Paper by Vincent J. Straub, Deborah Morgan, Jonathan Bright, Helen Margetts: “Recent advances in artificial intelligence (AI) and machine learning (ML) hold the promise of improving government. Given the advanced capabilities of AI applications, it is critical that these are embedded using standard operational procedures, clear epistemic criteria, and behave in alignment with the normative expectations of society. Scholars in multiple domains have subsequently begun to conceptualize the different forms that AI systems may take, highlighting both their potential benefits and pitfalls. However, the literature remains fragmented, with researchers in social science disciplines like public administration and political science, and the fast-moving fields of AI, ML, and robotics, all developing concepts in relative isolation. Although there are calls to formalize the emerging study of AI in government, a balanced account that captures the full breadth of theoretical perspectives needed to understand the consequences of embedding AI into a public sector context is lacking. Here, we unify efforts across social and technical disciplines by using concept mapping to identify 107 different terms used in the multidisciplinary study of AI. We inductively sort these into three distinct semantic groups, which we label the (a) operational, (b) epistemic, and (c) normative domains. We then build on the results of this mapping exercise by proposing three new multifaceted concepts to study AI-based systems for government (AI-GOV) in an integrated, forward-looking way, which we call (1) operational fitness, (2) epistemic completeness, and (3) normative salience. Finally, we put these concepts to work by using them as dimensions in a conceptual typology of AI-GOV and connecting each with emerging AI technical measurement standards to encourage operationalization, foster cross-disciplinary dialogue, and stimulate debate among those aiming to reshape public administration with AI…(More)”.
Sex and Gender Bias in Technology and Artificial Intelligence
Book edited by Davide Cirillo, Silvina Catuara Solarz, and Emre Guney: “…details the integration of sex and gender as critical factors in innovative technologies (artificial intelligence, digital medicine, natural language processing, robotics) for biomedicine and healthcare applications. By systematically reviewing existing scientific literature, a multidisciplinary group of international experts analyze diverse aspects of the complex relationship between sex and gender, health and technology, providing a perspective overview of the pressing need of an ethically-informed science. The reader is guided through the latest implementations and insights in technological areas of accelerated growth, putting forward the neglected and overlooked aspects of sex and gender in biomedical research and healthcare solutions that leverage artificial intelligence, biosensors, and personalized medicine approaches to predict and prevent disease outcomes. The reader comes away with a critical understanding of this fundamental issue for the sake of better future technologies and more effective clinical approaches….(More)”.
Could an algorithm predict the next pandemic?
Article by Simon Makin: “Leap is a machine-learning algorithm that uses sequence data to classify influenza viruses as either avian or human. The model had been trained on a huge number of influenza genomes — including examples of H5N8 — to learn the differences between those that infect people and those that infect birds. But the model had never seen an H5N8 virus categorized as human, and Carlson was curious to see what it made of this new subtype.
Somewhat surprisingly, the model identified it as human with 99.7% confidence. Rather than simply reiterating patterns in its training data, such as the fact that H5N8 viruses do not typically infect people, the model seemed to have inferred some biological signature of compatibility with humans. “It’s stunning that the model worked,” says Carlson. “But it’s one data point; it would be more stunning if I could do it a thousand more times.”
The zoonotic process of viruses jumping from wildlife to people causes most pandemics. As climate change and human encroachment on animal habitats increase the frequency of these events, understanding zoonoses is crucial to efforts to prevent pandemics, or at least to be better prepared.
Researchers estimate that around 1% of the mammalian viruses on the planet have been identified1, so some scientists have attempted to expand our knowledge of this global virome by sampling wildlife. This is a huge task, but over the past decade or so, a new discipline has emerged — one in which researchers use statistical models and machine learning to predict aspects of disease emergence, such as global hotspots, likely animal hosts or the ability of a particular virus to infect humans. Advocates of such ‘zoonotic risk prediction’ technology argue that it will allow us to better target surveillance to the right areas and situations, and guide the development of vaccines and therapeutics that are most likely to be needed.
