Paper by Baobao Zhang, Markus Anderljung, Lauren Kahn, Naomi Dreksler, Michael C. Horowitz, and Allan Dafoe: “Machine learning (ML) and artificial intelligence (AI) researchers play an important role in the ethics and governance of AI, including through their work, advocacy, and choice of employment. Nevertheless, this influential group’s attitudes are not well understood, undermining our ability to discern consensuses or disagreements between AI/ML researchers. To examine these researchers’ views, we conducted a survey of those who published in two top AI/ML conferences (N = 524). We compare these results with those from a 2016 survey of AI/ML researchers (Grace et al., 2018) and a 2018 survey of the US public (Zhang & Dafoe, 2020). We find that AI/ML researchers place high levels of trust in international organizations and scientific organizations to shape the development and use of AI in the public interest; moderate trust in most Western tech companies; and low trust in national militaries, Chinese tech companies, and Facebook….(More)”.
The Open-Source Movement Comes to Medical Datasets
Blog by Edmund L. Andrews: “In a move to democratize research on artificial intelligence and medicine, Stanford’s Center for Artificial Intelligence in Medicine and Imaging (AIMI) is dramatically expanding what is already the world’s largest free repository of AI-ready annotated medical imaging datasets.
Artificial intelligence has become an increasingly pervasive tool for interpreting medical images, from detecting tumors in mammograms and brain scans to analyzing ultrasound videos of a person’s pumping heart.
Many AI-powered devices now rival the accuracy of human doctors. Beyond simply spotting a likely tumor or bone fracture, some systems predict the course of a patient’s illness and make recommendations.
But AI tools have to be trained on expensive datasets of images that have been meticulously annotated by human experts. Because those datasets can cost millions of dollars to acquire or create, much of the research is being funded by big corporations that don’t necessarily share their data with the public.
“What drives this technology, whether you’re a surgeon or an obstetrician, is data,” says Matthew Lungren, co-director of AIMI and an assistant professor of radiology at Stanford. “We want to double down on the idea that medical data is a public good, and that it should be open to the talents of researchers anywhere in the world.”
Launched two years ago, AIMI has already acquired annotated datasets for more than 1 million images, many of them from the Stanford University Medical Center. Researchers can download those datasets at no cost and use them to train AI models that recommend certain kinds of action.
Now, AIMI has teamed up with Microsoft’s AI for Health program to launch a new platform that will be more automated, accessible, and visible. It will be capable of hosting and organizing scores of additional images from institutions around the world. Part of the idea is to create an open and global repository. The platform will also provide a hub for sharing research, making it easier to refine different models and identify differences between population groups. The platform can even offer cloud-based computing power so researchers don’t have to worry about building local resource intensive clinical machine-learning infrastructure….(More)”.
AI, big data, and the future of consent
Paper by Adam J. Andreotta, Nin Kirkham & Marco Rizzi: “In this paper, we discuss several problems with current Big data practices which, we claim, seriously erode the role of informed consent as it pertains to the use of personal information. To illustrate these problems, we consider how the notion of informed consent has been understood and operationalised in the ethical regulation of biomedical research (and medical practices, more broadly) and compare this with current Big data practices. We do so by first discussing three types of problems that can impede informed consent with respect to Big data use. First, we discuss the transparency (or explanation) problem. Second, we discuss the re-repurposed data problem. Third, we discuss the meaningful alternatives problem. In the final section of the paper, we suggest some solutions to these problems. In particular, we propose that the use of personal data for commercial and administrative objectives could be subject to a ‘soft governance’ ethical regulation, akin to the way that all projects involving human participants (e.g., social science projects, human medical data and tissue use) are regulated in Australia through the Human Research Ethics Committees (HRECs). We also consider alternatives to the standard consent forms, and privacy policies, that could make use of some of the latest research focussed on the usability of pictorial legal contracts…(More)”
The social dilemma in AI development and why we have to solve it
Paper by Inga Strümke, Marija Slavkovik and Vince I. Madai: “While the demand for ethical artificial intelligence (AI) systems increases, the number of unethical uses of AI accelerates, even though there is no shortage of ethical guidelines. We argue that a main underlying cause for this is that AI developers face a social dilemma in AI development ethics, preventing the widespread adaptation of ethical best practices. We define the social dilemma for AI development and describe why the current crisis in AI development ethics cannot be solved without relieving AI developers of their social dilemma. We argue that AI development must be professionalised to overcome the social dilemma, and discuss how medicine can be used as a template in this process….(More)”.
The Secret Bias Hidden in Mortgage-Approval Algorithms
An investigation by The Markup: “…has found that lenders in 2019 were more likely to deny home loans to people of color than to White people with similar financial characteristics—even when we controlled for newly available financial factors that the mortgage industry for years has said would explain racial disparities in lending.
