements is not widely available. In this paper, we present a novel approach to support the transformation of narrative texts via conceptual modeling into formal simulation models. The approach also stores provenance information which is conveyed via annotations of texts to the conceptual model and further on to the simulation model. This way, traceability of information is provided, which contributes to better understanding and transparency, and therewith enables stakeholders and policy modelers to return to the sources that informed the conceptual and simulation model.”