However, some researchers are sceptical of the ability of predictive technology to cope with the scale and ever-changing nature of the virome. Efforts to improve the models and the data they rely on are under way, but these tools will need to be a part of a broader effort if they are to mitigate future pandemics…(More)”.
The Proof is in the Pudding: Revealing the SDGs with Artificial Intelligence
AFD Research Paper: “The use of frontier technologies in the field of sustainability is likely to accompany its visibility, and the quality of information available to decision makers. This paper explores the possibility of using artificial intelligence to analyze Public Development Banks’ annual reports…(More)”.
Responsible AI licenses: a practical tool for implementing the OECD Principles for Trustworthy AI
Article by Carlos Muñoz Ferrandis: “Recent socio-ethical concerns on the development, use, and commercialization of AI-related products and services have led to the emergence of new types of licenses devoted to promoting the responsible use of AI systems: Responsible AI Licenses, or RAILs.
RAILs are AI-specific licenses that include restrictions on how the licensee can use the AI feature due to the licensor’s concerns about the technical capabilities and limitations of the AI feature. This approach concerns the two existing types of these licenses. The RAIL license can be used for ML models, source code, applications and services, and data. When these licenses allow free access and flexible downstream distribution of the licensed AI feature, they are OpenRAIL
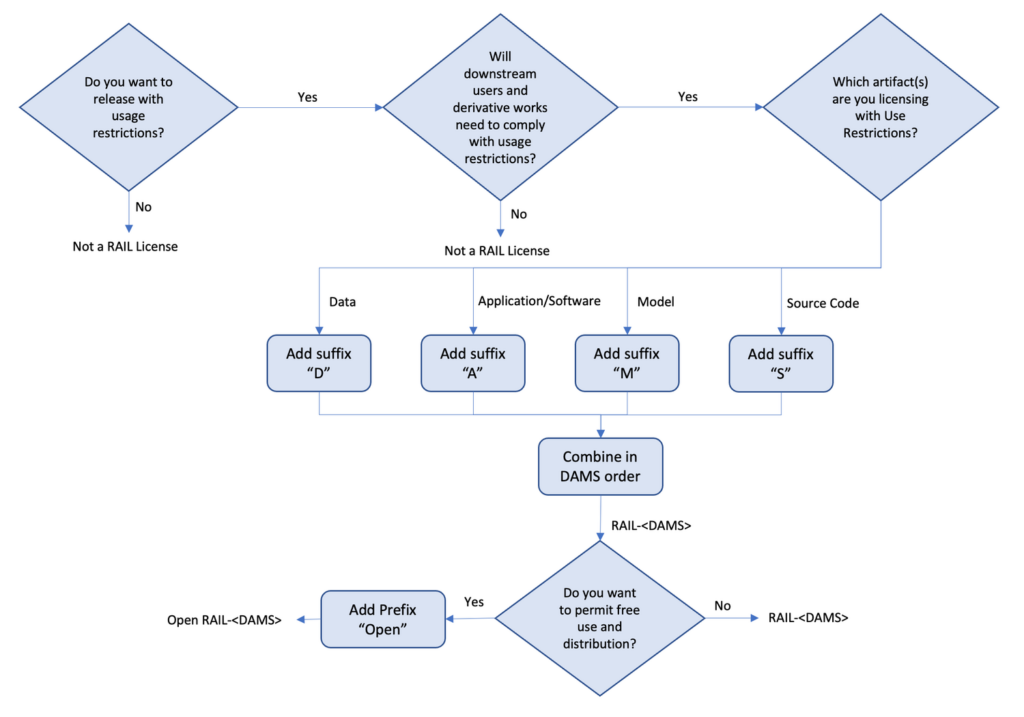
The RAIL Initiative was created in 2019 to encourage the industry to adopt use restrictions in licenses as a way to mitigate the risks of misuse and potential harm caused by AI systems…(More)”.