Holding 17 different factors steady in a complex statistical analysis of more than two million conventional mortgage applications for home purchases, we found that lenders were 40 percent more likely to turn down Latino applicants for loans, 50 percent more likely to deny Asian/Pacific Islander applicants, and 70 percent more likely to deny Native American applicants than similar White applicants. Lenders were 80 percent more likely to reject Black applicants than similar White applicants. These are national rates.
In every case, the prospective borrowers of color looked almost exactly the same on paper as the White applicants, except for their race.
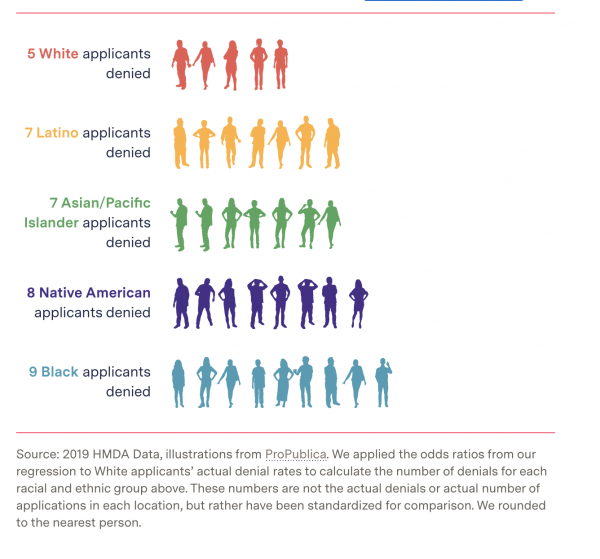
The industry had criticized previous similar analyses for not including financial factors they said would explain disparities in lending rates but were not public at the time: debts as a percentage of income, how much of the property’s assessed worth the person is asking to borrow, and the applicant’s credit score.
The first two are now public in the Home Mortgage Disclosure Act data. Including these financial data points in our analysis not only failed to eliminate racial disparities in loan denials, it highlighted new, devastating ones.
We found that lenders gave fewer loans to Black applicants than White applicants even when their incomes were high—$100,000 a year or more—and had the same debt ratios. In fact, high-earning Black applicants with less debt were rejected more often than high-earning White applicants who have more debt….(More)”
Understanding Potential Sources of Harm throughout the Machine Learning Life Cycle
Paper by Harini Suresh and John Guttag: “As machine learning (ML) increasingly affects people and society, awareness of its potential unwanted consequences has also grown. To anticipate, prevent, and mitigate undesirable downstream consequences, it is critical that we understand when and how harm might be introduced throughout the ML life cycle. In this case study, we provide a framework that identifies seven distinct potential sources of downstream harm in machine learning, spanning data collection, development, and deployment. We describe how these issues arise, how they are relevant to particular applications, and how they motivate different mitigations. …(More)
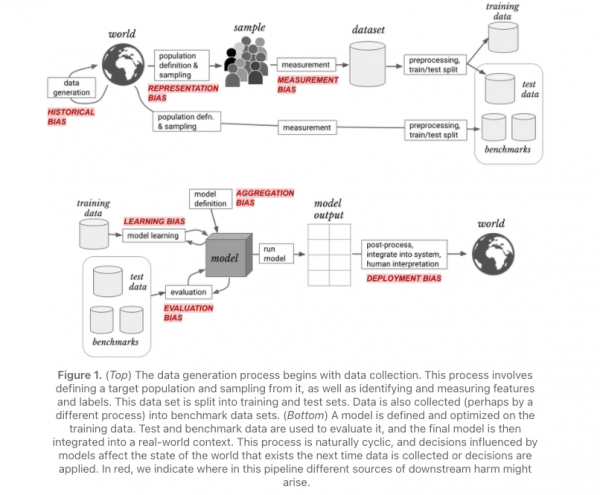
Looking Under the Hood of AI’s Dubious Models
Essay by Ethan Edwards: “In 2018, McKinsey Global Institute released “Notes from the AI Frontier,” a report that seeks to predict the economic impact of artificial intelligence. Looming over the report is how the changing nature of work might transform society and pose challenges for policymakers. The good news is that the experts at McKinsey think that automation will create more jobs than it eliminates, but obviously it’s not a simple question. And the answer they give rests on sophisticated econometric models that include a variety of qualifications and estimates. Such models are necessarily simplified, and even reductionistic, but are they useful? And for whom?
Without a doubt, when it comes to predictive modeling, the center of the action in our society—and the industry through which intense entrepreneurial energy and venture capital flows—is artificial intelligence itself. AI, of course, is nothing new. A subdiscipline dedicated to mimicking human capacities in sensing, language, and thought, it’s nearly as old as computer science itself. But for the last ten years or so the promise and the hype of AI have only accelerated. The most impressive results have come from something called “neural nets,” which has used linear algebra to mimic some of the biological structures of our brain cells and has been combined with far better hardware developed for video games. In only a few years, neural nets have revolutionized image processing, language processing, audio analysis, and media recommendation. The hype is that they can do far more.
