Paper by Harini Suresh and John Guttag: “As machine learning (ML) increasingly affects people and society, awareness of its potential unwanted consequences has also grown. To anticipate, prevent, and mitigate undesirable downstream consequences, it is critical that we understand when and how harm might be introduced throughout the ML life cycle. In this case study, we provide a framework that identifies seven distinct potential sources of downstream harm in machine learning, spanning data collection, development, and deployment. We describe how these issues arise, how they are relevant to particular applications, and how they motivate different mitigations. …(More)
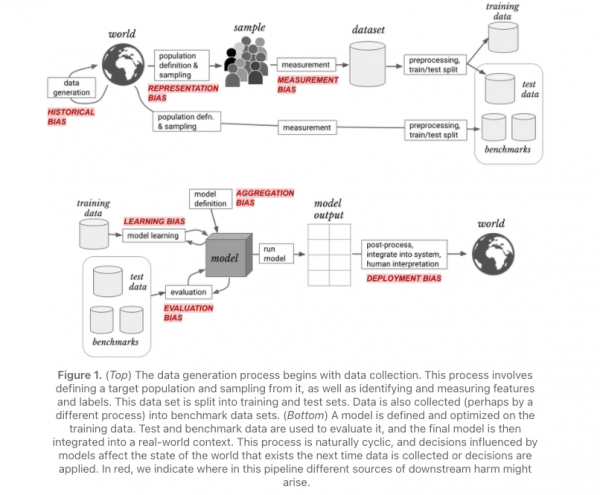