How Confucianism could put fear about Artificial Intelligence to bed
Article by Tom Cassauwers: “Western culture has had a long history of individualism, warlike use of technology, Christian apocalyptic thinking and a strong binary between body and soul. These elements might explain the West’s obsession with the technological apocalypse and its opposite: techno-utopianism. In Asia, it’s now common to explain China’s dramatic rise as a leader in AI and robotics as a consequence of state support from the world’s largest economy. But what if — in addition to the massive state investment — China and other Asian nations have another advantage, in the form of Eastern philosophies?
There’s a growing view among independent researchers and philosophers that Confucianism and Buddhism could offer healthy alternative perspectives on the future of technology. And with AI and robots rapidly increasing in importance across industries, it’s time for the West to turn to the East for answers…
So what would a non-Western way of thinking about tech look like? First, there might be a different interpretation of personhood. Both Confucianism and Buddhism potentially open up the way for nonhumans to reach the status of humans. In Confucianism, the state of reaching personhood “is not a given. You need to work to achieve it,” says Wong. The person’s attitude toward certain ethical virtues determines whether or not they reach the status of a human. That also means that “we can attribute personhood to nonhuman things like robots when they play ethically relevant roles and duties as humans,” Wong adds.
Buddhism offers a similar argument, where robots can hypothetically achieve a state of enlightenment, which is present everywhere, not only in humans — an argument made as early as the 1970s by Japanese roboticist Masahiro Mori. It may not be a coincidence that robots enjoy some of their highest social acceptance in Japan, with its Buddhist heritage. “Westerners are generally reluctant about the nature of robotics and AI, considering only humans as true beings, while Easterners more often consider devices as similar to humans,” says Jordi Vallverdú, a professor of philosophy at the Autonomous University of Barcelona….(More)”
The Exploited Labor Behind Artificial Intelligence
Essay by Adrienne Williams, Milagros Miceli, and Timnit Gebru: “The public’s understanding of artificial intelligence (AI) is largely shaped by pop culture — by blockbuster movies like “The Terminator” and their doomsday scenarios of machines going rogue and destroying humanity. This kind of AI narrative is also what grabs the attention of news outlets: a Google engineer claiming that its chatbot was sentient was among the most discussed AI-related news in recent months, even reaching Stephen Colbert’s millions of viewers. But the idea of superintelligent machines with their own agency and decision-making power is not only far from reality — it distracts us from the real risks to human lives surrounding the development and deployment of AI systems. While the public is distracted by the specter of nonexistent sentient machines, an army of precarized workers stands behind the supposed accomplishments of artificial intelligence systems today.
Many of these systems are developed by multinational corporations located in Silicon Valley, which have been consolidating power at a scale that, journalist Gideon Lewis-Kraus notes, is likely unprecedented in human history. They are striving to create autonomous systems that can one day perform all of the tasks that people can do and more, without the required salaries, benefits or other costs associated with employing humans. While this corporate executives’ utopia is far from reality, the march to attempt its realization has created a global underclass, performing what anthropologist Mary L. Gray and computational social scientist Siddharth Suri call ghost work: the downplayed human labor driving “AI”.
Tech companies that have branded themselves “AI first” depend on heavily surveilled gig workers like data labelers, delivery drivers and content moderators. Startups are even hiring people to impersonate AI systems like chatbots, due to the pressure by venture capitalists to incorporate so-called AI into their products. In fact, London-based venture capital firm MMC Ventures surveyed 2,830 AI startups in the EU and found that 40% of them didn’t use AI in a meaningful way…(More)”.