If we are—as many promoters assert—close to AIs that can do everything a human knowledge worker can and more, that is obviously a disruptive, even revolutionary, prospect. It’s also a claim that has turned on the spigot of investment capital. And that’s one reason it’s difficult to know the true potential of the industry. Talking about AI is a winning formula for startups, researchers, and anyone who wants funding, enough that the term AI gets used for more than just neural nets and is now a label for computer-based automation in general. Older methods that have nothing to do with the new boom have been rebranded under AI. Think tanks and universities are hosting seminars on the impact of AI on fields on which it has so far had no impact. Some startups who have built their company’s future profitability on the promise of their AI systems have actually had to hire low-wage humans to act like the hoped-for intelligences for customers and investors while they wait for the technology to catch up. Such hype produces a funhouse mirror effect that distorts the potential and therefore the value of firms and all but guarantees that some startups will squander valuable resources with broken (or empty) promises. But as long as some companies do keep their promises it’s gamble that many investors are still willing to take….(More)”.
Co-design and Ethical Artificial Intelligence for Health: Myths and Misconceptions
Paper by Joseph Donia and Jay Shaw: “Applications of artificial intelligence / machine learning (AI/ML) are dynamic and rapidly growing, and although multi-purpose, are particularly consequential in health care. One strategy for anticipating and addressing ethical challenges related to AI/ML for health care is co-design – or involvement of end users in design. Co-design has a diverse intellectual and practical history, however, and has been conceptualized in many different ways. Moreover, the unique features of AI/ML introduce challenges to co-design that are often underappreciated. This review summarizes the research literature on involvement in health care and design, and informed by critical data studies, examines the extent to which co-design as commonly conceptualized is capable of addressing the range of normative issues raised by AI/ML for health. We suggest that AI/ML technologies have amplified existing challenges related to co-design, and created entirely new challenges. We outline five co-design ‘myths and misconceptions’ related to AI/ML for health that form the basis for future research and practice. We conclude by suggesting that the normative strength of a co-design approach to AI/ML for health can be considered at three levels: technological, health care system, and societal. We also suggest research directions for a ‘new era’ of co-design capable of addressing these challenges….(More)”.
Philanthropy Can Help Communities Weed Out Inequity in Automated Decision Making Tools
Article by Chris Kingsley and Stephen Plank: “Two very different stories illustrate the impact of sophisticated decision-making tools on individuals and communities. In one, the Los Angeles Police Department publicly abandoned a program that used data to target violent offenders after residents in some neighborhoods were stopped by police as many as 30 times per week. In the other, New York City deployed data to root out landlords who discriminated against tenants using housing vouchers.
The second story shows the potential of automated data tools to promote social good — even as the first illustrates their potential for great harm.
Tools like these — typically described broadly as artificial intelligence or somewhat more narrowly as predictive analytics, which incorporates more human decision making in the data collection process — increasingly influence and automate decisions that affect people’s lives. This includes which families are investigated by child protective services, where police deploy, whether loan officers extend credit, and which job applications a hiring manager receives.
How these tools are built, used, and governed will help shape the opportunities of everyday citizens, for good or ill.
Civil-rights advocates are right to worry about the harm such technology can do by hardpwiring bias into decision making. At the Annie E. Casey Foundation, where we fund and support data-focused efforts, we consulted with civil-rights groups, data scientists, government leaders, and family advocates to learn more about what needs to be done to weed out bias and inequities in automated decision-making tools — and recently produced a report about how to harness their potential to promote equity and social good.
Foundations and nonprofit organizations can play vital roles in ensuring equitable use of A.I. and other data technology. Here are four areas in which philanthropy can make a difference:
Support the development and use of transparent data tools. The public has a right to know how A.I. is being used to influence policy decisions, including whether those tools were independently validated and who is responsible for addressing concerns about how they work. Grant makers should avoid supporting private algorithms whose design and performance are shielded by trade-secrecy claims. Despite calls from advocates, some companies have declined to disclose details that would allow the public to assess their fairness….(More)”
The Society of Algorithms
Paper by Jenna Burrell and Marion Fourcade: “The pairing of massive data sets with processes—or algorithms—written in computer code to sort through, organize, extract, or mine them has made inroads in almost every major social institution. This article proposes a reading of the scholarly literature concerned with the social implications of this transformation. First, we discuss the rise of a new occupational class, which we call the coding elite. This group has consolidated power through their technical control over the digital means of production and by extracting labor from a newly marginalized or unpaid workforce, the cybertariat. Second, we show that the implementation of techniques of mathematical optimization across domains as varied as education, medicine, credit and finance, and criminal justice has intensified the dominance of actuarial logics of decision-making, potentially transforming pathways to social reproduction and mobility but also generating a pushback by those so governed. Third, we explore how the same pervasive algorithmic intermediation in digital communication is transforming the way people interact, associate, and think. We conclude by cautioning against the wildest promises of artificial intelligence but acknowledging the increasingly tight coupling between algorithmic processes, social structures, and subjectivities….(More)”.