Does AI Debias Recruitment? Race, Gender, and AI’s “Eradication of Difference”
Paper by Eleanor Drage & Kerry Mackereth: “In this paper, we analyze two key claims offered by recruitment AI companies in relation to the development and deployment of AI-powered HR tools: (1) recruitment AI can objectively assess candidates by removing gender and race from their systems, and (2) this removal of gender and race will make recruitment fairer, help customers attain their DEI goals, and lay the foundations for a truly meritocratic culture to thrive within an organization. We argue that these claims are misleading for four reasons: First, attempts to “strip” gender and race from AI systems often misunderstand what gender and race are, casting them as isolatable attributes rather than broader systems of power. Second, the attempted outsourcing of “diversity work” to AI-powered hiring tools may unintentionally entrench cultures of inequality and discrimination by failing to address the systemic problems within organizations. Third, AI hiring tools’ supposedly neutral assessment of candidates’ traits belie the power relationship between the observer and the observed. Specifically, the racialized history of character analysis and its associated processes of classification and categorization play into longer histories of taxonomical sorting and reflect the current demands and desires of the job market, even when not explicitly conducted along the lines of gender and race. Fourth, recruitment AI tools help produce the “ideal candidate” that they supposedly identify through by constructing associations between words and people’s bodies. From these four conclusions outlined above, we offer three key recommendations to AI HR firms, their customers, and policy makers going forward…(More)”.
Four ways that AI and robotics are helping to transform other research fields
Article by Michael Eisenstein: “Artificial intelligence (AI) is already proving a revolutionary tool for bioinformatics; the AlphaFold database set up by London-based company DeepMind, owned by Google, is allowing scientists to predict the structures of 200 million proteins across 1 million species. But other fields are benefiting too. Here, we describe the work of researchers pursuing cutting-edge AI and robotics techniques to better anticipate the planet’s changing climate, uncover the hidden history behind artworks, understand deep sea ecology and develop new materials.
Marine biology with a soft touch
It takes a tough organism to withstand the rigours of deep-sea living. But these resilient species are also often remarkably delicate, ranging from soft and squishy creatures such as jellyfish and sea cucumbers, to firm but fragile deep-sea fishes and corals. Their fragility makes studying these organisms a complex task.
The rugged metal manipulators found on many undersea robots are more likely to harm such specimens than to retrieve them intact. But ‘soft robots’ based on flexible polymers are giving marine biologists such as David Gruber, of the City University of New York, a gentler alternative for interacting with these enigmatic denizens of the deep…(More)”.
Can Smartphones Help Predict Suicide?
Ellen Barry in The New York Times: “In March, Katelin Cruz left her latest psychiatric hospitalization with a familiar mix of feelings. She was, on the one hand, relieved to leave the ward, where aides took away her shoelaces and sometimes followed her into the shower to ensure that she would not harm herself.
But her life on the outside was as unsettled as ever, she said in an interview, with a stack of unpaid bills and no permanent home. It was easy to slide back into suicidal thoughts. For fragile patients, the weeks after discharge from a psychiatric facility are a notoriously difficult period, with a suicide rate around 15 times the national rate, according to one study.
This time, however, Ms. Cruz, 29, left the hospital as part of a vast research project which attempts to use advances in artificial intelligence to do something that has eluded psychiatrists for centuries: to predict who is likely to attempt suicide and when that person is likely to attempt it, and then, to intervene.
On her wrist, she wore a Fitbit programmed to track her sleep and physical activity. On her smartphone, an app was collecting data about her moods, her movement and her social interactions. Each device was providing a continuous stream of information to a team of researchers on the 12th floor of the William James Building, which houses Harvard’s psychology department.
In the field of mental health, few new areas generate as much excitement as machine learning, which uses computer algorithms to better predict human behavior. There is, at the same time, exploding interest in biosensors that can track a person’s mood in real time, factoring in music choices, social media posts, facial expression and vocal expression.
Matthew K. Nock, a Harvard psychologist who is one of the nation’s top suicide researchers, hopes to knit these technologies together into a kind of early-warning system that could be used when an at-risk patient is released from the hospital…(More)”.